Chapter 7. Neural-Symbolic Interaction and Co-Evolving
Frontiers in artificial intelligence and applications(2023)
Abstract
Symbolic knowledge is vital in various human cognitive functions, including reasoning, skill acquisition, and communication. Its integration is also essential for creating AI with human-like capabilities, such as robustness, creativity, and interpretability. Nevertheless, current machine learning approaches still dominantly emphasize learning from large data sets, struggling to effectively and scalably incorporate symbolic knowledge. This leads to fundamental limitations, such as brittle results when faced with complex or novel concepts, and difficulty in understanding or explaining the decision processes of models. Past attempts at integrating symbolic information with neural networks frequently rely on manually created knowledge bases that are defined in specific configurations, thereby impeding their generalizability to new applications and domains. This chapter seeks to introduce interaction and co-evolving mechanisms between neural models and symbolic knowledge bases. It starts from constructing a panoramic learning framework for learning with all experiences (data, rules, knowledge graphs, etc.). Subsequently, it delves into a novel inversion problem of extracting symbolic knowledge from black-box neural models. Finally, based on the components mentioned above, the chapter will explore a blueprint of a lifelong neural-symbolic system that accommodates human intervention.
MoreTranslated text
Key words
interaction,neural-symbolic,co-evolving
AI Read Science
Must-Reading Tree
Example
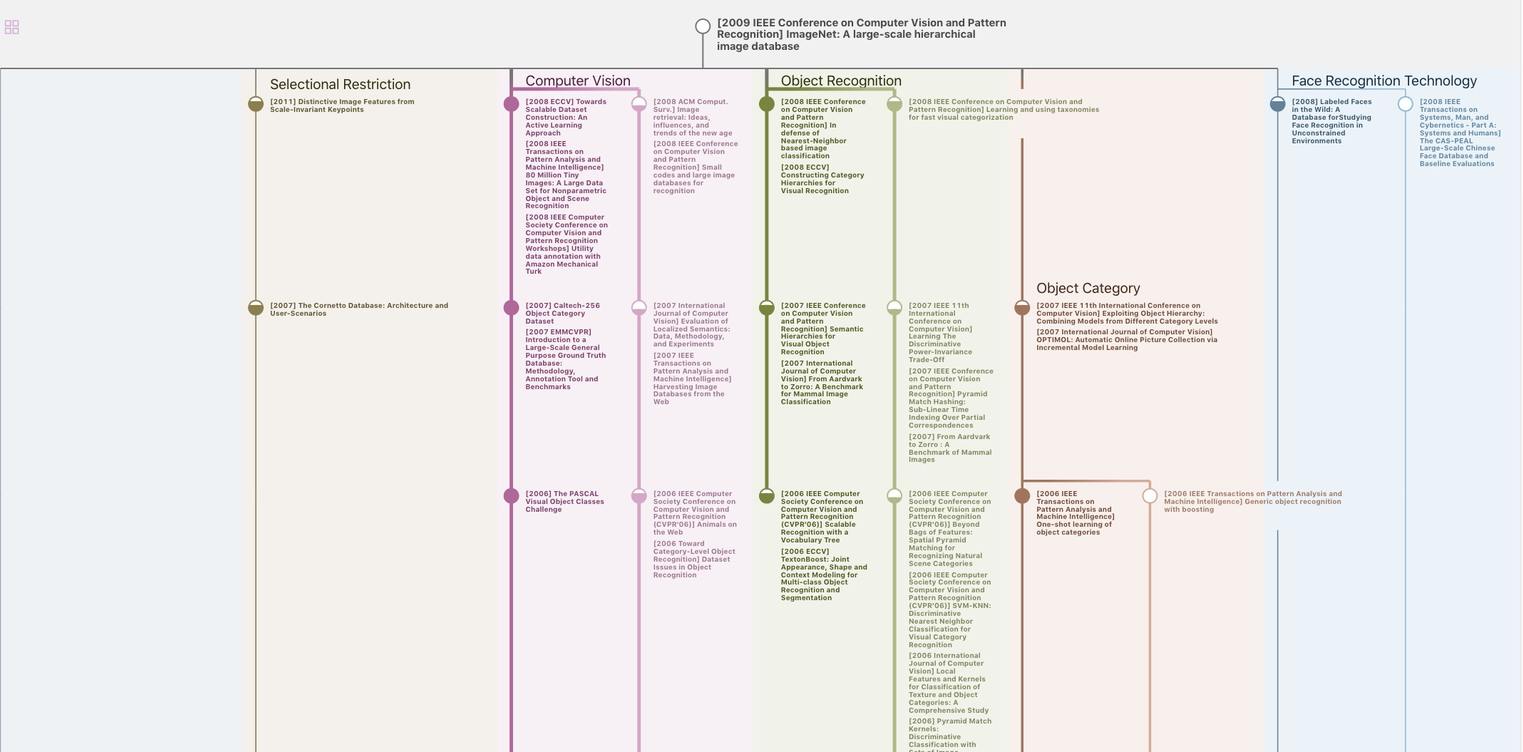
Generate MRT to find the research sequence of this paper
Chat Paper
Summary is being generated by the instructions you defined