Development and Evaluation of Intersection-Based Turning Movement Counts Framework Using Two Channel LiDAR Sensors
Journal of Transportation Technologies(2023)
摘要
This paper presents vehicle localization and tracking methodology to utilize two-channel LiDAR data for turning movement counts. The proposed methodology uniquely integrates a K-means clustering technique, an inverse sensor model, and a Kalman filter to obtain the final trajectories of an individual vehicle. The objective of applying K-means clustering is to robustly differentiate LiDAR data generated by pedestrians and multiple vehicles to identify their presence in the LiDAR’s field of view (FOV). To localize the detected vehicle, an inverse sensor model was used to calculate the accurate location of the vehicles in the LiDAR’s FOV with a known LiDAR position. A constant velocity model based Kalman filter is defined to utilize the localized vehicle information to construct its trajectory by combining LiDAR data from the consecutive scanning cycles. To test the accuracy of the proposed methodology, the turning movement data was collected from busy intersections located in Newark, NJ. The results show that the proposed method can effectively develop the trajectories of the turning vehicles at the intersections and has an average accuracy of 83.8%. Obtained R-squared value for localizing the vehicles ranges from 0.87 to 0.89. To measure the accuracy of the proposed method, it is compared with previously developed methods that focused on the application of multiple-channel LiDARs. The comparison shows that the proposed methodology utilizes two-channel LiDAR data effectively which has a low resolution of data cluster and can achieve acceptable accuracy compared to multiple-channel LiDARs and therefore can be used as a cost-effective measure for large-scale data collection of smart cities.
更多查看译文
关键词
channel lidar sensors,turning movement counts framework,intersection-based
AI 理解论文
溯源树
样例
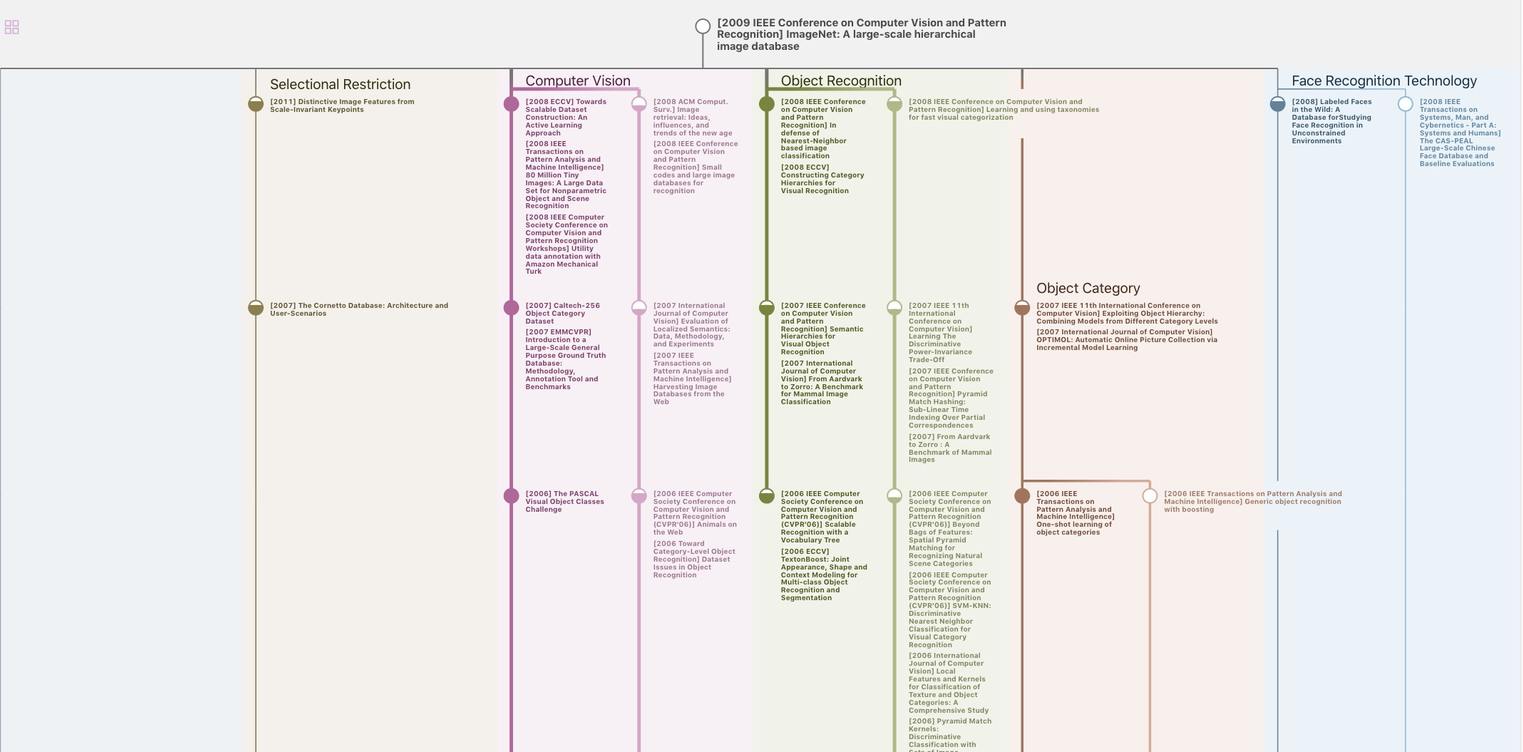
生成溯源树,研究论文发展脉络
Chat Paper
正在生成论文摘要