Data-driven design of eutectic high entropy alloys
Journal of materials informatics(2023)
摘要
Eutectic high entropy alloys (EHEAs) have attracted tremendous research interest over the past decade due to their superior physical and mechanical properties. Given the compositional complexity, there are no well-established phase diagrams for EHEAs. Therefore, the compositional design of EHEAs has been following a trial-and-error empirical approach, which is time-consuming, costly, and ineffective. To accelerate the search for EHEAs, data-driven approaches, particularly machine learning (ML) based modeling, have recently been utilized in lieu of the traditional empirical approach. In this article, we provide a critical overview of the recent efforts in the design and development of EHEAs, which covers the various empirical methods and the state-of-the-art machine learning models developed for EHEAs. In addition, we also briefly discuss the mechanical properties and plasticity strengthening mechanisms in EHEAs which are related to their heterogeneous microstructure, such as heterogeneous deformation induced strengthening, twinning induced strengthening, and phase transformation induced strengthening.
更多查看译文
关键词
alloys,entropy,data-driven
AI 理解论文
溯源树
样例
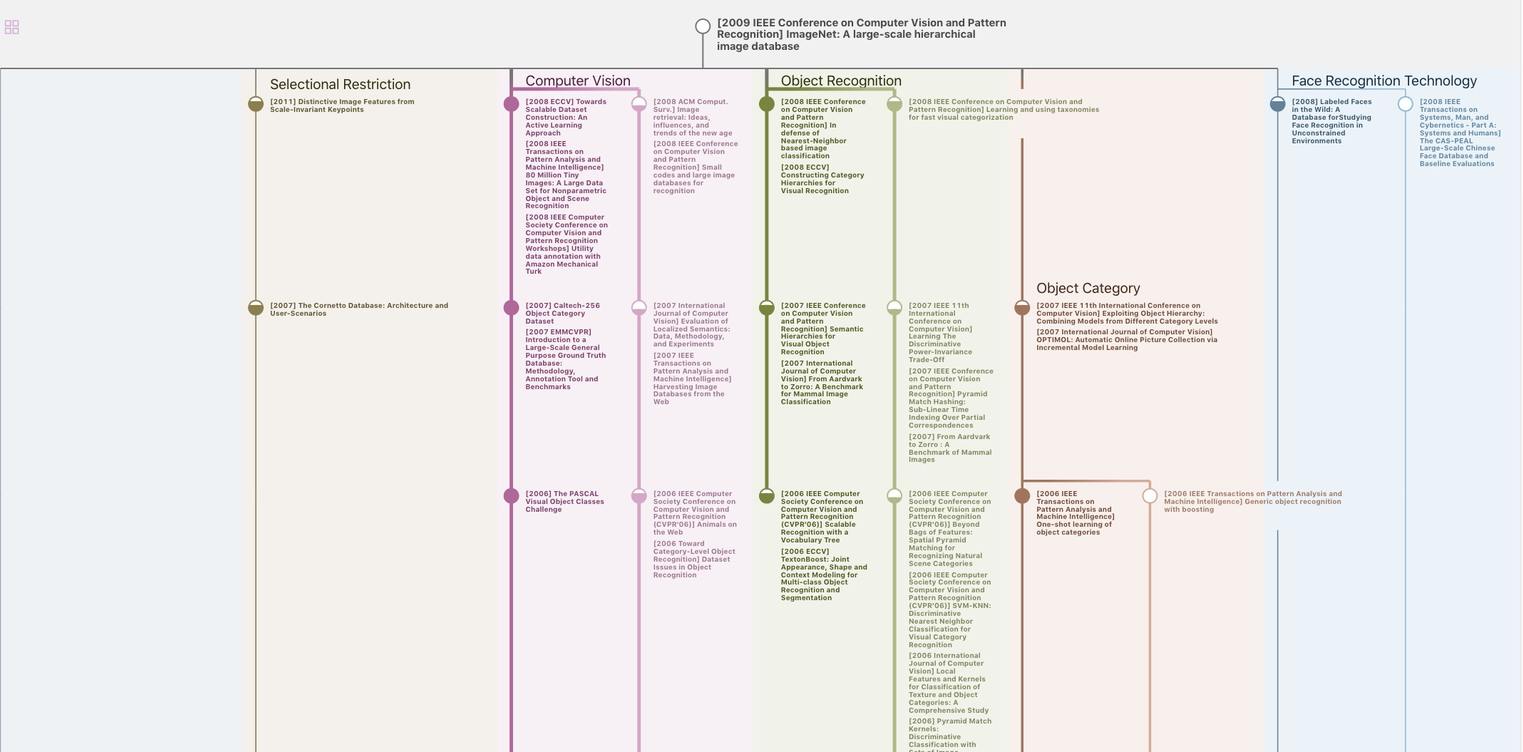
生成溯源树,研究论文发展脉络
Chat Paper
正在生成论文摘要