Machine learning model to predict the presence of paroxysmal atrial fibrillation in patients with hypertrophic cardiomyopathy
European Heart Journal - Cardiovascular Imaging(2023)
摘要
Abstract Funding Acknowledgements Type of funding sources: Public grant(s) – National budget only. Main funding source(s): Romanian Ministry of National Education, CNCS-UEFISCDI. Background Atrial fibrillation (AF) is the most frequent arrhythmia in hypertrophic cardiomyopathy (HCM), with a major impact on overall survival, thromboembolic risk, and quality of life. Early recognition and treatment of AF are essential to improve the outcome of HCM patients (pts). Despite the existence of several independent predictors of AF development, the identification of HCM pts at risk for AF is still inconsistent. The identification, quantification, and interpretation of the relationships between different clinical and imaging-derived variables may lead to improved risk stratification and prognosis. Predictive models based on machine learning (ML) could reduce variability while providing useful new medical knowledge. Purpose Develop a ML model (emerging from the integration of clinical and echocardiographic data) capable of accurately detecting HCM pts with AF. Methods A comprehensive clinical and echocardiographic assessment was performed in 151 consecutive pts (52±16 years, 72 men) with HCM, in sinus rhythm. Pts were divided into two groups according to the presence (38 pts) or absence (113 pts) of documented paroxysmal AF (24/48 h ambulatory ECG recordings). 81 features (clinical and echocardiographic parameters) were considered as input to the ML models. Four different ML models were evaluated: Deep Learning (DL), Linear Logistic Regression (LLR), Support Vector Machine (SVM) and Random Forest (RF). Ensemble learning and four-fold cross-validation were employed in all experiments. For each experiment the training dataset was augmented and balanced using the Synthetic Minority Over-sampling Technique. The models were fine-tuned using the following hyper-parameters: learning rate, batch size, number of hidden layers, and number of features used as input. The features were first ranked using the Recursive Feature Elimination method, and the top N features were selected during the hyper-parameter tuning. Results Each ML model was trained for 100 epochs, and the results were extracted from the epoch that led to the best results when combining all four folds. DL was the best performing model, with a learning rate of 0.01, a batch size of 32, 4 hidden layers (256/128/128/64 neurons), and 15 input features. The DL model had 5% higher accuracy compared to LLR, 10% higher than SVM and 8% higher than RF, with consistently higher sensitivity. The performance of the various models is detailed in the table below. Moreover, the DL model had significantly higher accuracy compared to conventional imaging parameters consistently related to the presence of AF in previous studies, such as maximum LA volume (AUC 0.66, accuracy 59%) or LA strain (AUC 0.61, accuracy 62%). Conclusions The DL based model can detect the presence of paroxysmal AF in patients with HCM with an accuracy of 84% (5% higher than the best classic ML model, and significantly higher than independent conventional imaging parameters).
更多查看译文
关键词
paroxysmal atrial fibrillation,atrial fibrillation,machine learning
AI 理解论文
溯源树
样例
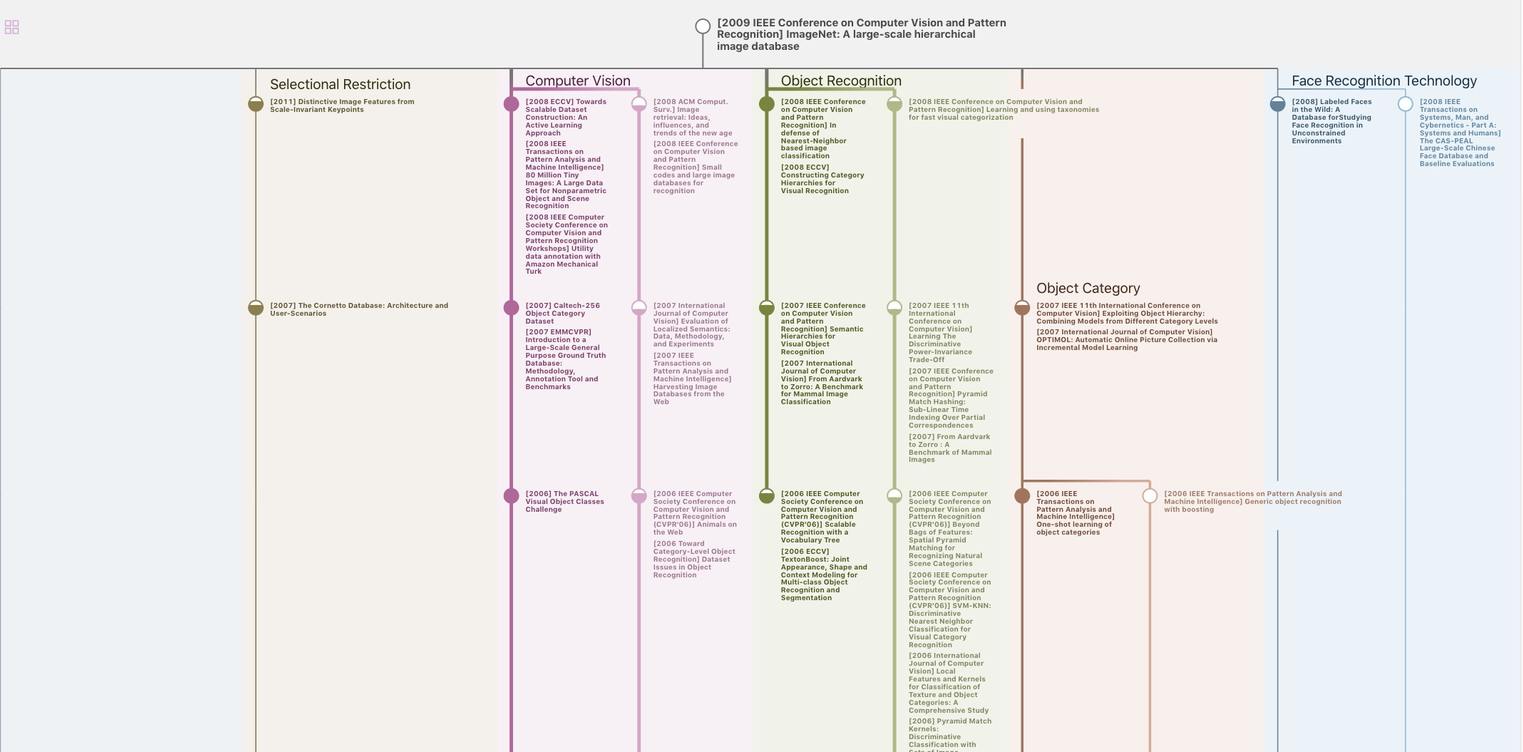
生成溯源树,研究论文发展脉络
Chat Paper
正在生成论文摘要