Mapping Deprived Urban Areas Using Open Geospatial Data and Machine Learning in Africa
URBAN SCIENCE(2023)
摘要
Reliable data on slums or deprived living conditions remain scarce in many low- and middle-income countries (LMICs). Global high-resolution maps of deprived areas are fundamental for both research- and evidence-based policies. Existing mapping methods are generally one-off studies that use proprietary commercial data or other physical or socio-economic data that are limited geographically. Open geospatial data are increasingly available for large areas; however, their unstructured nature has hindered their use in extracting useful insights to inform decision making. In this study, we demonstrate an approach to map deprived areas within and across cities using open-source geospatial data. The study tests this methodology in three African cities-Accra (Ghana), Lagos (Nigeria), and Nairobi (Kenya) using a three arc second spatial resolution. Using three machine learning classifiers, (i) models were trained and tested on individual cities to assess the scalability for large area application, (ii) city-to-city comparisons were made to assess how the models performed in new locations, and (iii) a generalized model to assess our ability to map across cities with training samples from each city was designed. Our best models achieved over 80% accuracy in all cities. The study demonstrates an inexpensive, scalable, and transferable approach to map deprived areas that outperforms existing large area methods.
更多查看译文
关键词
open geospatial,GIS,Africa,slums,deprived area,machine learning,remote sensing,OpenStreetMap,poverty mapping,informal settlements,LMIC
AI 理解论文
溯源树
样例
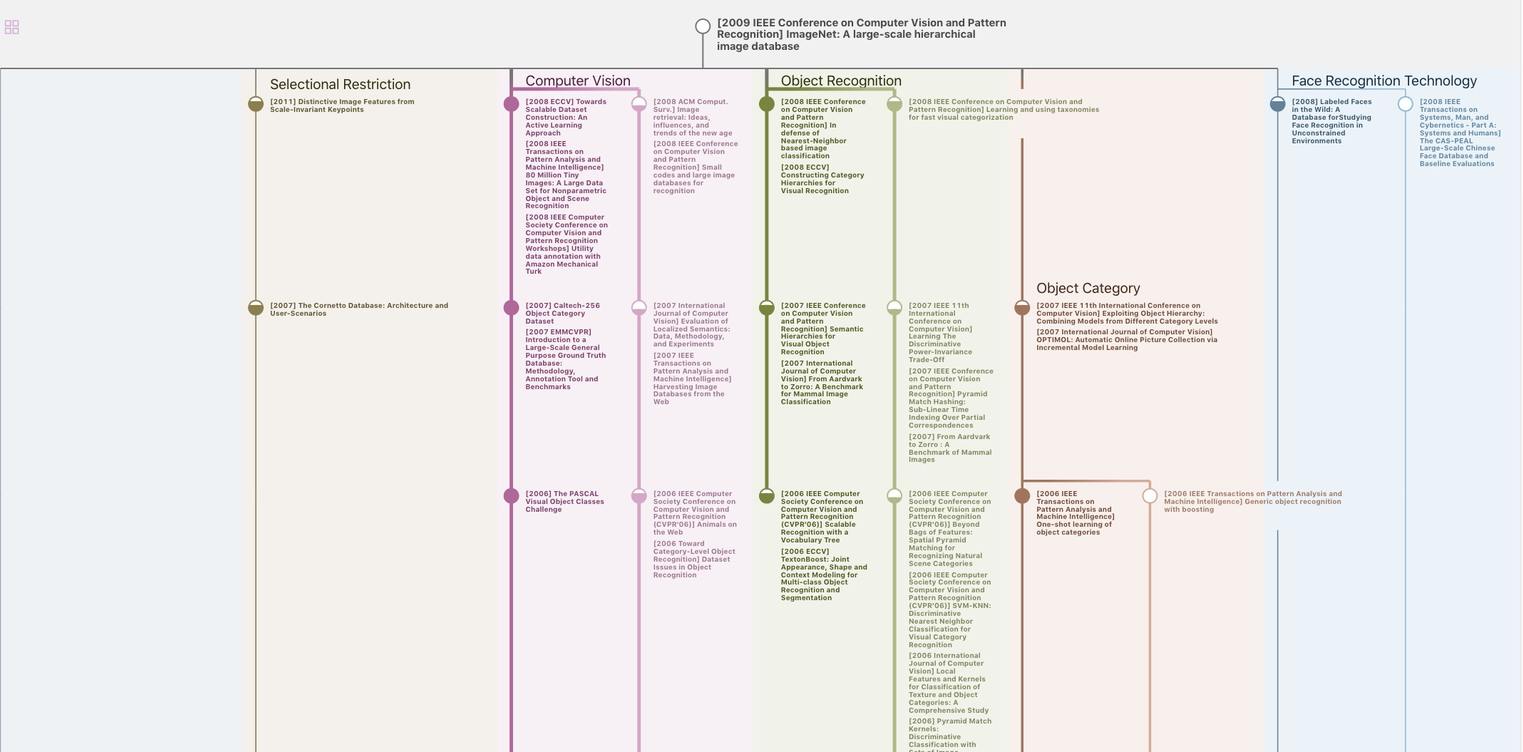
生成溯源树,研究论文发展脉络
Chat Paper
正在生成论文摘要