Enformer: An encoder-decoder generative model to enhance prediction of disease outcomes using electronic health records
Research Square (Research Square)(2023)
摘要
Abstract Deep learning transformer-based models using longitudinal electronic health records (EHRs) have shown a great success in prediction of clinical diseases or outcomes. Pretraining on a large dataset can help such models map the input space better and boost their performance on relevant tasks through finetuning with limited data. In this study, we present Enformer, a generative encoder-decoder model with transformer that was pretrained using a new pretraining objective - predicting all diseases and outcomes of a patient at a future visit from previous visits. Enformer’s encoder-decoder framework, paired with the novel pretraining objective, helped it achieve the new state-of-the-art (SOTA) performance on multiple clinical prediction tasks. Comparing with the previous SOTA model, Enformer improved area under the precision–recall curve (AUPRC) by 2% (p<0.001) for pancreatic cancer onset and by 24% (p<0.001) for intentional self-harm in patients with PTSD. The high performance in predicting intentional self-harm shows the potential of Enformer in building effective clinical intervention systems. Enformer is also generalizable and can be easily finetuned for clinical prediction tasks with limited data.
更多查看译文
关键词
electronic health records,disease outcomes,generative model,encoder-decoder
AI 理解论文
溯源树
样例
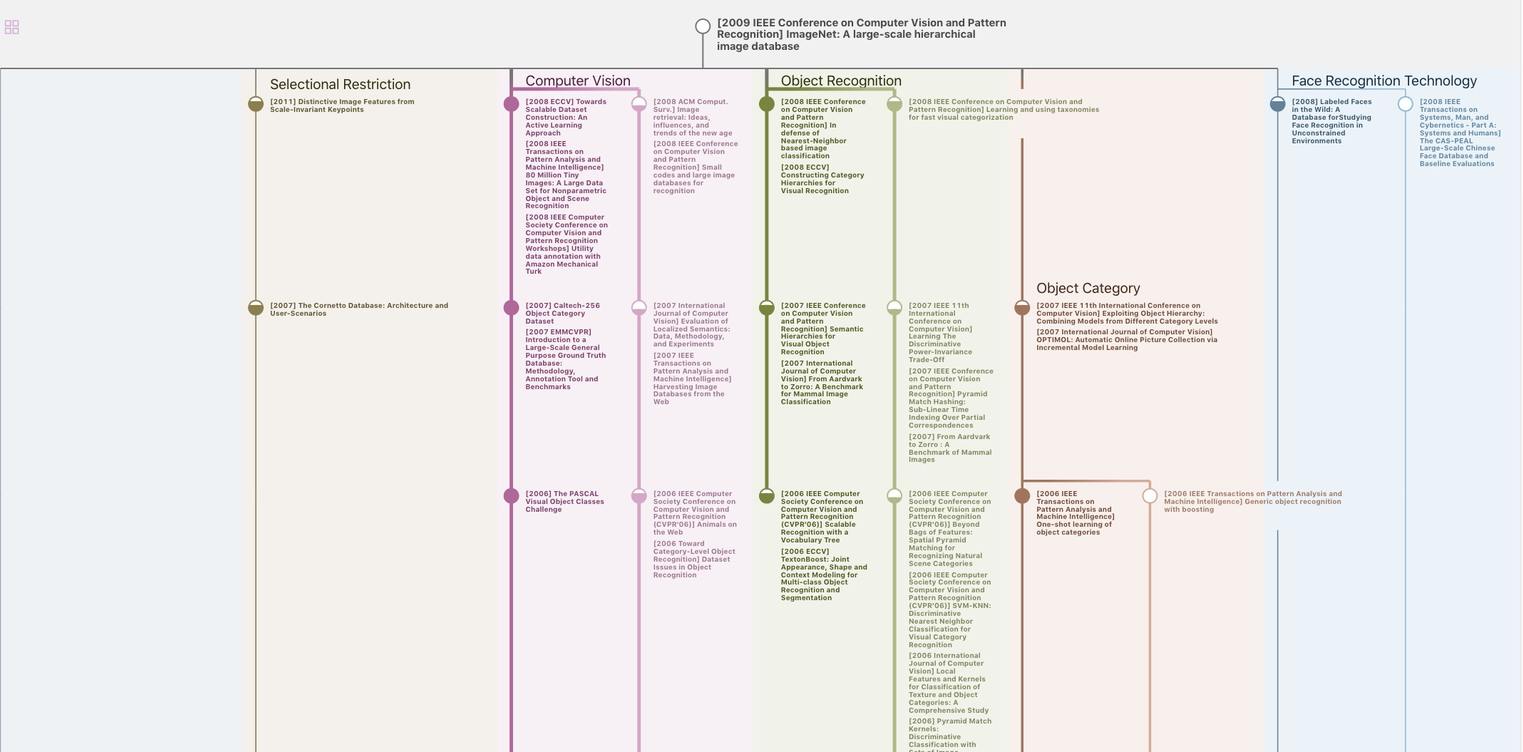
生成溯源树,研究论文发展脉络
Chat Paper
正在生成论文摘要