Novel Deep Layers-Extended Autoencoder With Correlation and Its Industrial Soft Sensing
IEEE TRANSACTIONS ON INDUSTRIAL INFORMATICS(2024)
摘要
Due to the increasing complexity of industrial processes, accurately constructing soft sensors has become a daunting challenge. Recently, stacked autoencoder (SAE) has been utilized to develop data-driven soft sensors due to their superior capability in feature extraction. However, the conventional SAE lacks correlation in feature extraction for each autoencoder (AE) as it is independent. In addition, the training process of AE is unsupervised, ignoring the correlation between input and output data. To address these issues, a novel SAE with a variant structure called deep layers-extended autoencoder (DLEAE) is proposed. In the DLEAE, an extended layer is introduced, and the original inputs and the information of the hidden layers of previous LEAE units are added to the follow-up LEAE unit. This extends the width of DLEAE and strengthens the utilization of the features extracted from preceding layers. Furthermore, the expected outputs are introduced into the feature extraction process of each LEAE unit, which filters out output-irrelevant information. Finally, experimental verifications using two actual complex industrial processes are performed to validate the effectiveness and superiority of the proposed DLEAE.
更多查看译文
关键词
Deep learning (DL),industrial processes,industrial soft sensor,layers-extended autoencoder (LEAE)
AI 理解论文
溯源树
样例
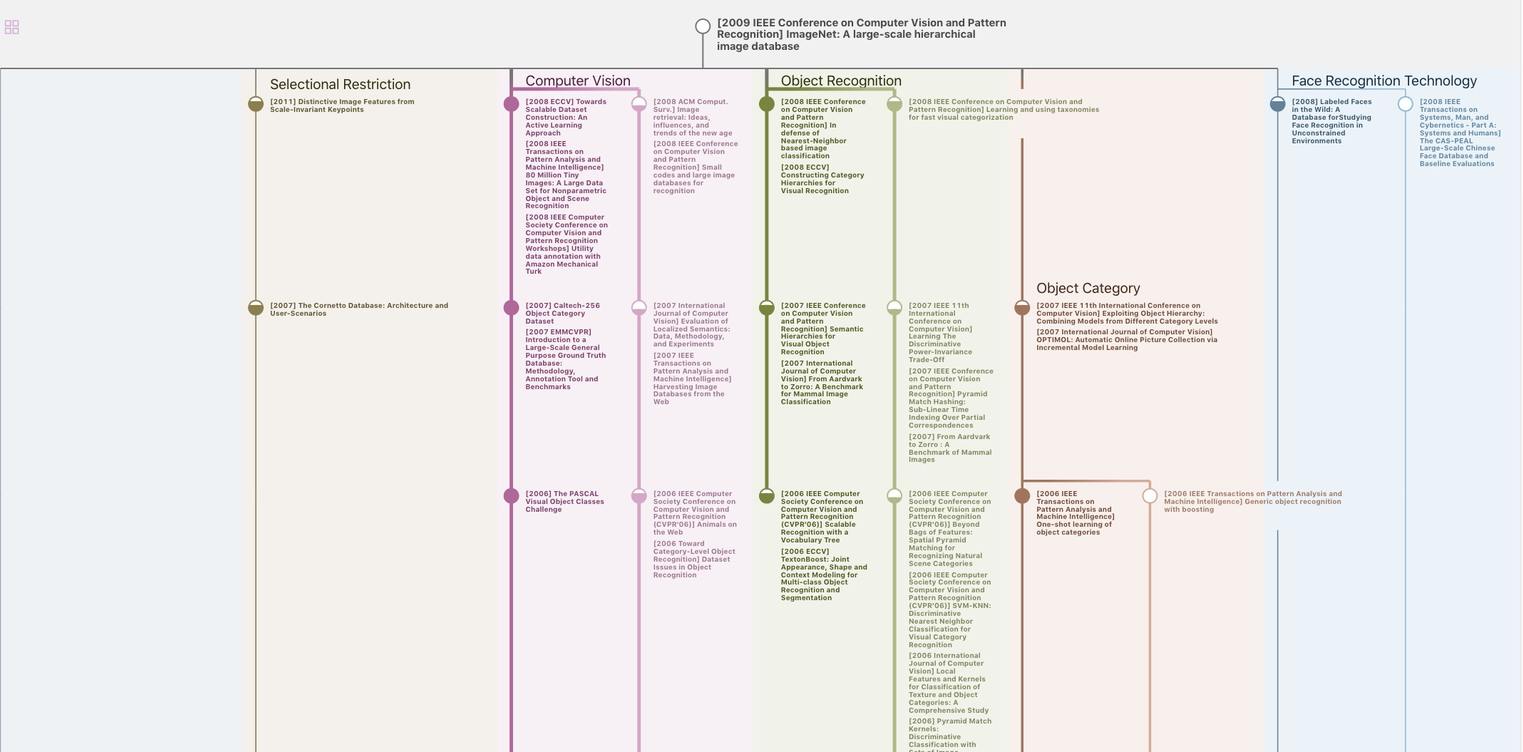
生成溯源树,研究论文发展脉络
Chat Paper
正在生成论文摘要