Lithium-Plating Suppressed and Deep Deterministic Policy Gradient Based Energy Management Strategy
IEEE Transactions on Transportation Electrification(2023)
摘要
Lithium plating and the formation of lithium dendrite are the main causes of battery internal short circuit and thermal runaway. This paper proposes a deep reinforcement learning-based energy management framework for power-split hybrid electric vehicles (HEVs) which effectively suppresses lithium plating inside batteries. First, single particle model with electrolyte (SPMe) and lithium-plating model are integrated into deep deterministic policy gradient based energy management strategy (DDPG-EMS) for the first time. Second, hybrid particle swarm optimization grey wolf optimizer (HPSOGWO) algorithm is applied to the parameter identification of the two models for the first time to ensure the optimal trade-off between convergence speed and identification accuracy. Third, the superiority of the control strategy is achieved by balancing among fuel consumption, lithium plating occurrence, and SOC sustainability effect under different initial cell temperatures, and verified by comparison with deep Q-network (DQN). The training and validation results both show that the proposed strategy with lithium plating considered not only slows down battery degradation by redressing the charging current in time before the negative overpotential drops to zero, but also reduces the fuel consumption cost as well as total cost.
更多查看译文
关键词
Hybrid electric vehicles (HEVs),energy management strategy (EMS),deep reinforcement learning (DRL),Lithium plating,battery electrochemical model
AI 理解论文
溯源树
样例
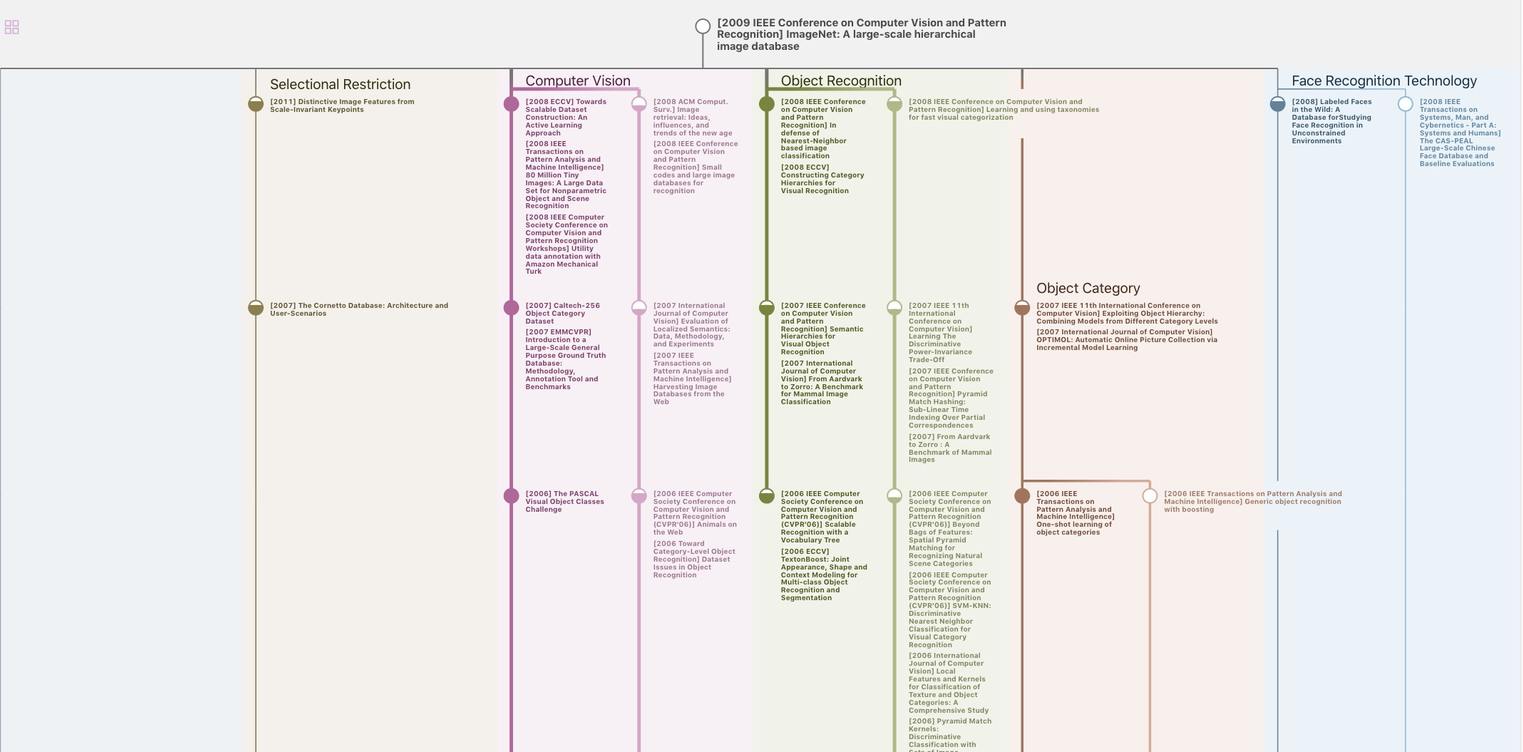
生成溯源树,研究论文发展脉络
Chat Paper
正在生成论文摘要