Double-Quantitative Feature Selection Approach for Multi-Granularity Ordered Decision Systems
IEEE transactions on artificial intelligence(2023)
摘要
Double-quantitative-based granular computing implies the systematic perspective, completeness and accuracy of rough approximation. However, most of existing researches only focus on the case of single quantification, and there are few research study on the simultaneous computing method of double quantification. In this paper, we explore feature selection with double quantification in multi-granularity ordered decision systems (MG-ODSs). Firstly, the related concepts of quantitative functions are interpreted from different viewpoints of relative and absolute quantification. Then, the multi-granularity double-quantitative rough sets in an ordered decision system (ODS) from optimistic and pessimistic cases, the related properties, and three-way decisions based on the presented quantitative levels are discussed. Furthermore, the greedy algorithm for feature selection is derived. By using twelve datasets from public repository, evaluations and comparisons are made on the parameter setting and classification accuracy. From these comparative experiments, advantages and effectiveness of the proposed feature selection algorithm could be demonstrated over the existing approaches.
更多查看译文
关键词
Double quantification,Feature selection,Granular computing,Ordered decision systems
AI 理解论文
溯源树
样例
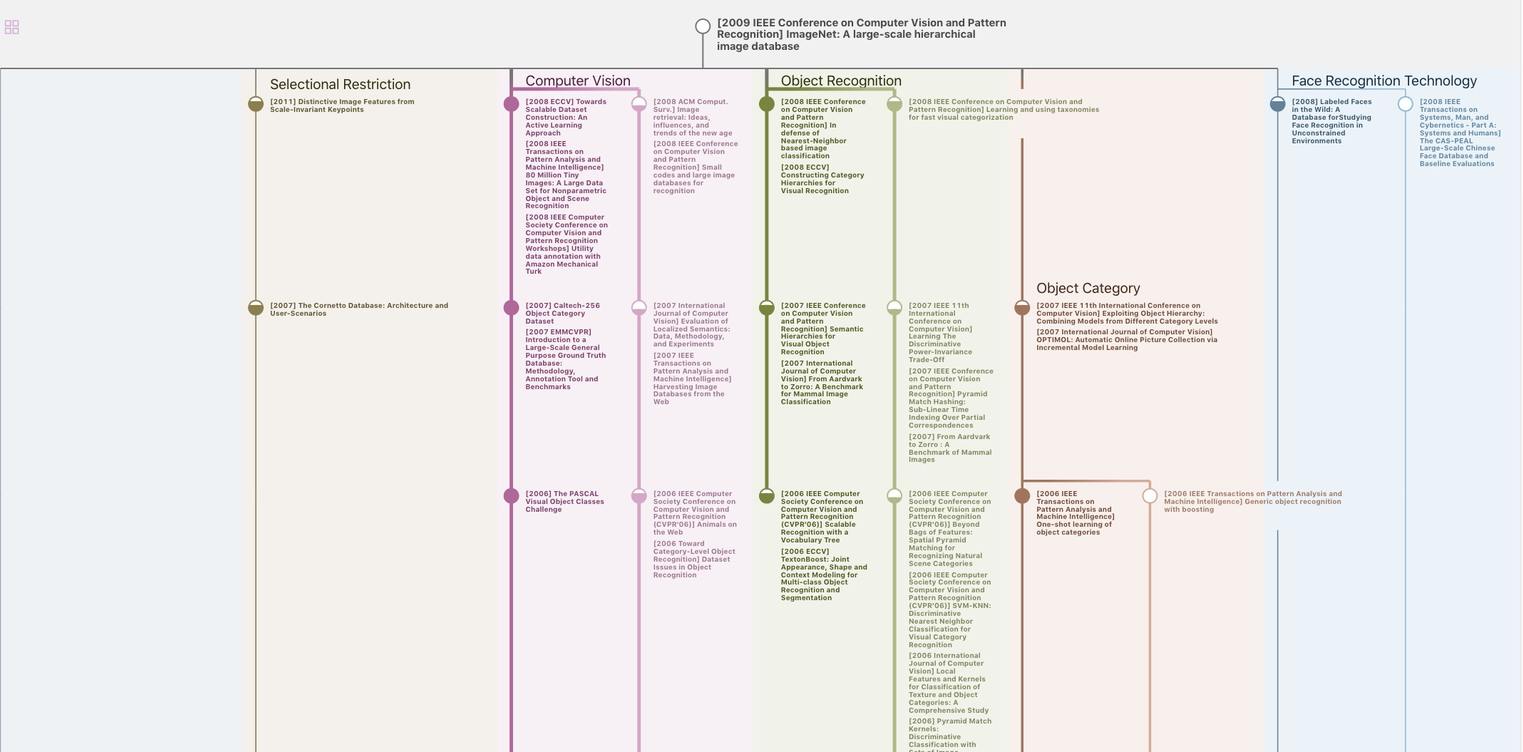
生成溯源树,研究论文发展脉络
Chat Paper
正在生成论文摘要