Centroid-Guided Domain Incremental Learning for EEG-Based Seizure Prediction
IEEE TRANSACTIONS ON INSTRUMENTATION AND MEASUREMENT(2024)
摘要
When building seizure prediction systems, the typical research scenario is patient-specific. In this scenario, the model is limited to performing well for individual patients and cannot acquire knowledge transferable to new patients to learn a set of universal parameters applicable to all patients. To this end, we investigate a new task scenario, domain incremental (DI) learning, which aims to build a unified epilepsy prediction system that performs well across patients by incrementally learning new patients. However, the neural network exhibits catastrophic forgetting (CF) due to abrupt shifts in domain distributions. The learned representations drift drastically during incremental training, which quickly forgets the knowledge learned from past tasks. To address this, we introduce the experience replay (ER) method, which stores a few samples from previous patients and then replays them in new patient training to facilitate episodic memory formation and consolidation. In addition, we propose a novel centroid-guided ER method (CGER) that computes the class centroid in the feature space using subsets stored in the memory buffer to provide semantic memory. The CGER regularizes incremental training using cosine similarity to measure the distance between sample embeddings and class centroids, providing additional guidance for parameter updates. Experimental results demonstrate that the ER approach substantially reduces CF and significantly improves performance when combined with CG.
更多查看译文
关键词
Catastrophic forgetting (CF),domain incremental (DI) learning,electroencephalogram (EEG),experience replay (ER),seizure prediction
AI 理解论文
溯源树
样例
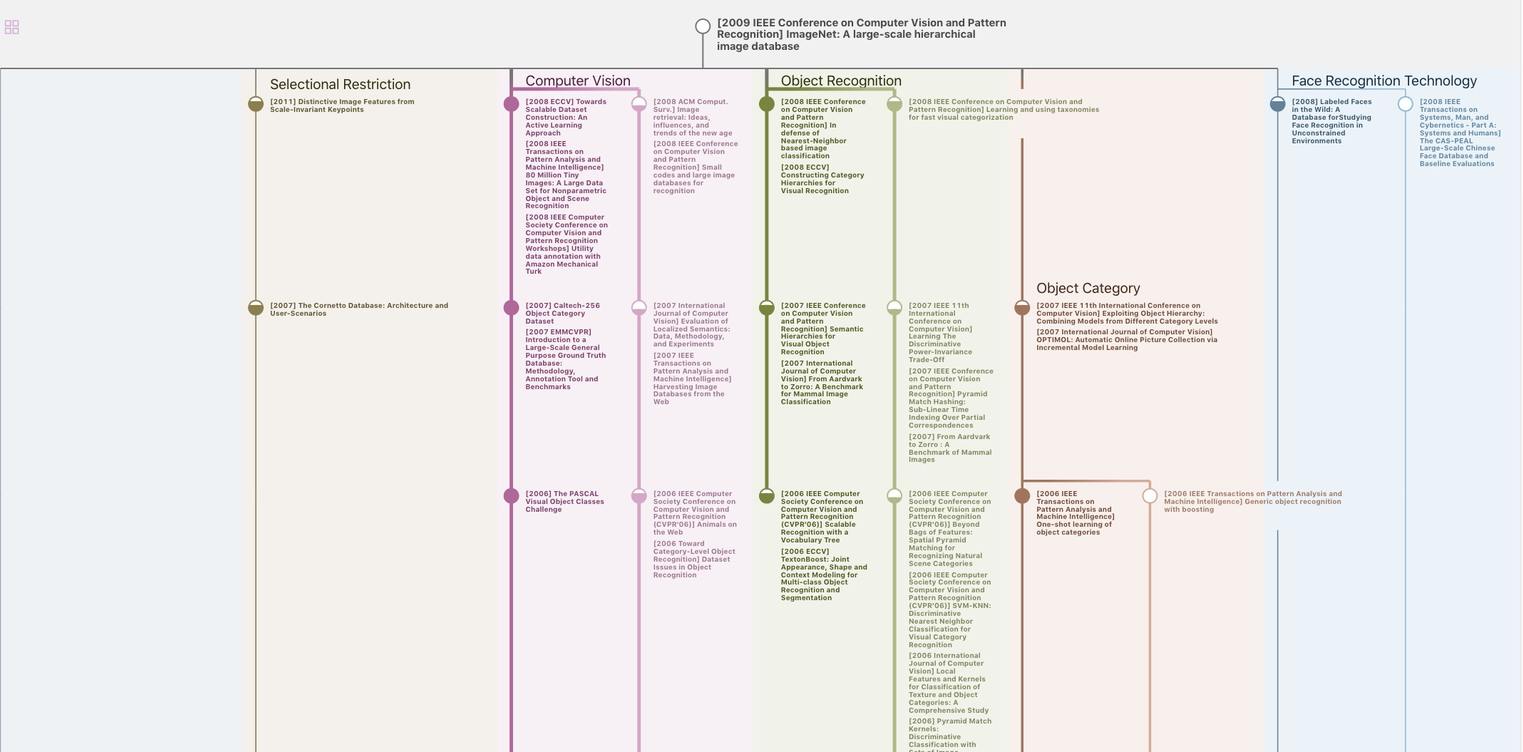
生成溯源树,研究论文发展脉络
Chat Paper
正在生成论文摘要