An exploratory model-based design of experiments technique to aid parameters identification and reduce prediction uncertainty
Computer-aided chemical engineering(2023)
摘要
When developing mathematical models to describe reaction processes, model parameters require to be estimated from experimental data. Experiments are traditionally designed through techniques aiming at space exploration, like space-filling methods (e.g., Latin Hypercube sampling or LHS), or at information maximization, like model-based design of experiments (MBDoE). However, the former methods do not minimize parameters uncertainty, while the latter do not ensure a minimization of model prediction uncertainty in the entire experimental design space. In this work, we propose a novel exploratory MBDoE (eMBDoE) approach based on G-optimality calculation (G-map eMBDoE) to simultaneously enhance space exploration and minimize model prediction variance. The method is tested on a case study related to the identification of kinetic parameters of catalytic total methane oxidation in a flow microreactor. Results show that the method is more explorative than conventional MBDoE and more efficient than LHS and MBDoE in reducing model prediction uncertainty and parameters uncertainty.
更多查看译文
关键词
experiments technique,prediction uncertainty,aid parameters identification,model-based
AI 理解论文
溯源树
样例
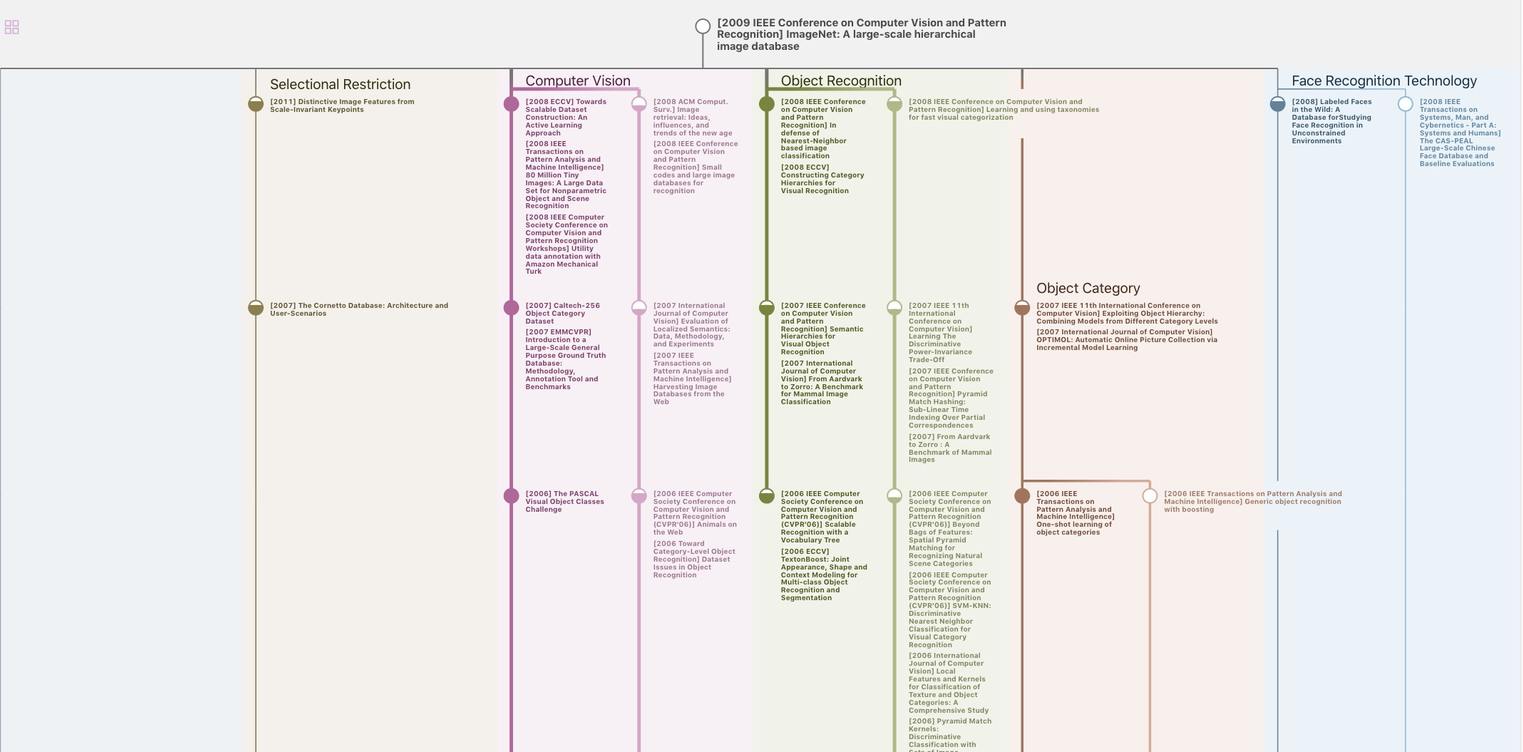
生成溯源树,研究论文发展脉络
Chat Paper
正在生成论文摘要