Unsupervised Machine Learning to Classify the Confinement of Waves in Periodic Superstructures
arXiv (Cornell University)(2023)
摘要
We employ unsupervised machine learning to enhance the accuracy of our recently presented scaling method for wave confinement analysis [1]. We employ the standard k-means++ algorithm as well as our own model-based algorithm. We investigate cluster validity indices as a means to find the correct number of confinement dimensionalities to be used as an input to the clustering algorithms. Subsequently, we analyze the performance of the two clustering algorithms when compared to the direct application of the scaling method without clustering. We find that the clustering approach provides more physically meaningful results, but may struggle with identifying the correct set of confinement dimensionalities. We conclude that the most accurate outcome is obtained by first applying the direct scaling to find the correct set of confinement dimensionalities and subsequently employing clustering to refine the results. Moreover, our model-based algorithm outperforms the standard k-means++ clustering.
更多查看译文
关键词
waves,periodic superstructures,machine learning,confinement
AI 理解论文
溯源树
样例
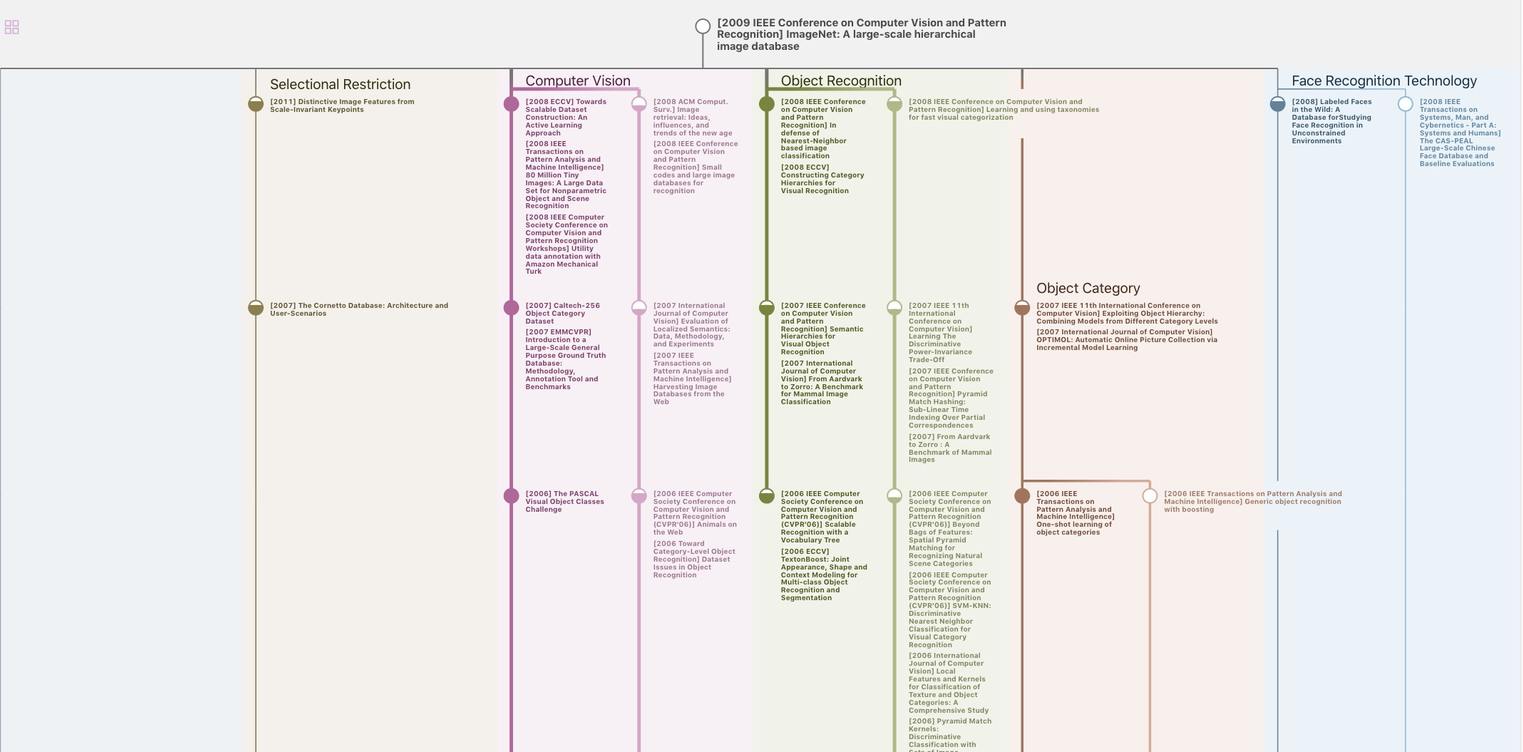
生成溯源树,研究论文发展脉络
Chat Paper
正在生成论文摘要