Machine Learning Algorithm Integrated with Real-Time In Situ Sensors and Physiochemical Principle-Driven Soft Sensors toward an Anaerobic Digestion-Data Fusion Framework
ACS ES&T water(2023)
摘要
Insufficient real-time in situ data and complicated model parameters are severe barriers for accurate prediction and precise control of anaerobic digestion (AD) systems. This study addresses the crucial challenge by developing an advanced data acquisition technology integrating Milli-electrode Array Physical Sensors (MAPS) with Anaerobic Digestion model No.1-based Soft Sensors (ADSS) and an interpretable machine learning algorithm (MLA) equipped with post hoc analysis. AD system mechanisms are retained in hybrid physical/soft sensor data sets at the mechanistic level and can be integrated into MLA through training at the data level. The MAPS-ADSS data exhibited deviations of less than 5% from the lab-based evaluations. The integrated MAPS-ADSS-MLA methodology improved the prediction accuracy from 91% (nonlinear MLA) and 78% (linear MLA) to 99% compared to conventional MLA. Furthermore, the SHapley Additive exPlanation values and Person (r) and Spearman (rs) correlation coefficients of the predictions identified the indirect impacts of pH, alkalinity, and NH4+ on biogas production via their adverse correlation with volatile fatty acid (r = −0.93, rs = −0.92). This study presents an innovative AD-Data fusion platform for accurate and interpretable predictions in complex wastewater systems, using a sensor-model-MLA loop and employing data as the carrier to integrate physiochemical principles into training and analysis.
更多查看译文
关键词
soft sensors,anaerobic,machine learning,real-time,principle-driven,digestion-data
AI 理解论文
溯源树
样例
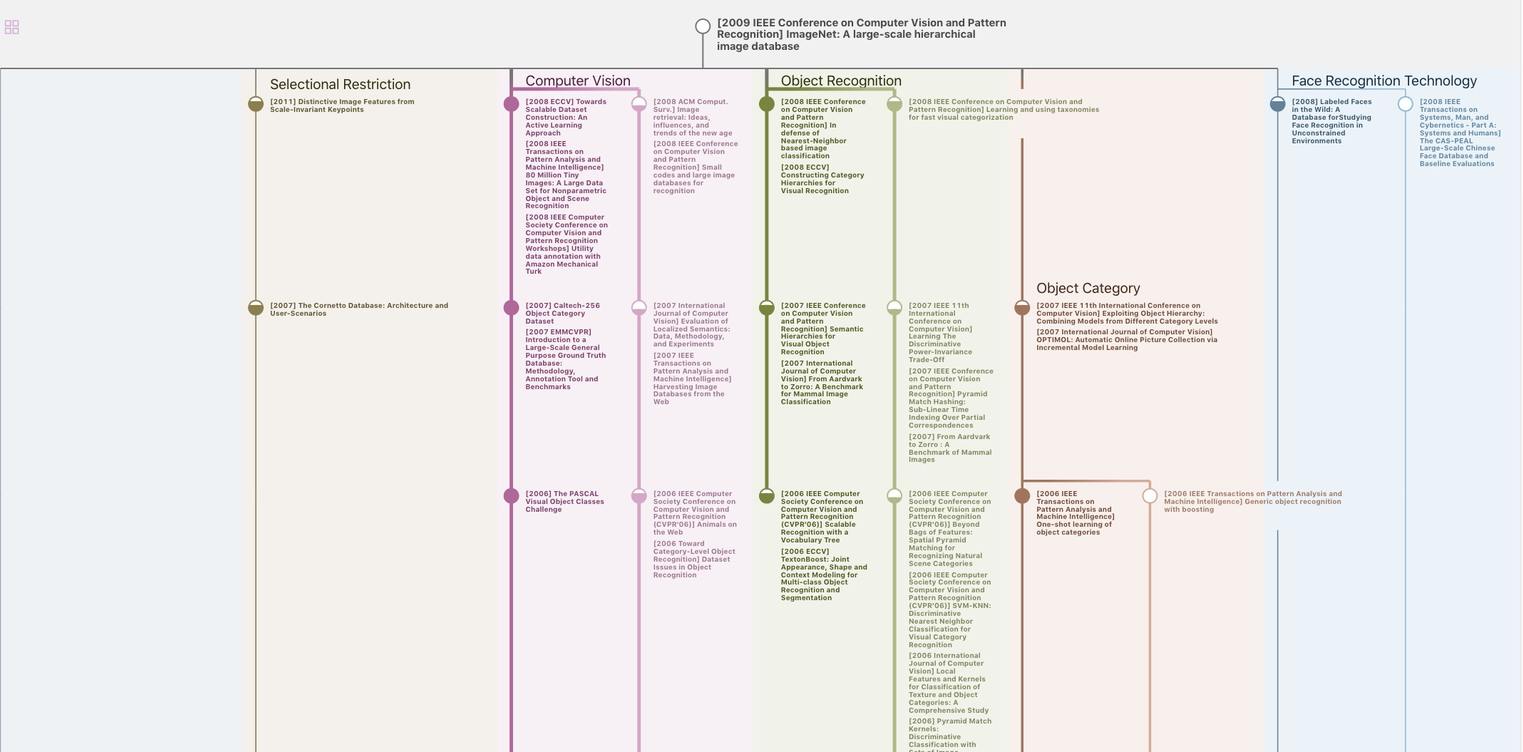
生成溯源树,研究论文发展脉络
Chat Paper
正在生成论文摘要