Coarse-to-Fine Nutrition Prediction.
IEEE Trans. Multim.(2024)
摘要
Healthy dietary intake has a broad influence on the quality of life, and nutrition prediction plays a great role in the auxiliary decision-making of diet. Given a food image, existing nutrition prediction methods directly regress the nutrition content. However, due to the complex variations in food images, such as differences in viewpoint and lighting conditions, directly regressing the nutrition content faces significant challenges. The complexity of the food image data results in a high-dimensional and feature-rich input space, which poses difficulties for traditional regression models to efficiently navigate and optimize. Consequently, the direct regression paradigm usually generates inaccurate nutrition predictions. To alleviate the ambiguity challenge in the prediction progress, we propose to narrow the searchable space for the model's predictions by decomposing the direct regression into two steps: first coarsely selecting the nutrition scope and then finely refining the prediction value, forming a coarse-to-fine nutrition prediction paradigm. Although the process of coarse prediction which selects a bin from a series of scope bins can be formulated as a standard classification problem, it exhibits a distinguishable characteristic, i.e. the closer to the ground truth bin, the less punishment in the training phase. However, most of the current methods have ignored this phenomenon, thus, we specially design the linearly smoothed label in the nutrition prediction task to reveal the relative distance to the ground truth bin, leading to extraordinary improvements. Furthermore, we conduct a pair-wise comparison among all bins by extending the 1D label into 2D space and propose the structure loss to guide the bin selection process effectively. Due to the narrowed decision space, the nutrition prediction problem can be effectively optimized, and the proposed method achieves promising results on three benchmarks ECUSTFD, VFD and Nutrition5K, demonstrating the efficiency of the coarse-to-fine paradigm equipped with the linear-smoothed structure loss.
更多查看译文
关键词
Nutrition Prediction,Coarse-to-fine,Multi-task Learning,Label Smoothing
AI 理解论文
溯源树
样例
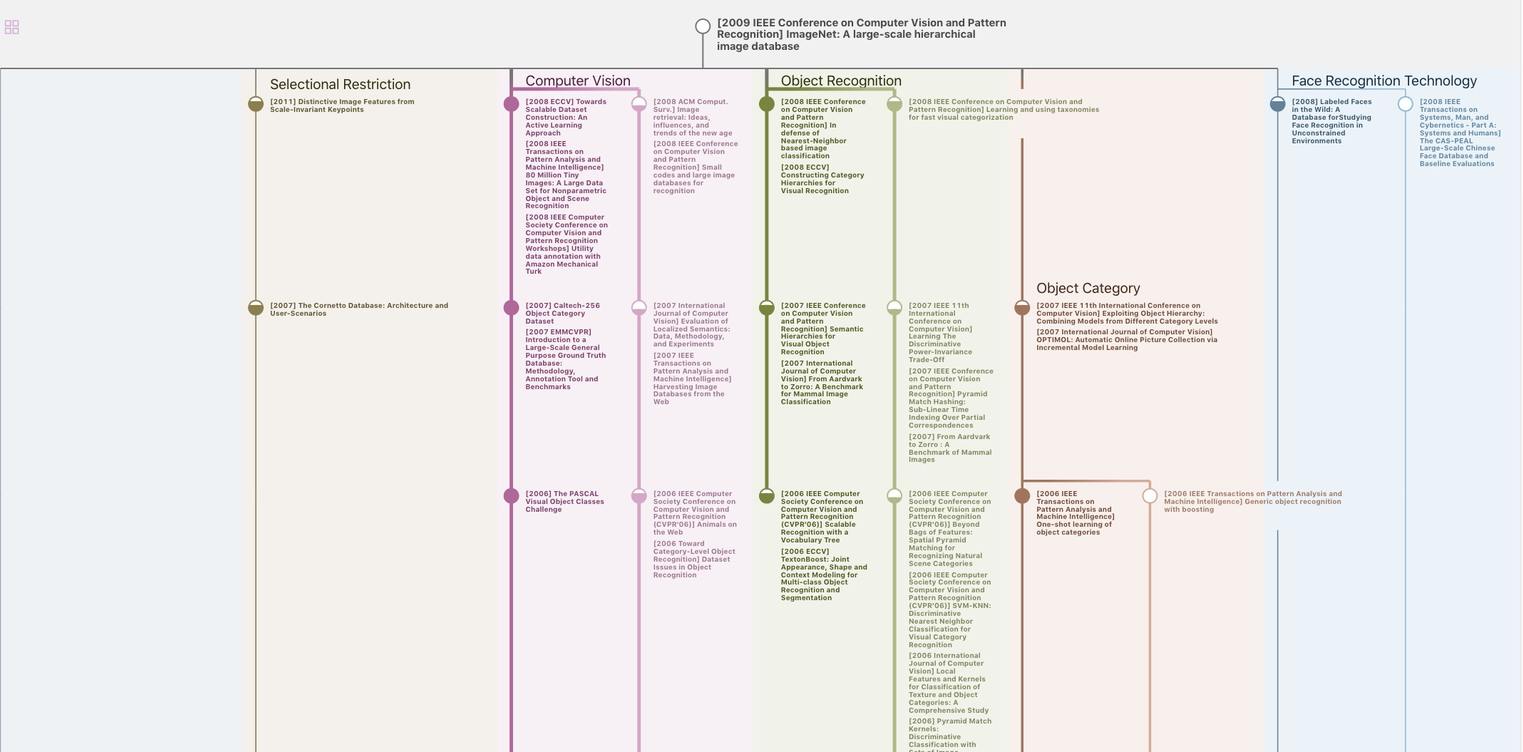
生成溯源树,研究论文发展脉络
Chat Paper
正在生成论文摘要