Online Training Flow Scheduling for Geo-Distributed Machine Learning Jobs Over Heterogeneous and Dynamic Networks.
IEEE Trans. Cogn. Commun. Netw.(2024)
摘要
Geo-Distributed Machine Leaning (Geo-DML) has been a promising technology, which performs collaborative learning across geographically dispersed data centers (DCs) with privacy-preserving over Wide Area Networks (WANs). Unfortunately, the limited and heterogeneous WAN bandwidth poses significant challenges to the performance of Geo-DML systems, leading to increased communication overhead and affecting the revenue of ISPs eventually. In particular, when multiple online jobs coexist in Geo-DML systems, the competition for bandwidth between training flows of different jobs aggravates this negative impact. To alleviate it, this paper investigates the problem of online training flow scheduling for Geo-DML jobs. We first formulate the studied problem as an Linear Programming (LP) model with the objective of maximizing the revenue of ISPs. Then, we propose an online traffic scheduling algorithm called Training Flow Adaptive Steering (TFAS), which exploits a primal-dual framework, tailored for efficient resource allocation of jobs to schedule training flows, such that system resources are maximally utilized and training procedures can be expedited and completed in a timely manner. Meanwhile, we conduct rigorous theoretical analysis to guarantee that the proposed algorithm can achieve a good competitive ratio. Extensive evaluation results demonstrate that our algorithm performs well and outperforms commonly adopted solutions 36.2%-49.4% in average.
更多查看译文
关键词
Geo-Distributed Machine Leaning,Training Jobs,Resource Allocation,Online Scheduling
AI 理解论文
溯源树
样例
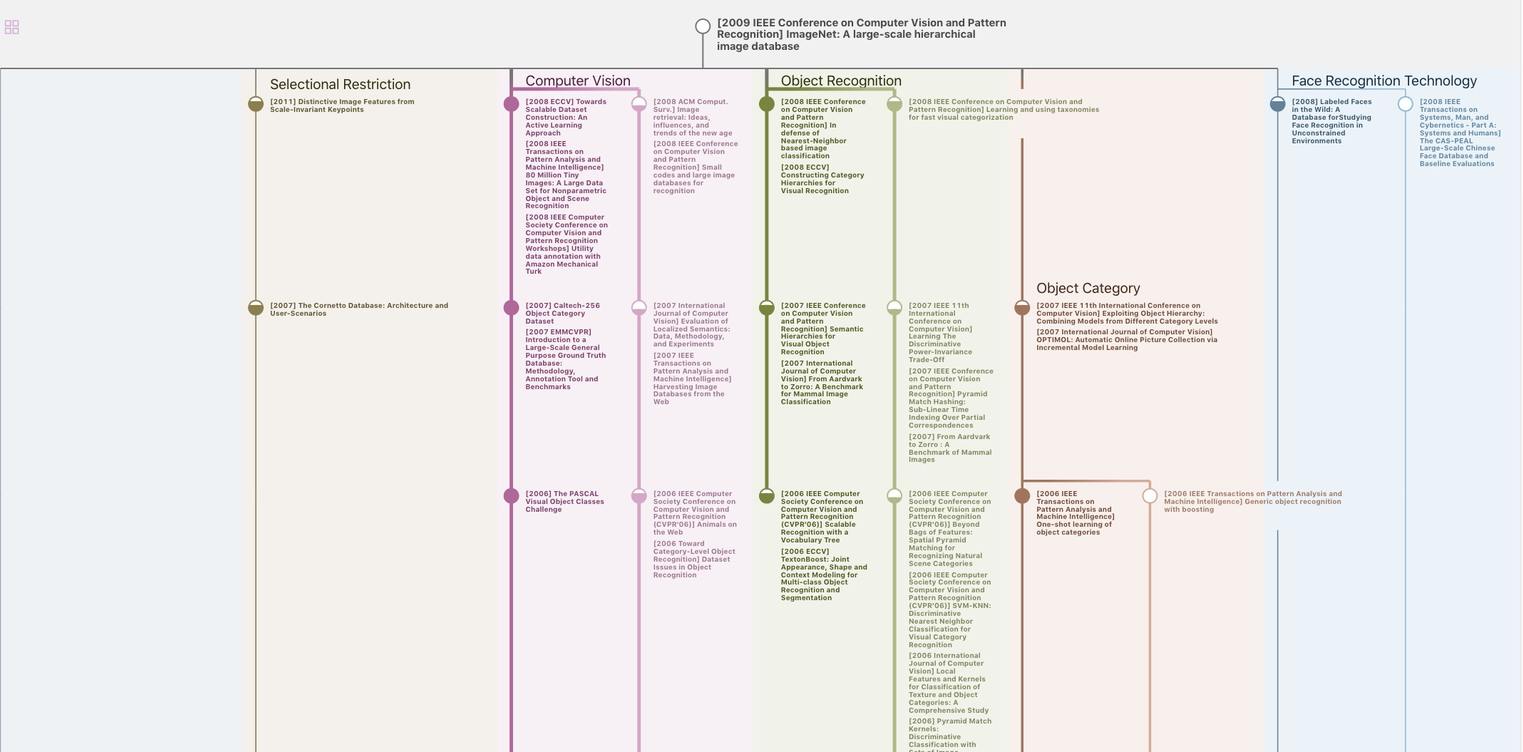
生成溯源树,研究论文发展脉络
Chat Paper
正在生成论文摘要