Pitfalls of Using Multinomial Regression Analysis to Identify Class-Structure-Relevant Variables in Biomedical Data Sets: Why a Mixture of Experts (MOE) Approach Is Better
BioMedInformatics(2023)
摘要
Recent advances in mathematical modeling and artificial intelligence have challenged the use of traditional regression analysis in biomedical research. This study examined artificial data sets and biomedical data sets from cancer research using binomial and multinomial logistic regression. The results were compared with those obtained with machine learning models such as random forest, support vector machine, Bayesian classifiers, k-nearest neighbors, and repeated incremental clipping (RIPPER). The alternative models often outperformed regression in accurately classifying new cases. Logistic regression had a structural problem similar to early single-layer neural networks, which limited its ability to identify variables with high statistical significance for reliable class assignments. Therefore, regression is not per se the best model for class prediction in biomedical data sets. The study emphasizes the importance of validating selected models and suggests that a “mixture of experts” approach may be a more advanced and effective strategy for analyzing biomedical data sets.
更多查看译文
关键词
multinomial regression analysis,biomedical data sets,experts,class-structure-relevant
AI 理解论文
溯源树
样例
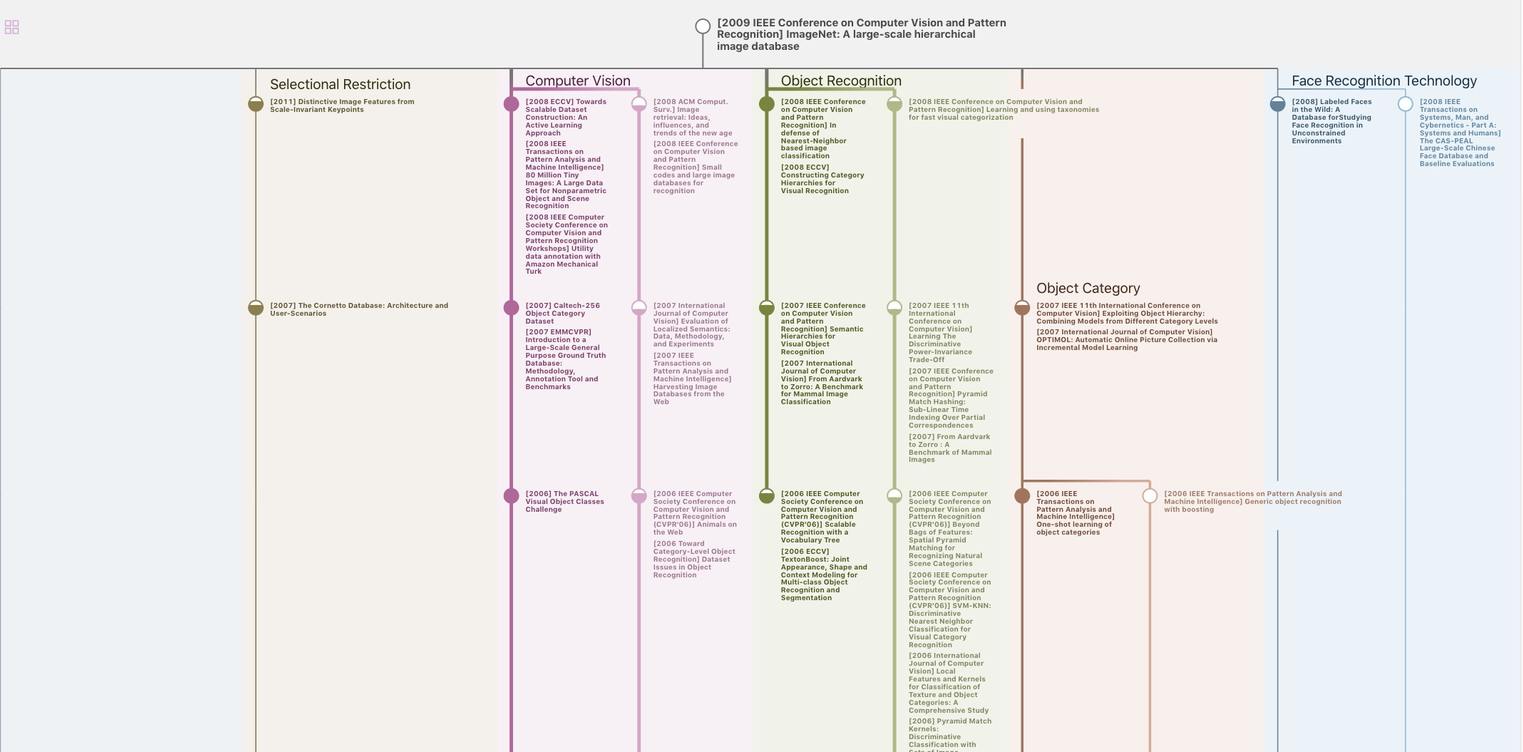
生成溯源树,研究论文发展脉络
Chat Paper
正在生成论文摘要