Attention-Based Deep Reinforcement Learning for Edge User Allocation
IEEE TRANSACTIONS ON NETWORK AND SERVICE MANAGEMENT(2024)
Abstract
Edge computing, a recently developed computing paradigm, seeks to extend cloud computing by providing users minimal latency. In a mobile edge computing (MEC) environment, edge servers are placed close to edge users to offer computing resources, and the coverage of adjacent edge servers may partially overlap. Because of the restricted resource and coverage of each edge server, edge user allocation (EUA), i.e., determining the optimal way to allocate users to different servers in the overlapping area, has emerged as a major challenge in edge computing. Despite the NP-hardness of obtaining an optimal solution, it is possible to evaluate the quality of a solution in a short amount of time with given metrics. Consequently, deep reinforcement learning (DRL) can be used to solve EUA by attempting numerous allocations and optimizing the allocation strategy depending on the rewards of those allocations. In this study, we propose the Dual-sequence Attention Model (DSAM) as the DRL agent, which encodes users using self-attention mechanisms and directly outputs the probability of matching between users and servers using an attention-based pointer mechanism, enabling the selection of the most suitable server for each user. Experimental results show that our method outperforms the baseline approaches in terms of allocated users, required servers, and resource utilization, and its running speed meets real-time requirements.
MoreTranslated text
Key words
Edge user allocation,deep reinforcement learning,edge computing
AI Read Science
Must-Reading Tree
Example
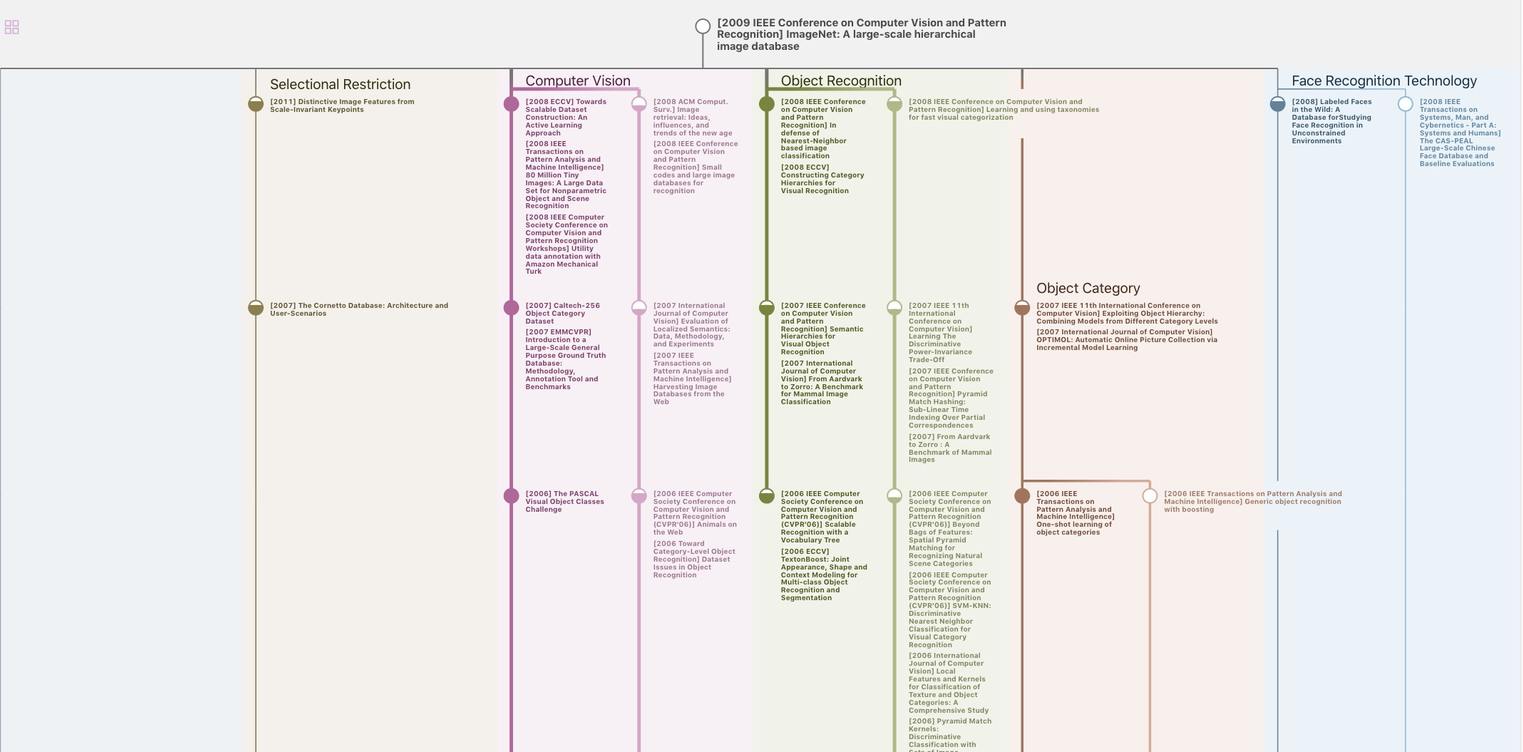
Generate MRT to find the research sequence of this paper
Chat Paper
Summary is being generated by the instructions you defined