Large Language Models can be Guided to Evade AI-Generated Text Detection
arXiv (Cornell University)(2023)
摘要
Large language models (LLMs) have shown remarkable performance in various tasks and have been extensively utilized by the public. However, the increasing concerns regarding the misuse of LLMs, such as plagiarism and spamming, have led to the development of multiple detectors, including fine-tuned classifiers and statistical methods. In this study, we equip LLMs with prompts, rather than relying on an external paraphraser, to evaluate the vulnerability of these detectors. We propose a novel Substitution-based In-Context example Optimization method (SICO) to automatically construct prompts for evading the detectors. SICO is cost-efficient as it requires only 40 human-written examples and a limited number of LLM inferences to generate a prompt. Moreover, once a task-specific prompt has been constructed, it can be universally used against a wide range of detectors. Extensive experiments across three real-world tasks demonstrate that SICO significantly outperforms the paraphraser baselines and enables GPT-3.5 to successfully evade six detectors, decreasing their AUC by 0.5 on average. Furthermore, a comprehensive human evaluation as well as a validation experiment in the wild show that the SICO-generated text achieves human-level readability and task completion rates. Finally, the strong performance of SICO exhibits its potential as a reliable evaluation tool for future detectors. The codes and data are located on https://github.com/ColinLu50/Evade-GPT-Detector.
更多查看译文
关键词
large language models,detection,text,ai-generated
AI 理解论文
溯源树
样例
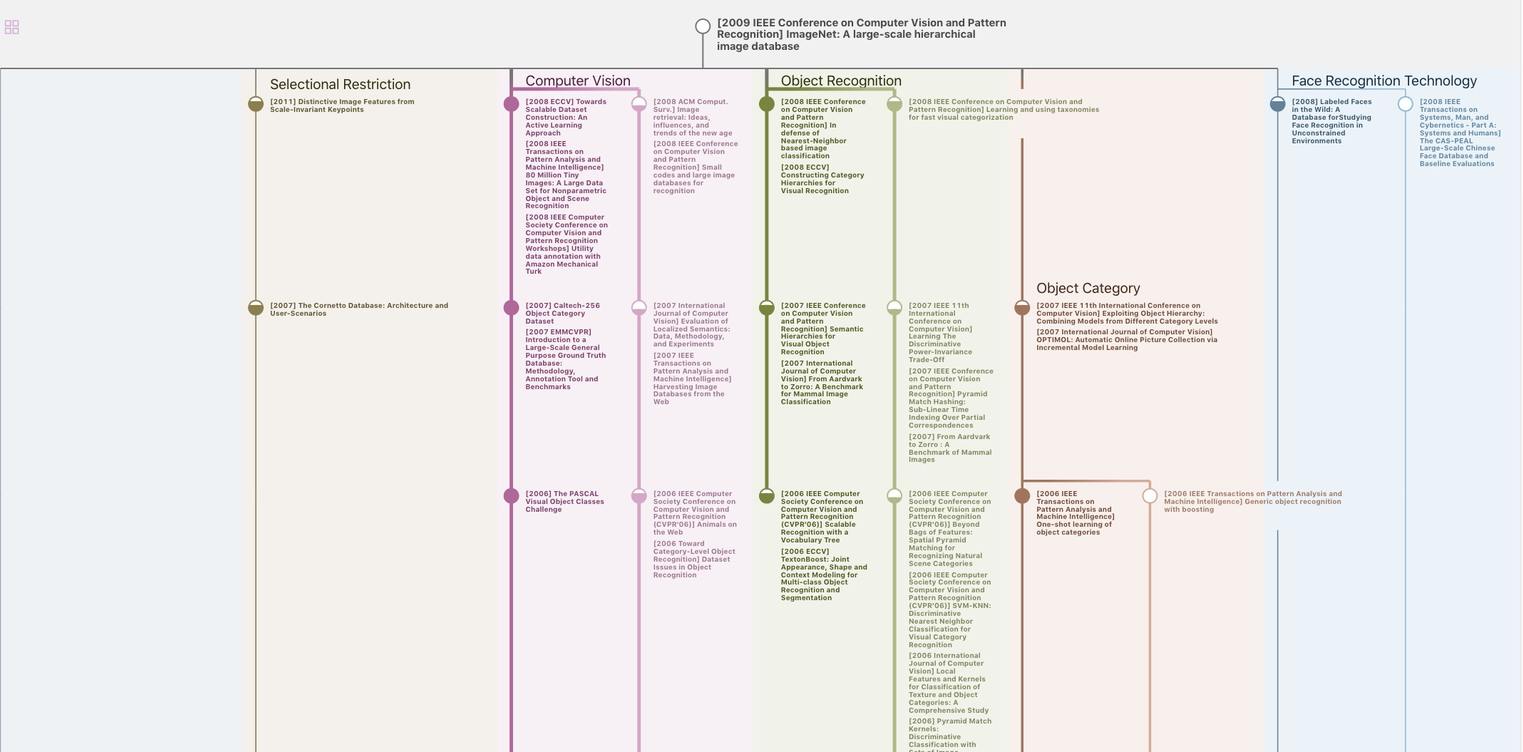
生成溯源树,研究论文发展脉络
Chat Paper
正在生成论文摘要