MEGAN: Multi-explanation Graph Attention Network
Communications in computer and information science(2023)
摘要
We propose a multi-explanation graph attention network (MEGAN). Unlike existing graph explainability methods, our network can produce node and edge attributional explanations along multiple channels, the number of which is independent of task specifications. This proves crucial to improve the interpretability of graph regression predictions, as explanations can be split into positive and negative evidence w.r.t to a reference value. Additionally, our attention-based network is fully differentiable and explanations can actively be trained in an explanation-supervised manner. We first validate our model on a synthetic graph regression dataset with known ground-truth explanations. Our network outperforms existing baseline explainability methods for the single- as well as the multi-explanation case, achieving near-perfect explanation accuracy during explanation supervision. Finally, we demonstrate our model’s capabilities on multiple real-world datasets. We find that our model produces sparse high-fidelity explanations consistent with human intuition about those tasks.
更多查看译文
关键词
attention,graph,network,multi-explanation
AI 理解论文
溯源树
样例
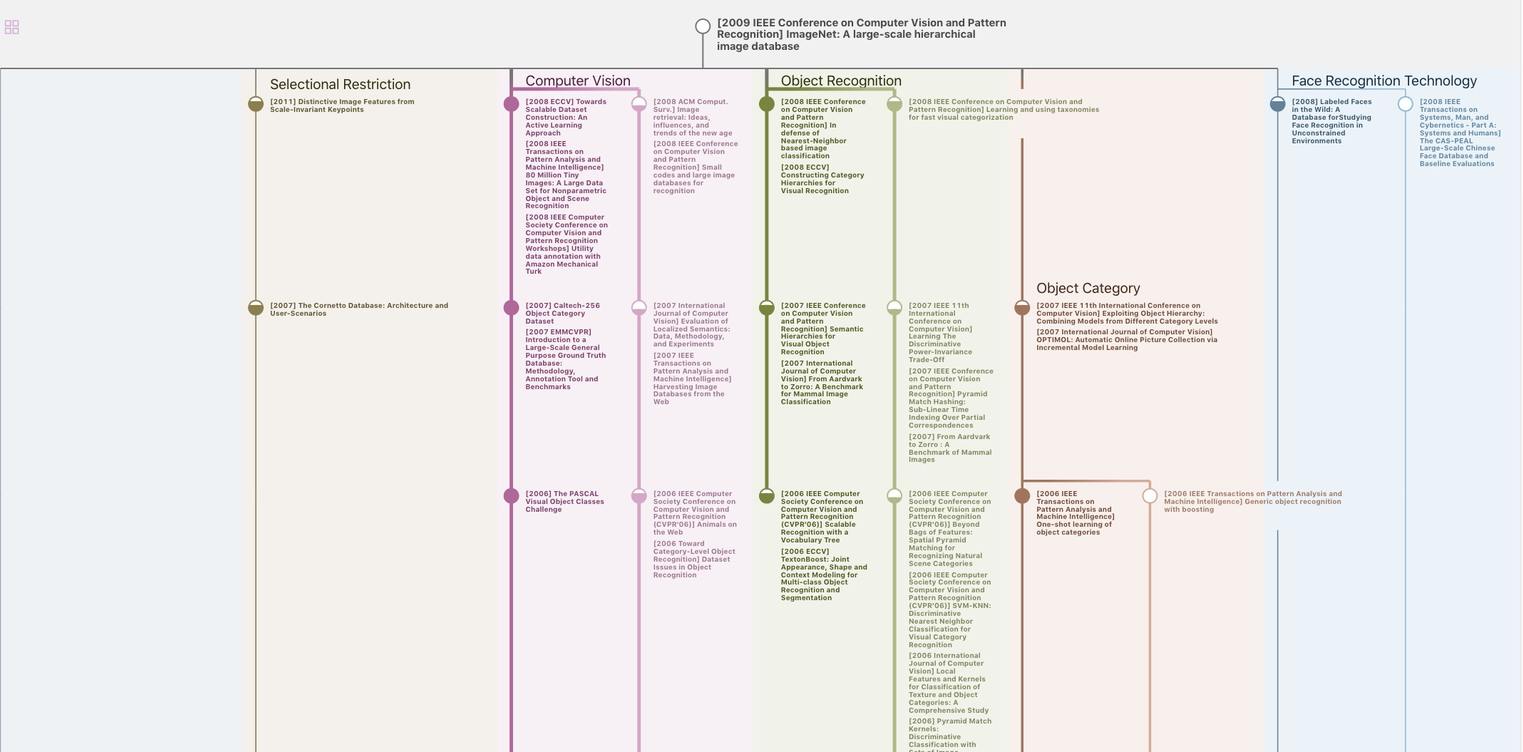
生成溯源树,研究论文发展脉络
Chat Paper
正在生成论文摘要