Spatial-Attention-Based Channel Estimation in IRS-Assisted mmWave MU-MISO Systems
IEEE INTERNET OF THINGS JOURNAL(2024)
摘要
Intelligent reflecting surface (IRS)-assisted communication systems rely heavily on the precise estimation of channel state information (CSI) for their effective operation. However, obtaining the accurate CSI is challenging due to the substantial pilot overhead required in such systems. In this article, we propose a spatial attention (SA)-based method to estimate the cascaded downlink channel in an IRS-assisted millimeter-wave (mmWave) multiuser multiple input single output (MU-MISO) system that operates with the frequency division duplex (FDD) protocol. In order to estimate the channel information, we formulate it as a nonlinear prediction problem and propose a residual network-based channel estimation (RN-CE) framework that reduces the pilot overhead. To implement the RN-CE, we utilize the values of the least square (LS) estimators as coarse estimates for neural network training. Additionally, we develop a spatial attention-based RN-CE (SA-RN-CE) that integrates a lightweight SA module capable of extracting internal connections among spatial features. Simulation results demonstrate that both RN-CE and SA-RN-CE outperform the LS algorithm, minimum mean squared error (MMSE) algorithm, and convolutional neural network (CNN)-based ChannelNet algorithm in terms of normalized mean square error (NMSE). It has been found that by balancing the NMSE and pilot overhead, the length of the pilot signals used for training the networks in RN-CE and SA-RN-CE can be compressed to 1/8 of its original length, while still maintaining a minimal impact on NMSE, which is particularly advantageous in cascaded channels where pilot training overhead is typically extensive.
更多查看译文
关键词
Channel estimation,Training,Millimeter wave communication,Feature extraction,Estimation,Reflection,Downlink,deep learning,intelligent reflecting surface (IRS),residual neural network,spatial attention (SA)
AI 理解论文
溯源树
样例
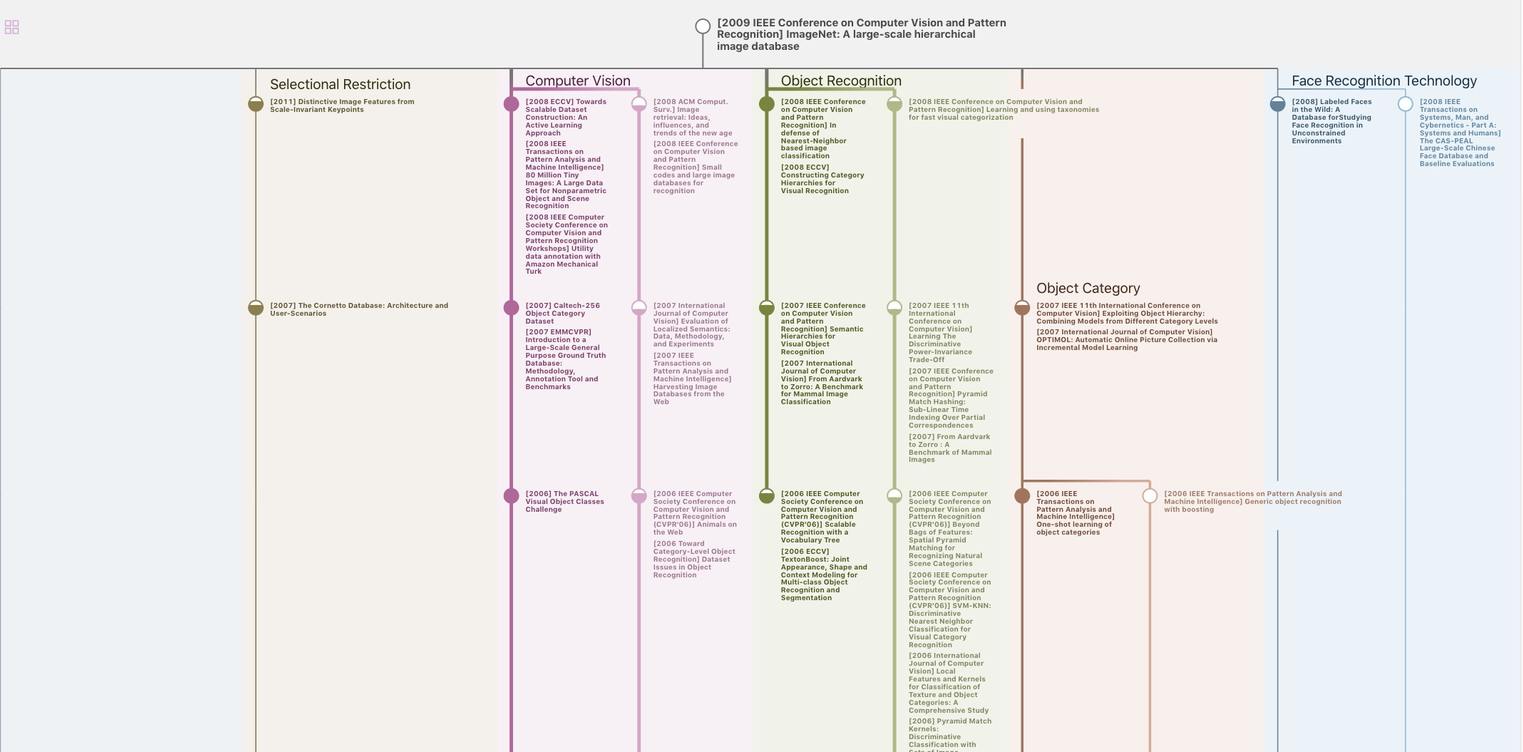
生成溯源树,研究论文发展脉络
Chat Paper
正在生成论文摘要