MooseNet: A Trainable Metric for Synthesized Speech with a PLDA Module
arXiv (Cornell University)(2023)
摘要
We present MooseNet, a trainable speech metric that predicts the listeners' Mean Opinion Score (MOS). We propose a novel approach where the Probabilistic Linear Discriminative Analysis (PLDA) generative model is used on top of an embedding obtained from a self-supervised learning (SSL) neural network (NN) model. We show that PLDA works well with a non-finetuned SSL model when trained only on 136 utterances (ca. one minute training time) and that PLDA consistently improves various neural MOS prediction models, even state-of-the-art models with task-specific fine-tuning. Our ablation study shows PLDA training superiority over SSL model fine-tuning in a low-resource scenario. We also improve SSL model fine-tuning using a convenient optimizer choice and additional contrastive and multi-task training objectives. The fine-tuned MooseNet NN with the PLDA module achieves the best results, surpassing the SSL baseline on the VoiceMOS Challenge data.
更多查看译文
关键词
synthesized speech,plda module,trainable metric
AI 理解论文
溯源树
样例
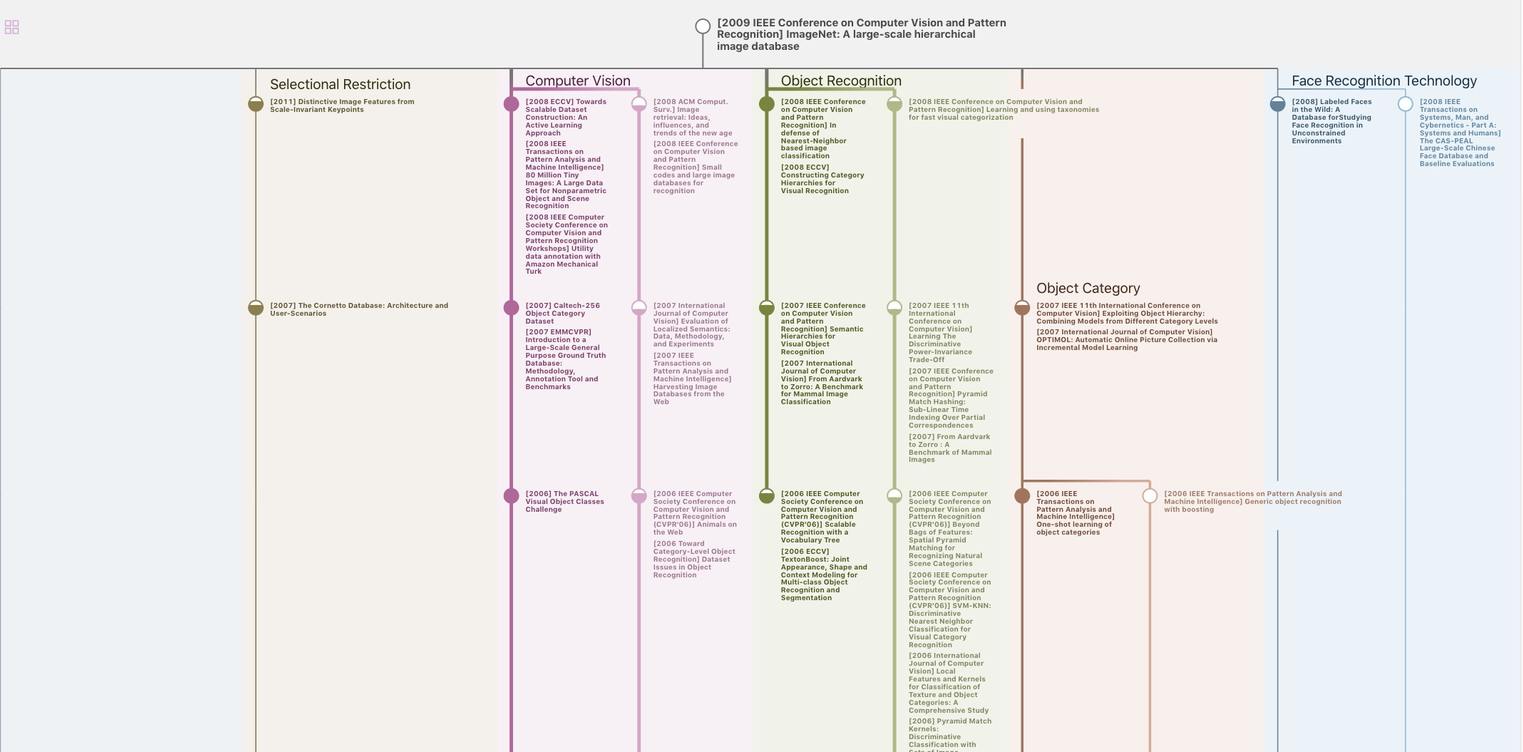
生成溯源树,研究论文发展脉络
Chat Paper
正在生成论文摘要