Probing Conceptual Understanding of Large Visual-Language Models
arXiv (Cornell University)(2023)
摘要
In recent years large visual-language (V+L) models have achieved great success in various downstream tasks. However, it is not well studied whether these models have a conceptual grasp of the visual content. In this work we focus on conceptual understanding of these large V+L models.To facilitate this study, we propose novel benchmarking datasets for probing three different aspects of content understanding, 1) relations, 2) composition and 3) context. Our probes are grounded in cognitive science and help determine if a V+L model can, for example, determine if ``snow garnished with a man'' is implausible, or if it can identify beach furniture by knowing it is located on a beach. We experimented with five different state-of-the-art V+L models and observe that these models mostly fail to demonstrate a conceptual understanding. This study reveals several interesting insights such as cross-attention helps learning conceptual understanding, and that CNNs are better with texture and patterns, while Transformers are better at color and shape. We further utilize some of these insights and propose a baseline for improving performance by a simple finetuning technique that rewards the three conceptual understanding measures with promising initial results. We believe that the proposed benchmarks will help the community assess and improve the conceptual understanding capabilities of large V+L models.
更多查看译文
关键词
visual-language visual-language,conceptual understanding,models
AI 理解论文
溯源树
样例
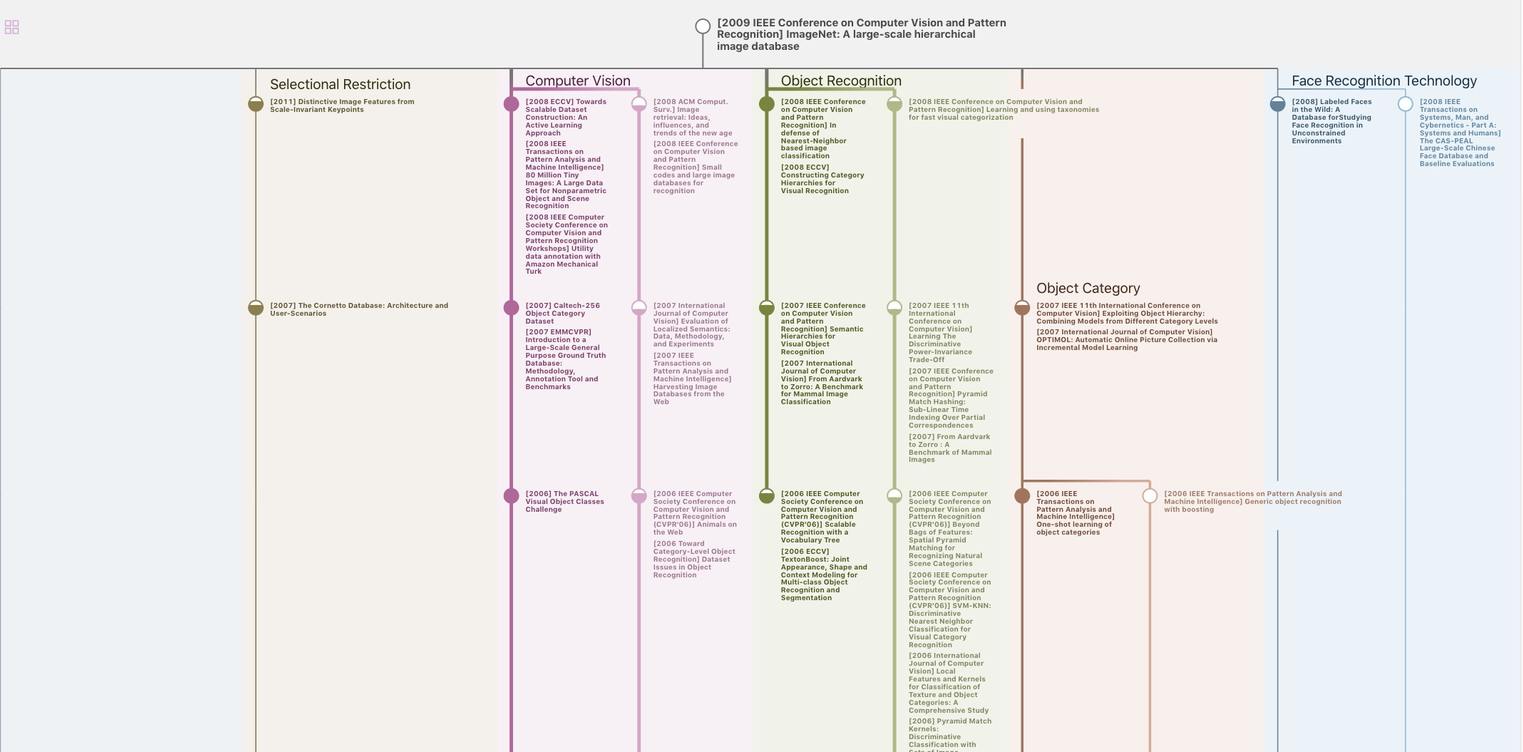
生成溯源树,研究论文发展脉络
Chat Paper
正在生成论文摘要