Sentiment Analysis of Sentence-Level using Dependency Embedding and Pre-trained BERT Model
Penelitian ilmu komputer sistem embedded & logic(2023)
摘要
Sentiment analysis is a valuable field of research in NLP with many applications. Dependency tree is one of the language features that can be utilized in this field. Dependency embedding, as one of the semantic representations of a sentence, has shown to provide more significant results compared to other embeddings, which makes it a potential way to improve the performance of sentiment analysis tasks. This study aimed to investigate the effect of dependency embedding on sentence-level sentiment analysis through experimental research. The study replaced the Vocabulary Graph embedding in the VGCN-BERT sentiment classification system architecture with several dependency embedding representations, including word vector, context vector, average of word and context vectors, weighting on word and context vectors, and merging of word and context vectors. The experiments were conducted on two datasets, SST-2 and CoLA, with more than 19 thousand labeled sentiment sentences. The results indicated that dependency embedding can enhance the performance of sentiment analysis at the sentence level.
更多查看译文
关键词
bert model,dependency embedding,sentence-level,pre-trained
AI 理解论文
溯源树
样例
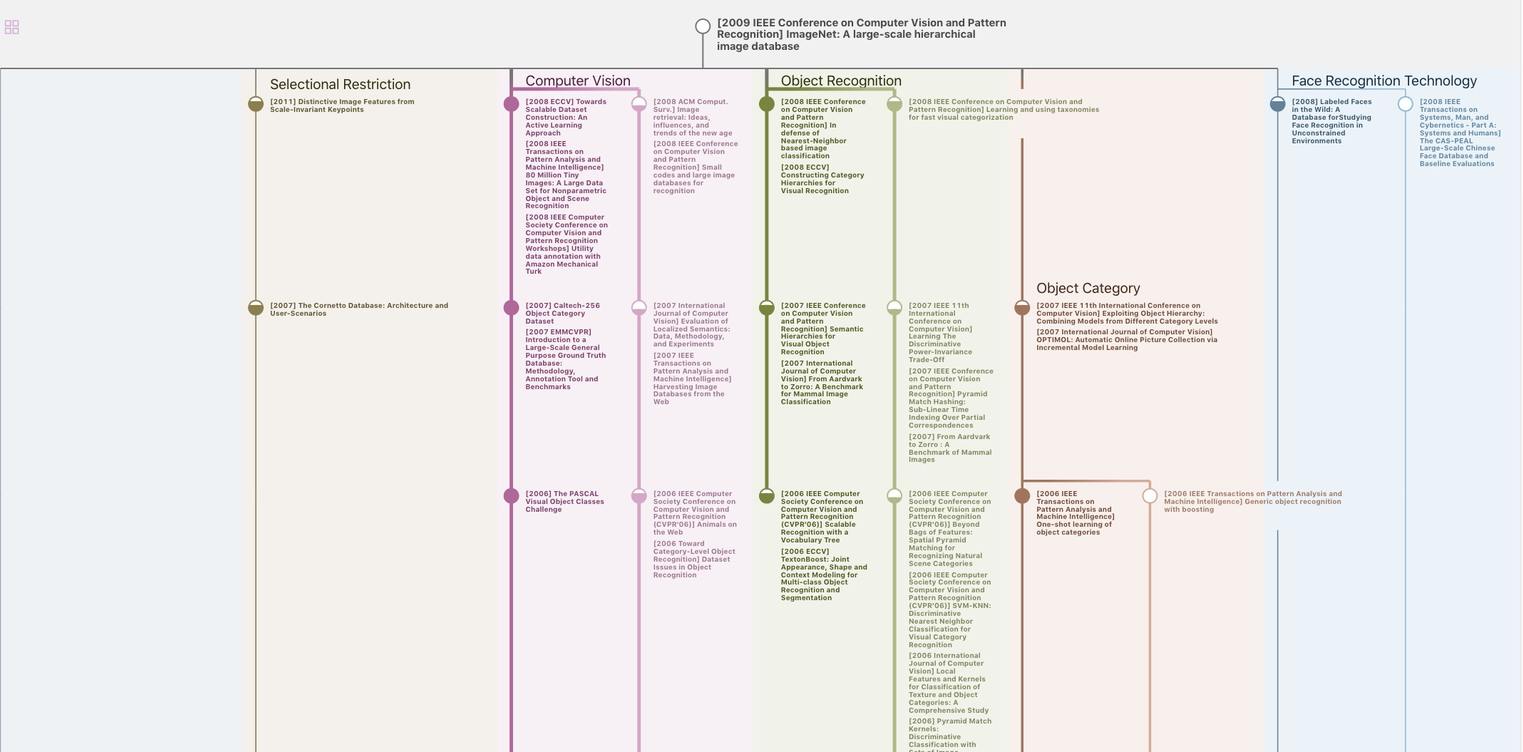
生成溯源树,研究论文发展脉络
Chat Paper
正在生成论文摘要