DSGRAE: Deep Sparse Graph Regularized Autoencoder for Anomaly Detection
Lecture Notes in Computer Science(2023)
摘要
Anomaly detection aims to distinguish significant deviation data from an observed dataset, which has wide applications in various fields. Autoencoder (AE) is an effective approach, which maps the original data into latent feature space, and then identifies the anomalies with higher reconstruction errors. However, the performance of autoencoder-based approach heavily relies on feature representations in the latent space, which requires the feature representations be captured as much essential as possible. Therefore, a graph regularization constraint term is first introduced into Deep Autoencoder (DAE) to explore the geometric structure information. Moreover, to avoid the problem of overfitting and enhance the ability of feature representations, a constraint term is imposed and then a Deep Sparse Graph Regularized Autoencoder (DSGRAE) approach is proposed. Finally, we carry out extensive experiments on 14 widely used datasets and compare them with other state-of-the-art methods, which demonstrate the effectiveness of the proposed method.
更多查看译文
关键词
anomaly
AI 理解论文
溯源树
样例
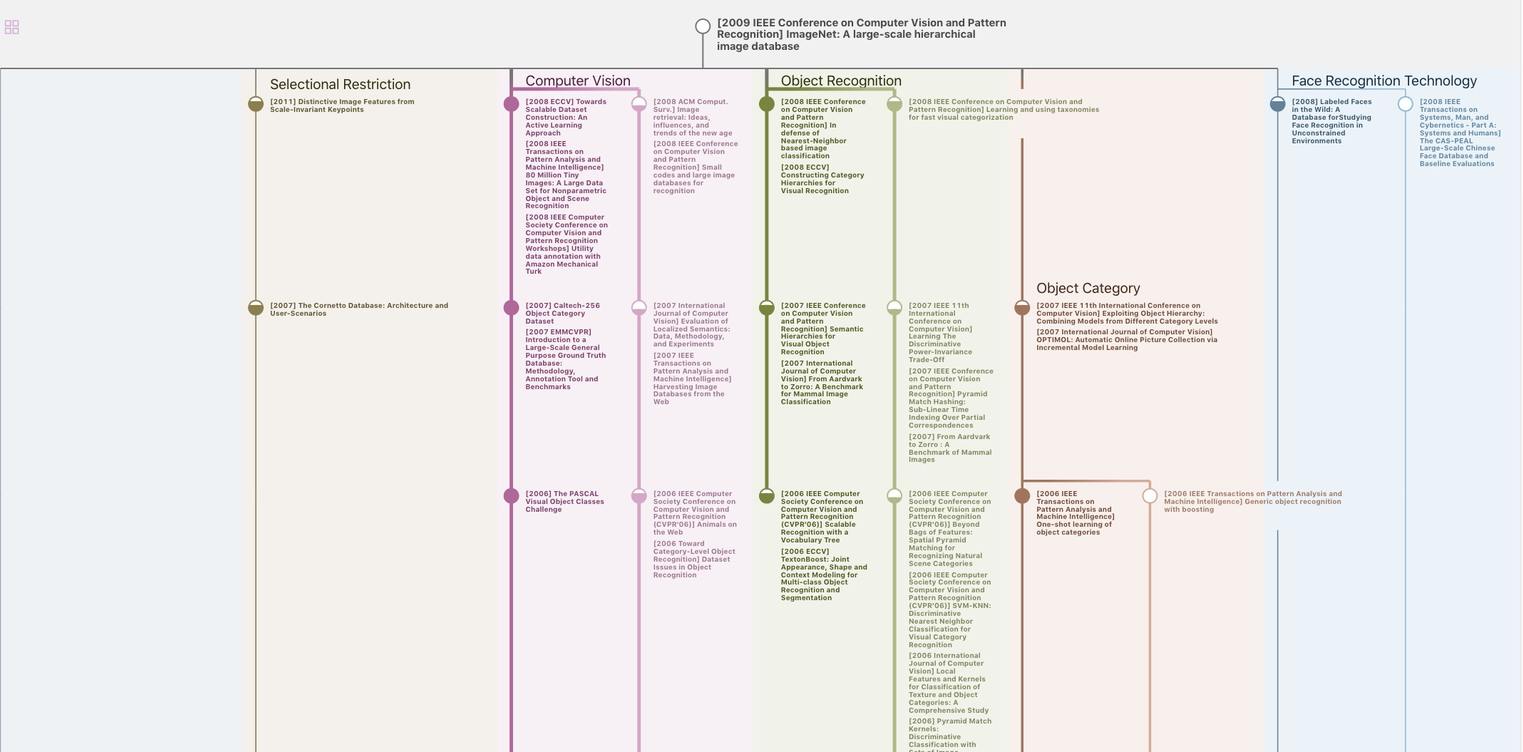
生成溯源树,研究论文发展脉络
Chat Paper
正在生成论文摘要