Online Query Expansion Hashing for Efficient Image Retrieval
IEEE TRANSACTIONS ON CIRCUITS AND SYSTEMS FOR VIDEO TECHNOLOGY(2024)
摘要
Unsupervised hashing has the desirable advantages of label independence, high storage, and retrieval efficiency, which is suitable for scalable image retrieval. Most existing methods focus on enhancing the image hashing model training process at the offline stage. However, little attention has been paid to the query content analysis by them at the online retrieval stage. They still suffer from important query semantic shortages, and thus limit the online retrieval performance, which is the ultimate objective of the image retrieval system. In this paper, we propose an Online Query Expansion Hashing (OQEH) for efficient image retrieval, by adaptively enhancing the discriminative capability of query hash codes in an expansion manner at the online retrieval stage. Specifically, we first design a self-expansion network to learn semantically invariant feature representations from images and their visual augmentations. Then, we conduct neighborhood-expansion to search similar samples for each image from a query expansion set with the semantically invariant features and design a Transformer architecture to adaptively transfer the semantics of neighbor samples to their corresponding images. With the support of semantically invariant features, query expansion set, and adaptive semantic transfer, the representation capability of query hash codes can be enhanced at the online retrieval stage. Experimental results demonstrate that the proposed OQEH method achieves superior retrieval accuracy and comparable retrieval efficiency compared with the state-of-the-art methods. Particularly, on MS COCO dataset, OQEH can obtain about 6% performance improvement compared with the state-of-the-art results.
更多查看译文
关键词
Semantics,Codes,Training,Image retrieval,Image reconstruction,Adaptation models,Visualization,unsupervised deep hashing,query expansion,transformer
AI 理解论文
溯源树
样例
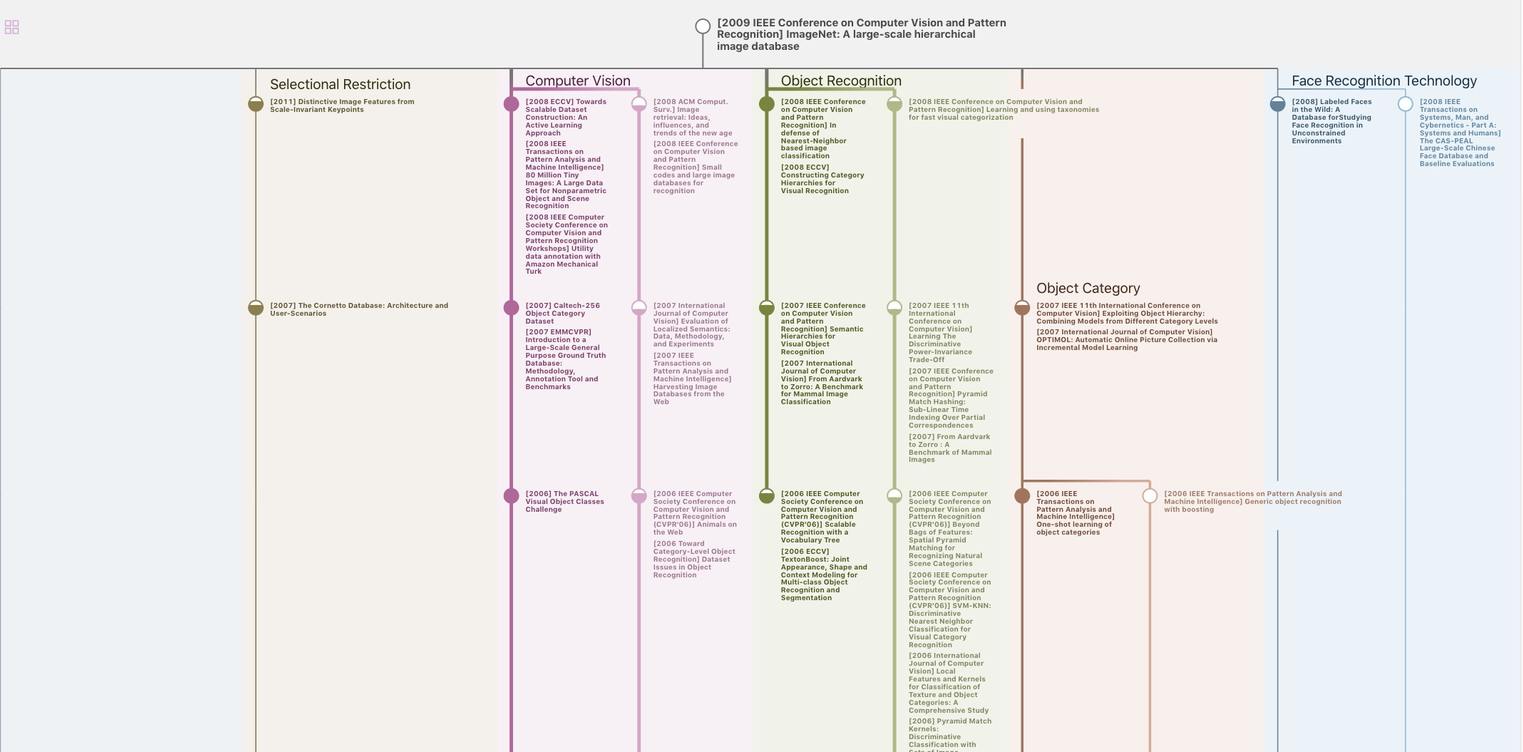
生成溯源树,研究论文发展脉络
Chat Paper
正在生成论文摘要