Improving Gene Regulatory Network Inference using Dropout Augmentation
bioRxiv (Cold Spring Harbor Laboratory)(2023)
摘要
Abstract Motivation Many methods have been proposed to infer gene regulatory networks (GRNs) from single-cell RNA sequencing (scRNA-seq) data. One major challenge when working with single-cell data is the prevalence of dropout events, when some expression values are not captured. Here we propose to improve GRN inference using Dropout Augmentation (DA), which helps models stay robust against stochastic dropout events by introducing more dropout noise during training. Results Benchmarking experiments illustrate the improved performance and increased stability of the proposed DAZZLE model over existing approaches. Exploration of the source of gain shows that DA plays a significant role in improving performance, while restricting the sparsity of the adjacency matrix at a later stage stabilizes the model. The practical application of the DAZZLE model on a longitudinal mouse microglia dataset, containing over 15,000 genes, illustrates its ability to handle real-world single cell data with minimal gene filtration. Conclusions The improved robustness and stability of DAZZLE make it a practical and valuable addition to the toolkit for GRN inference from single-cell data. Dropout Augmentation may have wider applications beyond the GRN-inference problem. Availability and implementation Project website: https://bcb.cs.tufts.edu/DAZZLE ; Visualization of inferred mouse microglia network: https://bcb.cs.tufts.edu/DAZZLE/hammond.html ; Code available at https://github.com/TuftsBCB/dazzle and on PyPI under the grn-dazzle package. Contact hao.zhu@tufts.edu ; donna.slonim@tufts.edu
更多查看译文
关键词
sem model,gene,inference,grn-vae
AI 理解论文
溯源树
样例
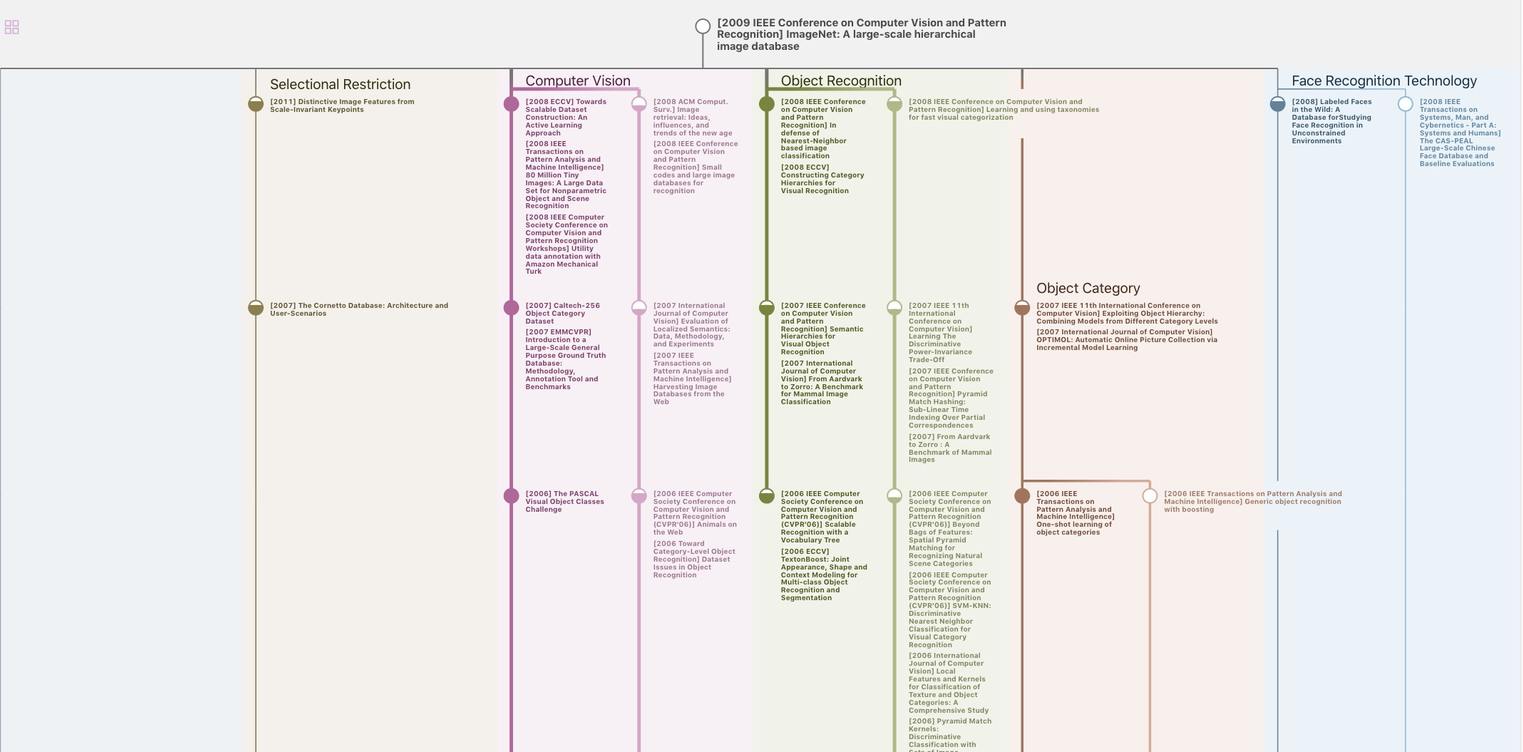
生成溯源树,研究论文发展脉络
Chat Paper
正在生成论文摘要