A Mixture Learning Strategy for Predicting Aquifers Permeability Coefficient K
Social Science Research Network(2023)
摘要
The collection of aquifer permeability coefficient k is very expensive in hydro-geophysical engineering projects. Many companies spend a lot of money without obtaining the recommended value of parameter k which creates a huge loss of investment. The use of machine learning for k-parameter prediction seems an alternative way to reduce the cost of data collection thereby saving money. However, the borehole data comes with a lot of missing since the parameter is strongly tied to the aquifer after the pumping test. In other words, the k-parameter collection is feasible if the layer in the well is an aquifer. Unfortunately, predicting some samples of k in a large set of missing data remains an issue using the classical supervised learning methods. We, therefore propose an alternative approach called a mixture learning strategy (MXS) to solve these double issues. It entails predicting upstream a naïve group of aquifers (NGA) combined with the real values k to counterbalance the missing values and yield an optimal prediction score. The method, first, implies the K-Means and Hierarchical Agglomerative Clustering (HAC) algorithms, and second, the Support Vector (SVMs) and Extreme Gradient Boosting (XGB) machines. The former (K-Means and HAC) is used for NGA label predicting whereas the latter is used for the final prediction. As a result, the SVMs and the XGB performed >70% of correct predictions on the validation set. Moreover, the best performances are obtained via the radial basis function kernel (83%) and XGB (87%). The final prediction carried out with a test borehole demonstrated the efficiency of the MXS approach. Henceforth, MXS could be used to reduce unsuccessful pumping tests that occur during drilling operations thereby saving money.
更多查看译文
关键词
mixture learning strategy,permeability
AI 理解论文
溯源树
样例
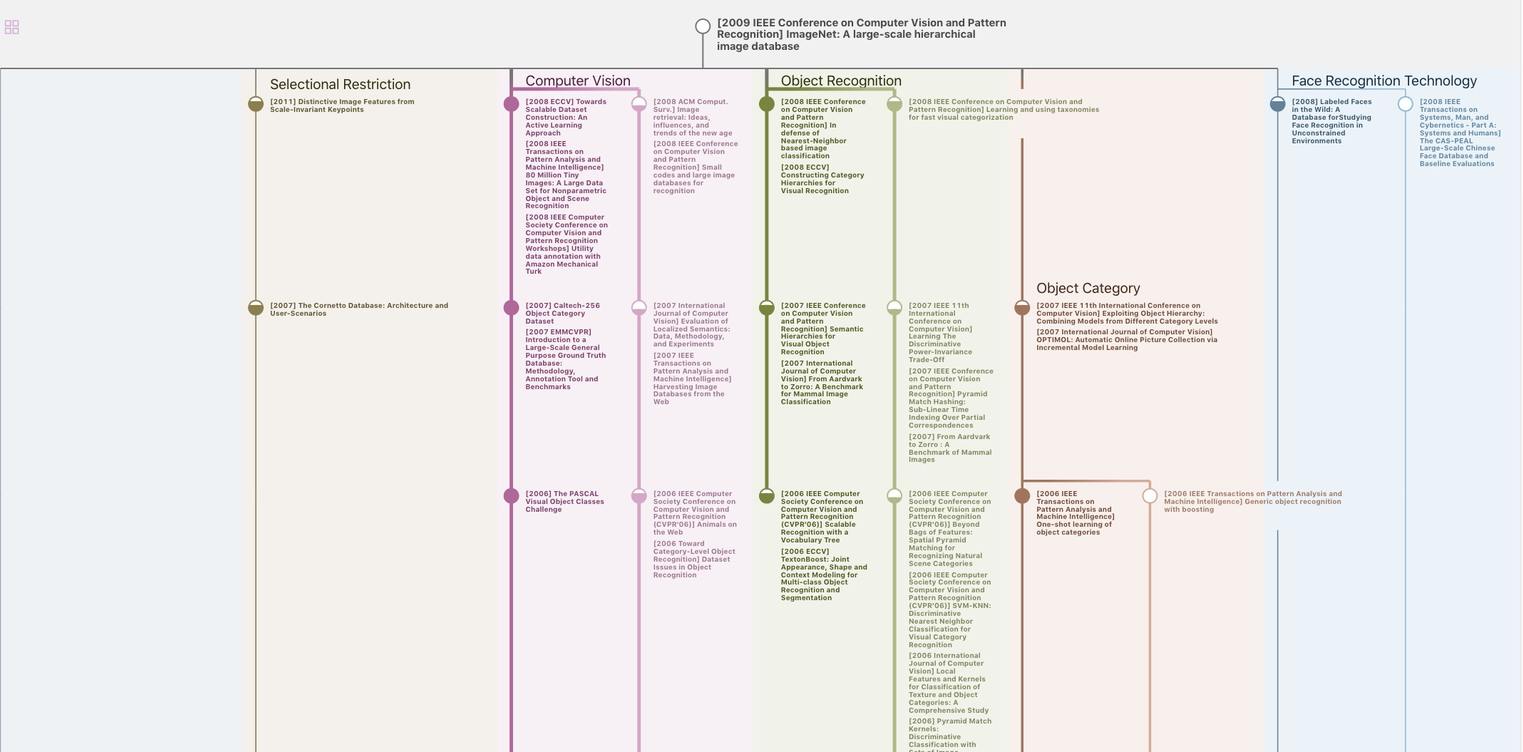
生成溯源树,研究论文发展脉络
Chat Paper
正在生成论文摘要