Learning from Learned Network: An Introspective Model for Arthroscopic Scene Segmentation
Studies in Autonomic, Data-driven and Industrial Computing(2023)
摘要
The knee joint, due to its complex, confined, and dynamic nature, poses several challenges in visualizing anatomical details for diagnostic and minimally invasive surgical procedures. Furthermore, presence of texture- and feature-less tissues introduce image artifacts, and large intra-class dissimilarity makes automatic image segmentation a complex problem to solve. Segmentation models cannot handle situations when image frame distribution differs from the training data set (out-of-distribution). In this study, we implement a secondary ‘learning network’ with the ability to learn as the primary segmentation network fails in handling segment with inconsistent data distributions. The combined architecture significantly increases the overall accuracy and fidelity of the segmentation process. We have achieved this by evaluating internal feature maps and confidence maps of the segmentation model in a separate training within the fully convolutional network. The proposed two-stage segmentation network flags failures in segmentation and re-labels them as it can learn both in- and out- data distributions. This provides state-of-the-art segmentation accuracy across different tissue types with dice similarity 0.9112, 0.963, 0.862, for ACL, femur, tibia, respectively.
更多查看译文
关键词
arthroscopic scene segmentation,learned network,learning
AI 理解论文
溯源树
样例
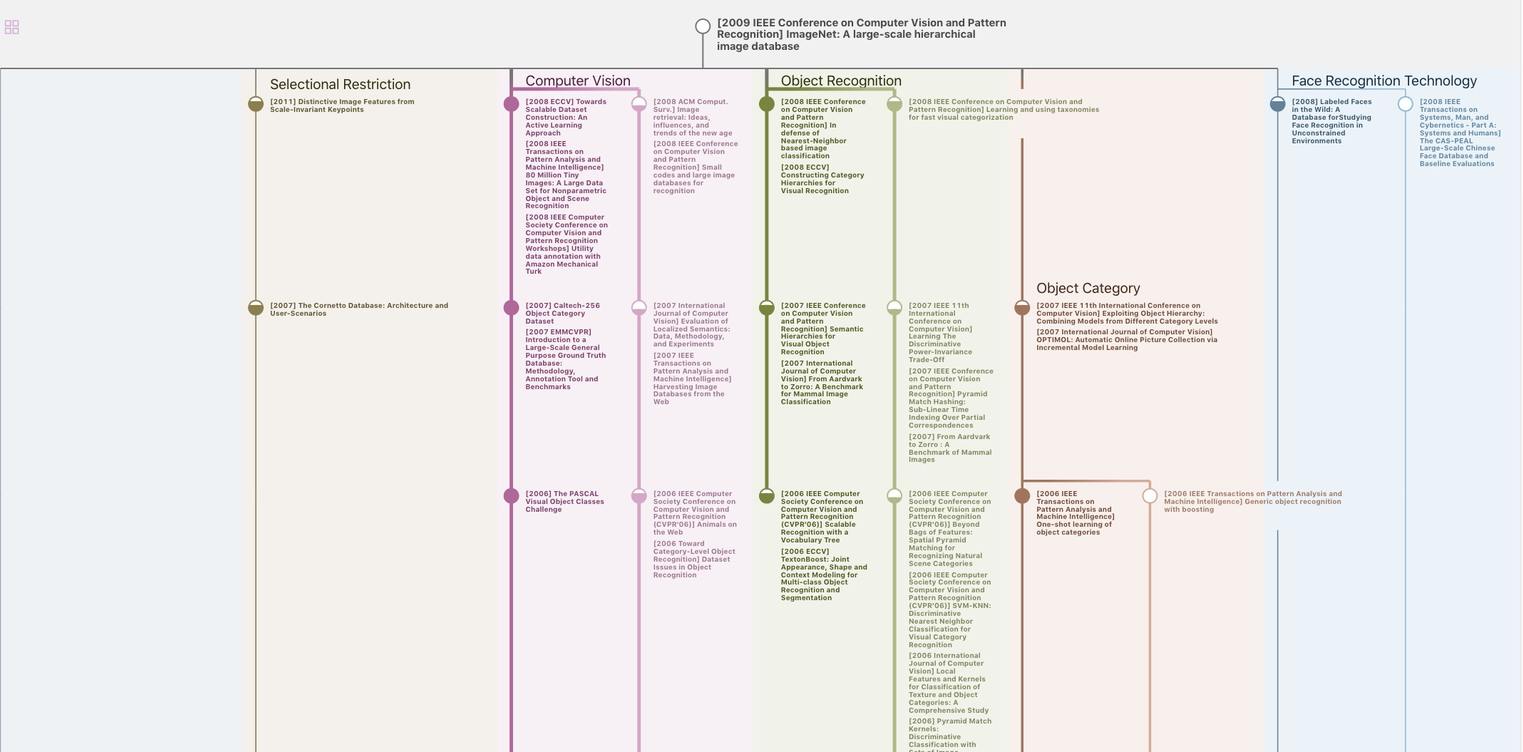
生成溯源树,研究论文发展脉络
Chat Paper
正在生成论文摘要