Data-driven multinomial random forest
arXiv (Cornell University)(2023)
摘要
In this article, we strengthen the proof methods of some previously weakly consistent variants of random forests into strongly consistent proof methods, and improve the data utilization of these variants, in order to obtain better theoretical properties and experimental performance. In addition, based on the multinomial random forest (MRF) and Bernoulli random forest (BRF), we propose a data-driven multinomial random forest (DMRF) algorithm, which has lower complexity than MRF and higher complexity than BRF while satisfying strong consistency. It has better performance in classification and regression problems than previous RF variants that only satisfy weak consistency, and in most cases even surpasses standard random forest. To the best of our knowledge, DMRF is currently the most excellent strongly consistent RF variant with low algorithm complexity
更多查看译文
关键词
forest,data-driven
AI 理解论文
溯源树
样例
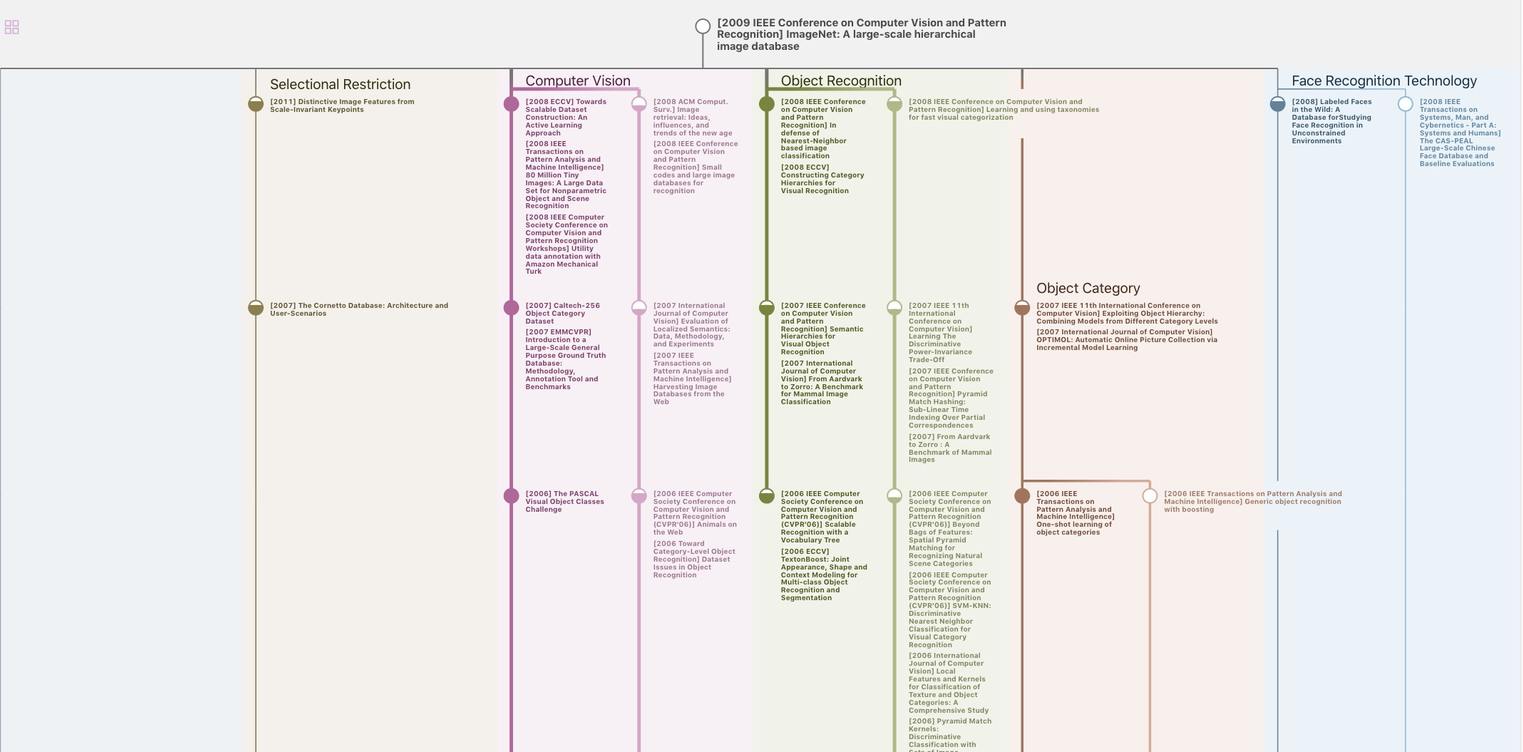
生成溯源树,研究论文发展脉络
Chat Paper
正在生成论文摘要