A Variational Auto-Encoder-Based Multisource Deep Domain Adaptation Model Using Optimal Transport for Cross-Machine Fault Diagnosis of Rotating Machinery
IEEE TRANSACTIONS ON INSTRUMENTATION AND MEASUREMENT(2024)
Abstract
In recent years, most existing domain-adapted bearing fault diagnoses for rotating machinery have been designed to decrease domain drifts for various operating conditions with an assumption that sufficient tag data are available. To overcome data scarcity, a possible solution is to use fault information of other machines of the same category to diagnose the status of a target machine (i.e., cross-machine diagnosis). This article proposes a variational auto-encoder (VAE)-based multisource deep domain adaptation model using optimal transport for cross-machine fault diagnosis of rotating machinery (named MDVAEOT). This is fundamentally different from most diagnostic models where both train and test data belong to the same machine. First, it uses unlabeled samples of the machines to be diagnosed to establish the target dataset and faulty samples of machines of the same category (containing labels) to form the source dataset. Additionally, the method performs feature extraction on the dataset using VAE networks and improves the reliability of extracted data features by the approximation of fixed probability. Finally, to shrink cross-machine differences between the two domains, we introduce optimal transport (OT) theory. OT distance is used to share fault-related features between the two domains mentioned above to complete the cross-machine diagnosis task. Better accuracy and timeliness are offered by this proposed means compared to other existing intelligent methods in this field.
MoreTranslated text
Key words
Cross-machine,domain adaptation,fault diagnosis,optimal transport (OT),rotating machinery,variational auto-encoder (VAE)
AI Read Science
Must-Reading Tree
Example
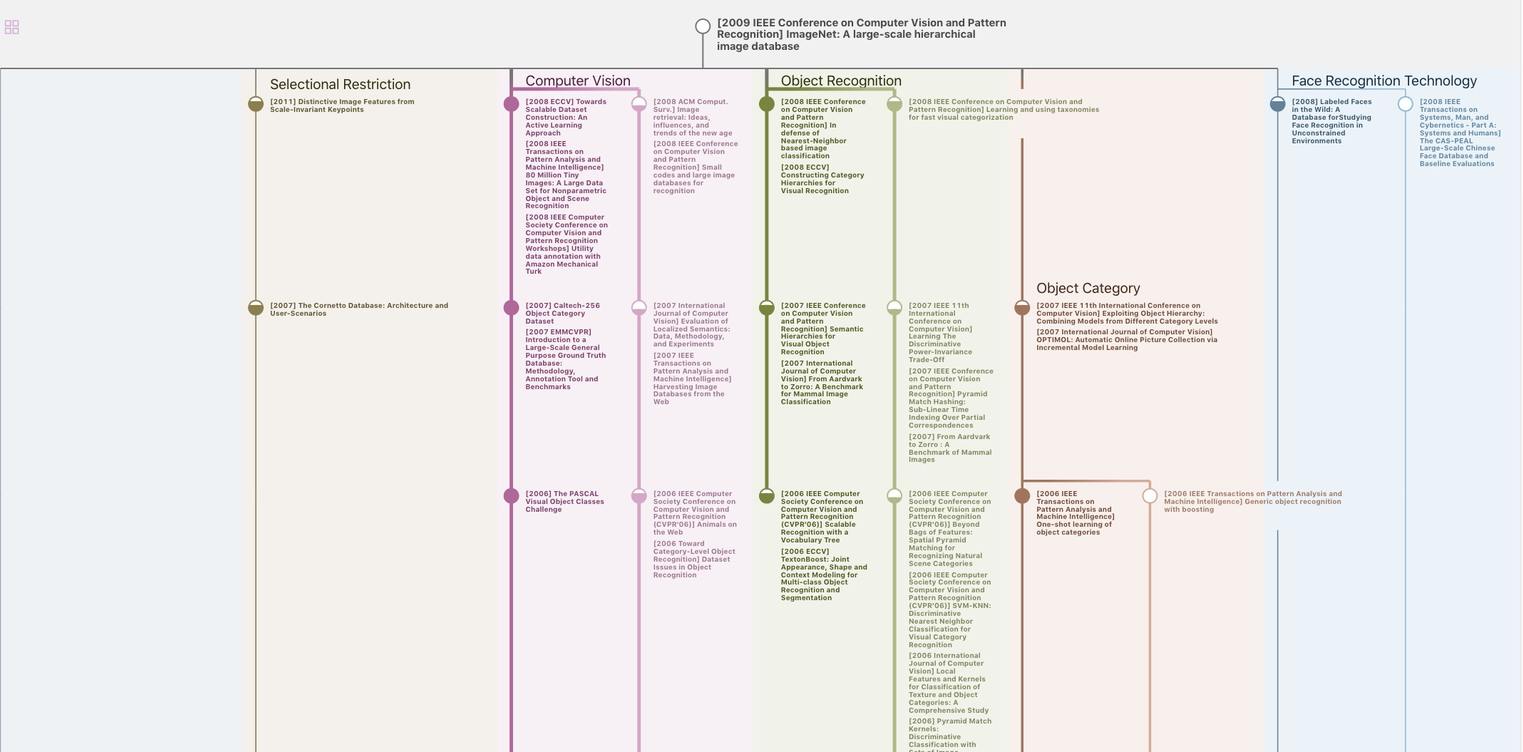
Generate MRT to find the research sequence of this paper
Chat Paper
Summary is being generated by the instructions you defined