A long short-term memory neural network-based error estimator for three-dimensional dynamically adaptive mesh generation
Physics of Fluids(2023)
摘要
Adaptive meshes are pivotal in numerical modeling and simulation, offering a means to efficiently, precisely, and flexibly represent intricate physical phenomena, particularly when grappling with their intricacies and varying scales. However, the transition from two dimensions (2D) to three dimensions (3D) poses a substantial challenge, as the computational demands of dynamically adaptive mesh techniques increase exponentially. Addressing this challenge effectively, we turn to the cutting-edge realm of artificial intelligence and neural networks. In our study, we harness the innovative power of a long short-term memory (LSTM) neural network as an error estimator for adapting unstructured meshes in both 2D and 3D scenarios. This LSTM network predicts the evolution of the adaptive grid based on specified variables, presenting itself as an artificial intelligence-driven architecture to optimize the adaptive criterion for the target variable. This is achieved by establishing a direct correspondence between the Riemann metric and these variables. To demonstrate the practical applicability of our approach, we seamlessly integrate the LSTM error estimator into the 3D adaptive atmospheric model Fluidity-Atmosphere (Fluidity-Atmos), thereby enabling real-time mesh adaptation during numerical simulations. We assess the effectiveness of this method in terms of simulation precision and computational efficiency through a series of experiments in both 2D and 3D settings. Our results not only reveal that the mesh patterns generated by the LSTM error estimator within Fluidity-Atmos closely resemble those produced by traditional error estimators but also underscore its superior performance in enhancing simulation accuracy. Notably, as the number of nodes increases, the LSTM mesh generator substantially reduces CPU time requirements by up to 50% in 3D cases compared to the conventional mesh generator within Fluidity-Atmos, highlighting its remarkable computational efficiency.
更多查看译文
关键词
adaptive,error estimator,short-term,network-based,three-dimensional
AI 理解论文
溯源树
样例
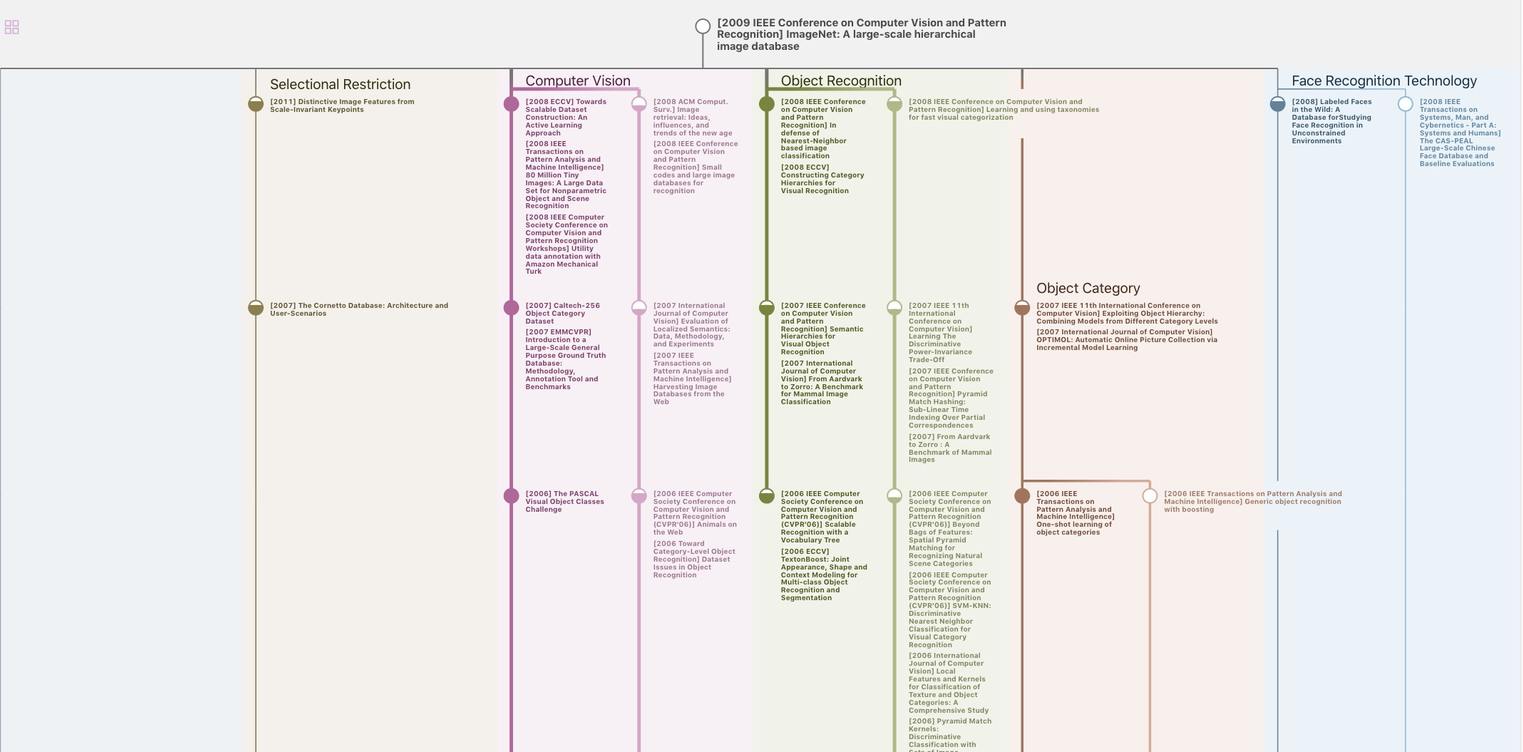
生成溯源树,研究论文发展脉络
Chat Paper
正在生成论文摘要