Solving PDEs with Unmeasurable Source Terms Using Coupled Physics-Informed Neural Network with Recurrent Prediction for Soft Sensors
arXiv (Cornell University)(2023)
摘要
Partial differential equations (PDEs) are a model candidate for soft sensors in industrial processes with spatiotemporal dependence. Although physics-informed neural networks (PINNs) are a promising machine learning method for solving PDEs, they are infeasible for the nonhomogeneous PDEs with unmeasurable source terms. To this end, a coupled PINN (CPINN) with a recurrent prediction (RP) learning strategy (CPINN- RP) is proposed. First, CPINN composed of NetU and NetG is proposed. NetU is for approximating PDEs solutions and NetG is for regularizing the training of NetU. The two networks are integrated into a data-physics-hybrid loss function. Then, we theoretically prove that the proposed CPINN has a satisfying approximation capability for solutions to nonhomogeneous PDEs with unmeasurable source terms. Besides the theoretical aspects, we propose a hierarchical training strategy to optimize and couple NetU and NetG. Secondly, NetU-RP is proposed for compensating information loss in data sampling to improve the prediction performance, in which RP is the recurrently delayed outputs of well-trained CPINN and hard sensors. Finally, the artificial and practical datasets are used to verify the feasibility and effectiveness of CPINN-RP for soft sensors.
更多查看译文
关键词
pdes,recurrent prediction,soft sensors,unmeasurable source terms,physics-informed
AI 理解论文
溯源树
样例
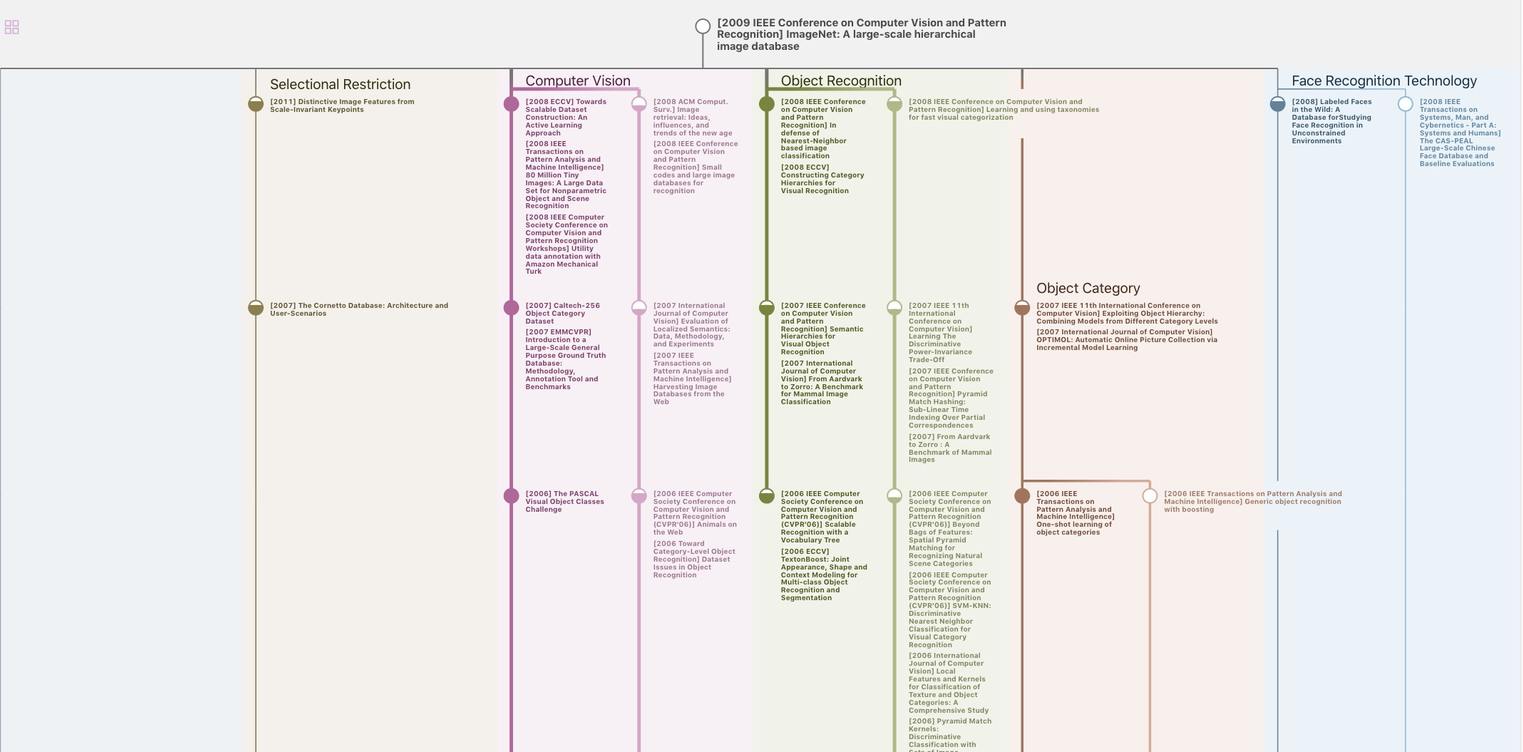
生成溯源树,研究论文发展脉络
Chat Paper
正在生成论文摘要