Data-driven prediction of peptide-MHC binding using oscillations of physicochemical properties
Computer-aided chemical engineering(2023)
摘要
The Major Histocompatibility Complex (MHC) molecules play a major role in T-cell immunogenicity through the recognition of 'non-self' peptides derived from foreign antigens. Therefore, predicting peptides that trigger immune responses is of great interest for the general understanding of T-cell-mediated immunity and the design of peptide-based vaccines and cancer immunotherapy treatments. The presented work tests the performance of allele-specific SVM classification models in the prediction of pMHC binding. The models aim to classify MHC class II binding and non-binding peptides based on their amino acid sequences and derived features. In developing the models, we take advantage of underlying periodicities in physicochemical properties along the sequence of a peptide that has been shown to be predictive of protein structure and function. Once the physicochemical descriptors are generated, Fourier transforms are then applied to be able to encode peptide sequences of varying lengths. In training and testing the model, a comprehensive dataset of MHC class II binding peptides that includes 44 unique MHC class II alleles (molecules) with 60630 binding affinities is taken from the IEDB database. Cross-validation and hyperparameter tuning are applied across multiple train and test datasets. A feature selection algorithm is also incorporated into the model development to identify an essential set of predictive features. The blind test set prediction accuracy of the developed allele-specific models ranges from 0.58- 0.93, with an average classification accuracy of 0.73.
更多查看译文
关键词
physicochemical properties,binding,oscillations,data-driven,peptide-mhc
AI 理解论文
溯源树
样例
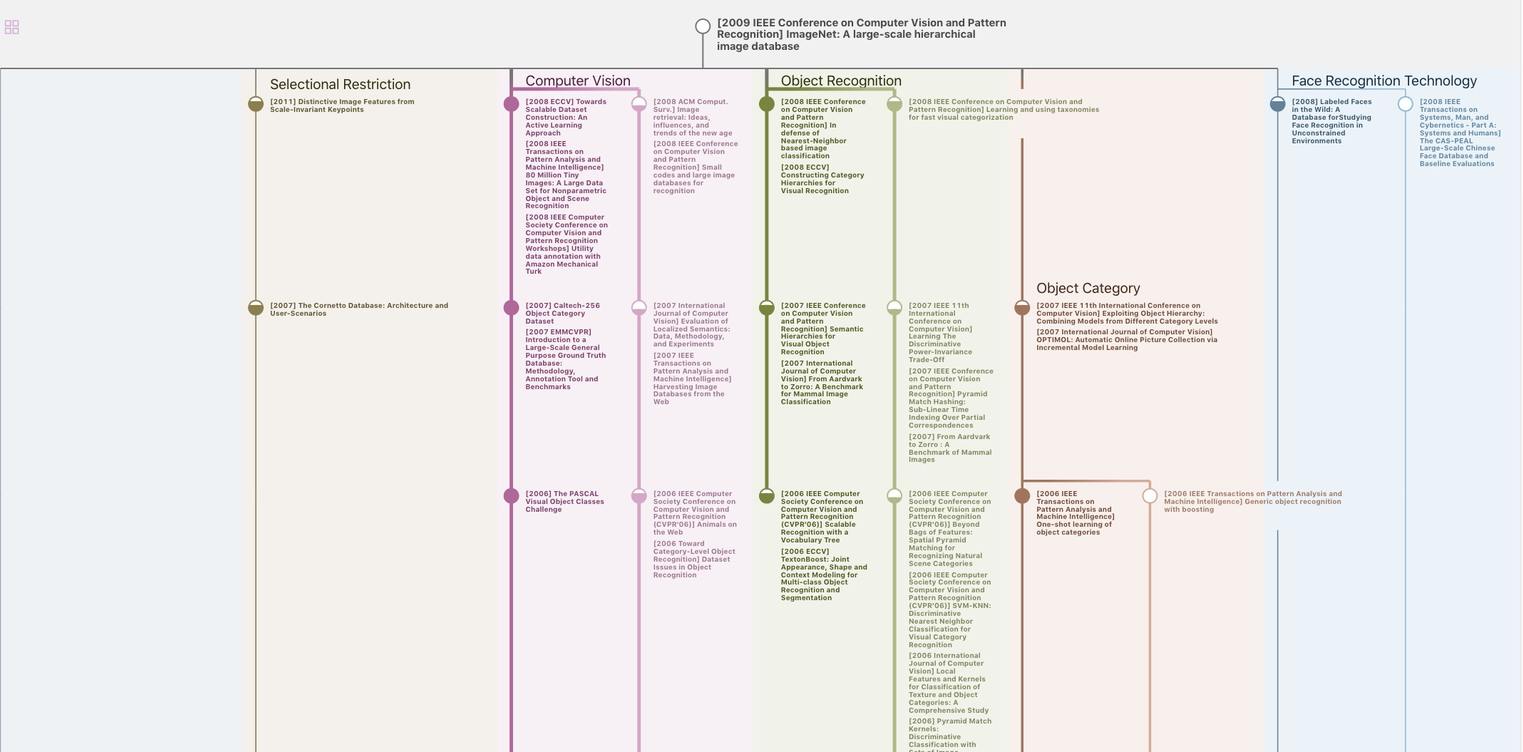
生成溯源树,研究论文发展脉络
Chat Paper
正在生成论文摘要