Predicting and monitoring blood glucose through nutritional factors in type 1 diabetes by artificial neural networks
Acta IMEKO(2023)
摘要
The monitoring and management of Postprandial Glucose Response (PGR), by administering an insulin bolus before meals, is a crucial issue in Type 1 Diabetes (T1D) patients. Artificial Pancreas (AP), which combines autonomous insulin delivery and blood glucose sensor, is a promising solution; nevertheless, it still requires input from patients about meal carbohydrate intake for bolus administration. This is due to the limited knowledge of the factors that influence PGR. Even though meal carbohydrates are regarded as the major factor influencing PGR, medical experience suggests that other nutritional should be considered. To address this issue, in this work, we propose a Machine Learning (ML)-based approach for a more comprehensive analysis of the impact of nutritional factors (i.e., carbohydrates, protein, lipids, fiber, and energy intake) on the blood glucose levels (BGLs). In particular, the proposed ML-model takes into account BGLs, insulin doses, and nutritional factors in T1D patients to predict BGLs in 60-minute time windows after a meal. A Feed-Forward Neural Network was fed with different combinations of BGLs, insulin, and nutritional factors, providing a predicted glycaemia curve as output. The validity of the proposed system was demonstrated through tests on public data and on self-produced data, adopting intra- and inter-subject approach. Results anticipate that patient-specific data about nutritional factors of a meal have a major role in the prediction of postprandial BGLs.
更多查看译文
关键词
blood glucose,diabetes,artificial neural networks,nutritional factors
AI 理解论文
溯源树
样例
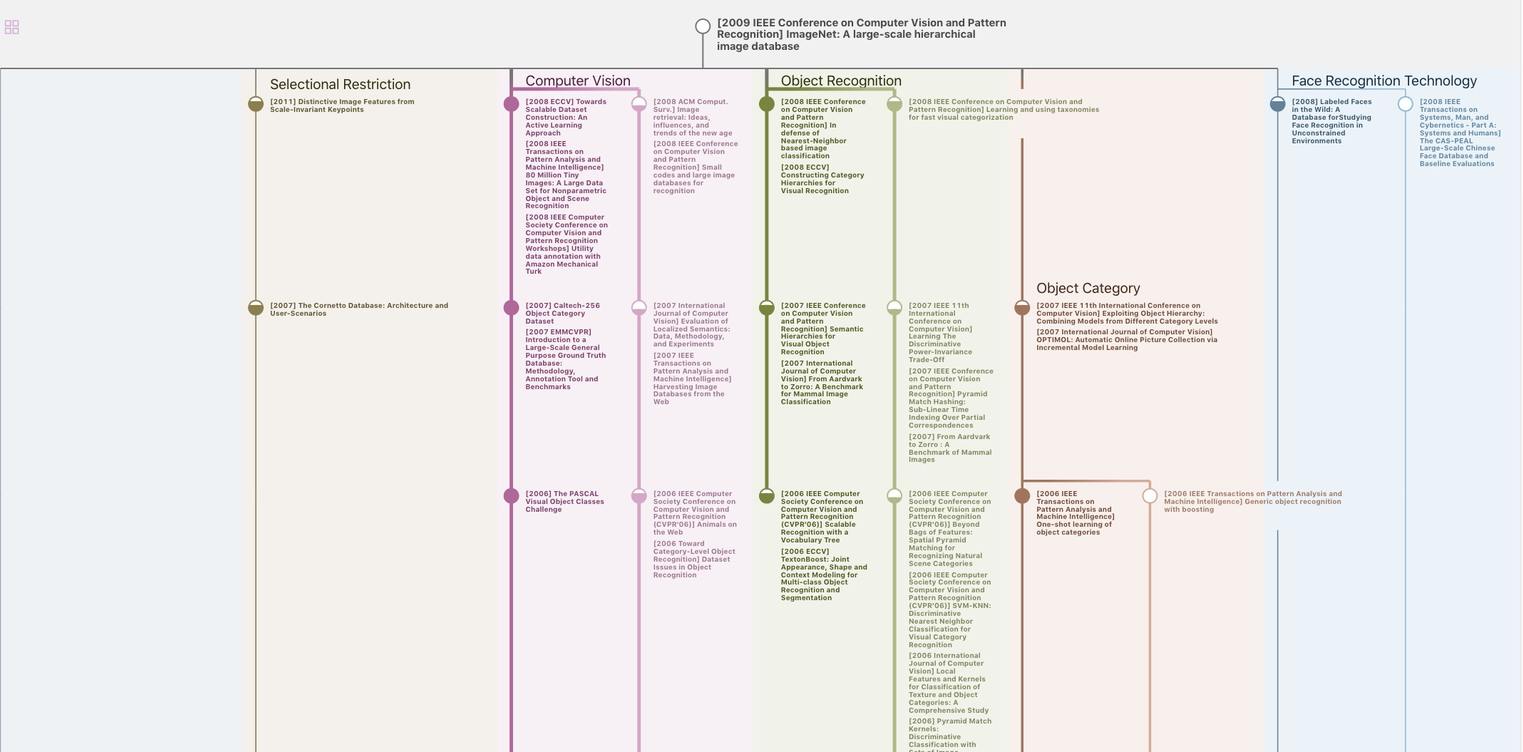
生成溯源树,研究论文发展脉络
Chat Paper
正在生成论文摘要