Enhanced mammogram classification with convolutional neural network: An improved algorithm for automated breast cancer detection
MEASUREMENT(2023)
Abstract
The current use of medical image processing can be employed to detect various diseases or tumors present in the human body. Breast cancer, which predominantly affects women and can result in death, can be identified at an early stage using mammography, a special screening method of computer tomography (CT) scan, which may potentially save the patient's life. Despite advancements in medical imaging technology, radiologists encounter difficulties in precisely discriminating between non-cancerous and cancerous brain tumors owing to their cellular composition. This study aims to solve the issue of automatic detection of breast cancer utilizing efficient image processing methods. The quality of mammography pictures is increased using Non-Local-Means (NLM) filtering, adaptive histogram equalization (AHE), and clustering with a morphological technique. The Gray Level Run Length Matrix (GLRLM), which considers the shape, surface, and location of the breast tumour cells, is utilized to extract important details from the mammography image. The K-nearest neighbor (K-NN) method is used to categorize the image as normal or abnormal based on the selected attributes. Using a kernel-based convolutional neural network, the tumour is then isolated from the aberrant image and categorized as benign or malignant (KBCNN). This approach has demonstrated improved precision compared to existing methods, achieving more than 95% accuracy.
MoreTranslated text
Key words
Breast Cancer,Mammogram,Convolutional Neural Network,Machine Learning Algorithms,Tumors,Adaptive histogram equalization
AI Read Science
Must-Reading Tree
Example
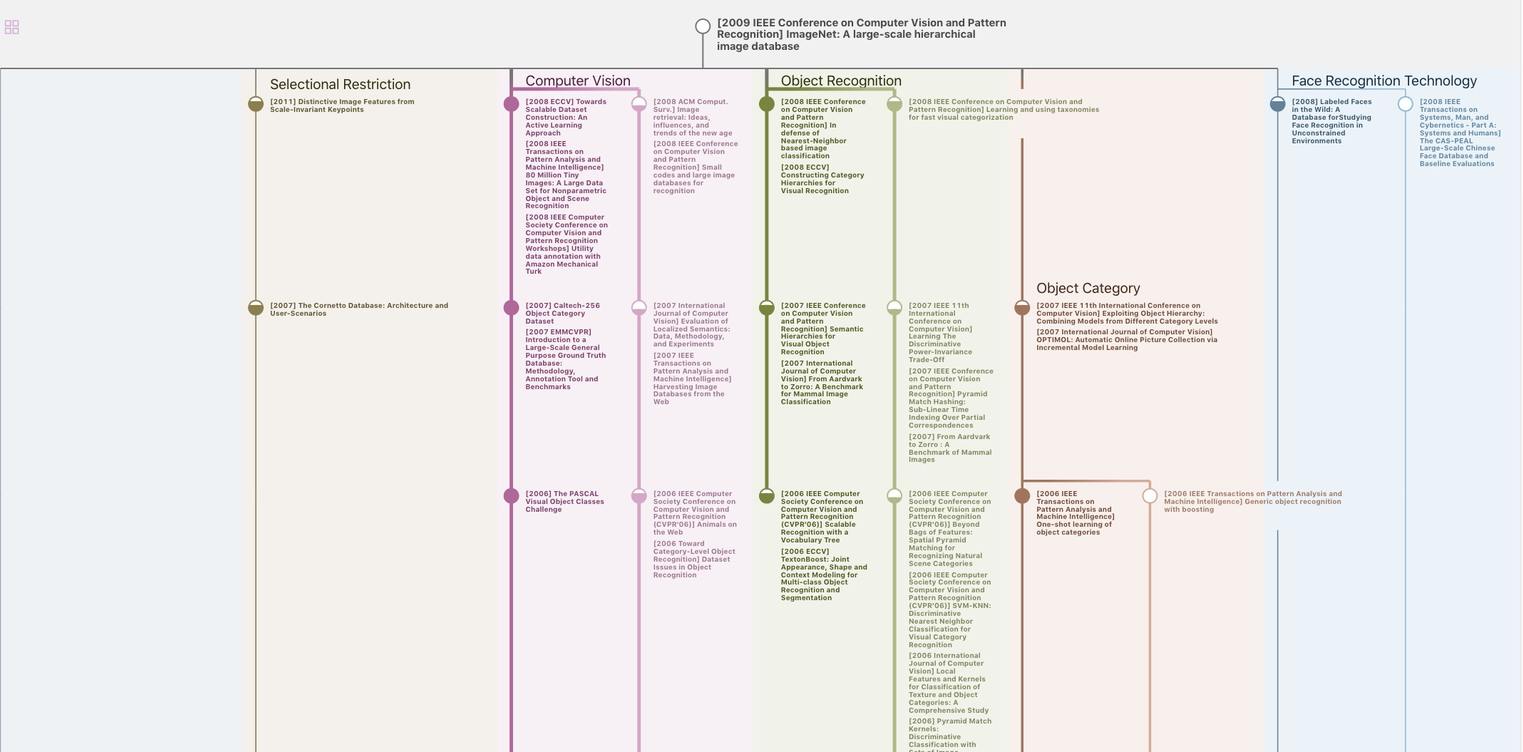
Generate MRT to find the research sequence of this paper
Chat Paper
Summary is being generated by the instructions you defined