Diffusion-based kernel density estimation improves the assessment of carbon isotope modelling
arXiv (Cornell University)(2023)
摘要
Comparing differently sized data sets is one main task in model assessment and calibration. This is due to field data being generally sparse compared to simulated model results. We tackled this task by the application of a new diffusion-based kernel density estimator (diffKDE) that approximates probability density functions of a data set nearly independent of the amount of available data. We compared the resulting density estimates of measured and simulated marine particulate organic carbon-13 isotopes qualitatively and quantitatively by the Wasserstein distance. For reference we also show the corresponding comparison based on equally sized data set with reduced simulation and field data. The comparison based on all available data reveals a better fit of the simulation to the field data and shows misleading model properties in the masked analysis. A comparison between the diffKDE and a traditional Gaussian KDE shows a better resolution of data features under the diffKDE. We are able to show a promising advantage in the application of KDEs in calibration of models, especially in the application of the diffKDE.
更多查看译文
关键词
kernel density estimation,isotope,carbon,diffusion-based
AI 理解论文
溯源树
样例
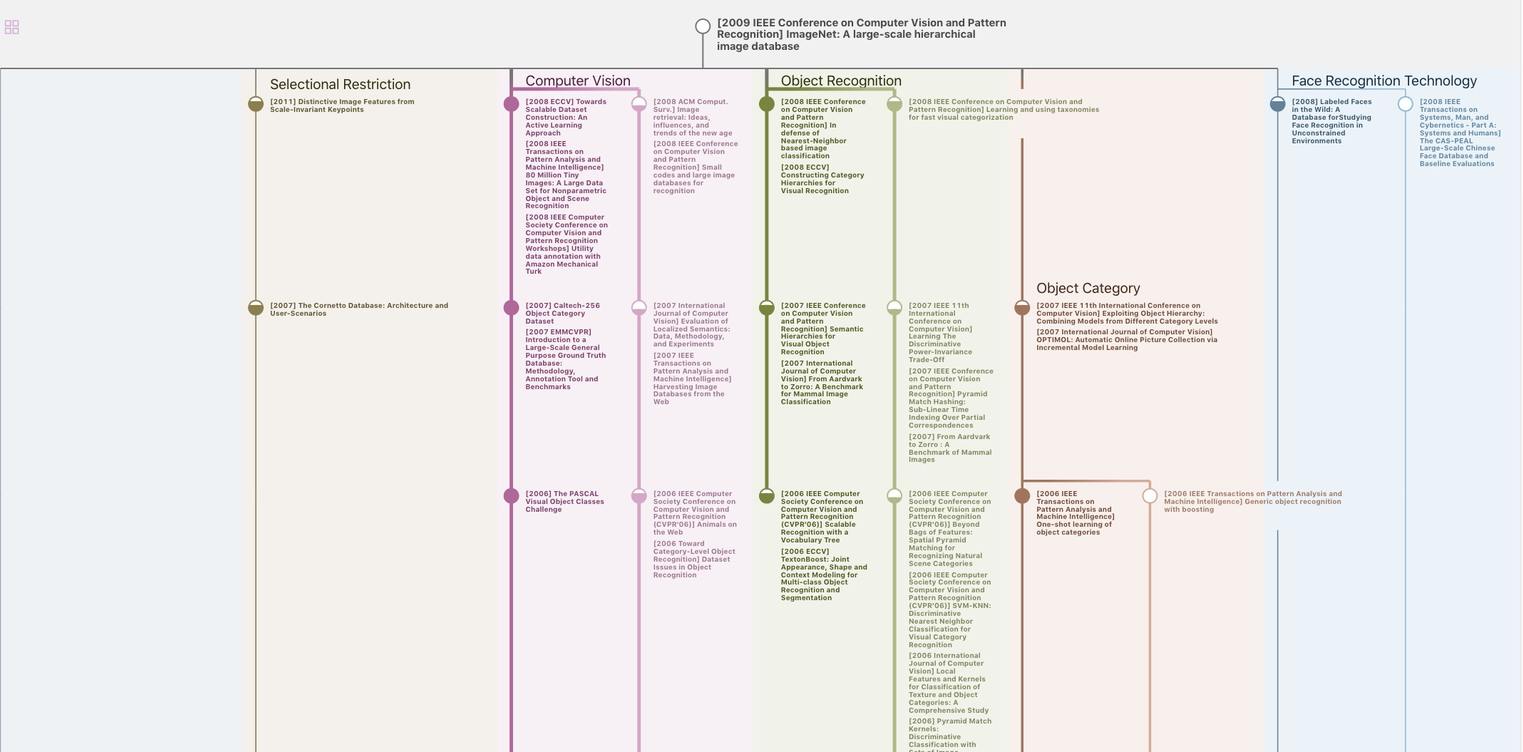
生成溯源树,研究论文发展脉络
Chat Paper
正在生成论文摘要