An Explainable Coarse-to-Fine Survival Analysis Method on Multi-Center Whole Slide Images.
IEEE Trans. Artif. Intell.(2024)
摘要
Survival models based on whole slide images (WSIs) are widely used in precision medicine to treat cancer patients better. Most previous studies attempted to address the challenge of WSIs' gigapixel resolutions to survival models, but they failed in terms of the computational efficiency and interpretability of models. This study proposes a coarse-to-fine survival model called WSISur based on graph neural networks, which not only solves the above two problems but also achieves the best survival prediction performance. To solve the issue of computational efficiency, coarse WSI graphs are first constructed on low-resolution images in WSIs, and then fine WSI graphs are built with high-resolution images on the basis of coarse WSI graphs. Subsequent survival analysis is performed on the constructed WSI graphs. To solve the issue of interpretability of the model, WSIs' regions most relevant to patients' lifetimes are identified by gradient-weighted class activation mapping. Nevertheless, due to the imbalance of data labels, there is a problem of probability failure in end-to-end mini-batch training. To this end, survival event sampling strategy is proposed to balance data labels. In addition, a series of experiments are carried out on multi-center datasets to evaluate the proposed model. Experimental results show that WSISur achieves the state-of-the-art result compared with other existing related methods, and has stronger domain invariance. Results also demonstrate the interpretability of WSISur.
更多查看译文
关键词
multi-center survival analysis,whole slide image,graph neural network,interpretability
AI 理解论文
溯源树
样例
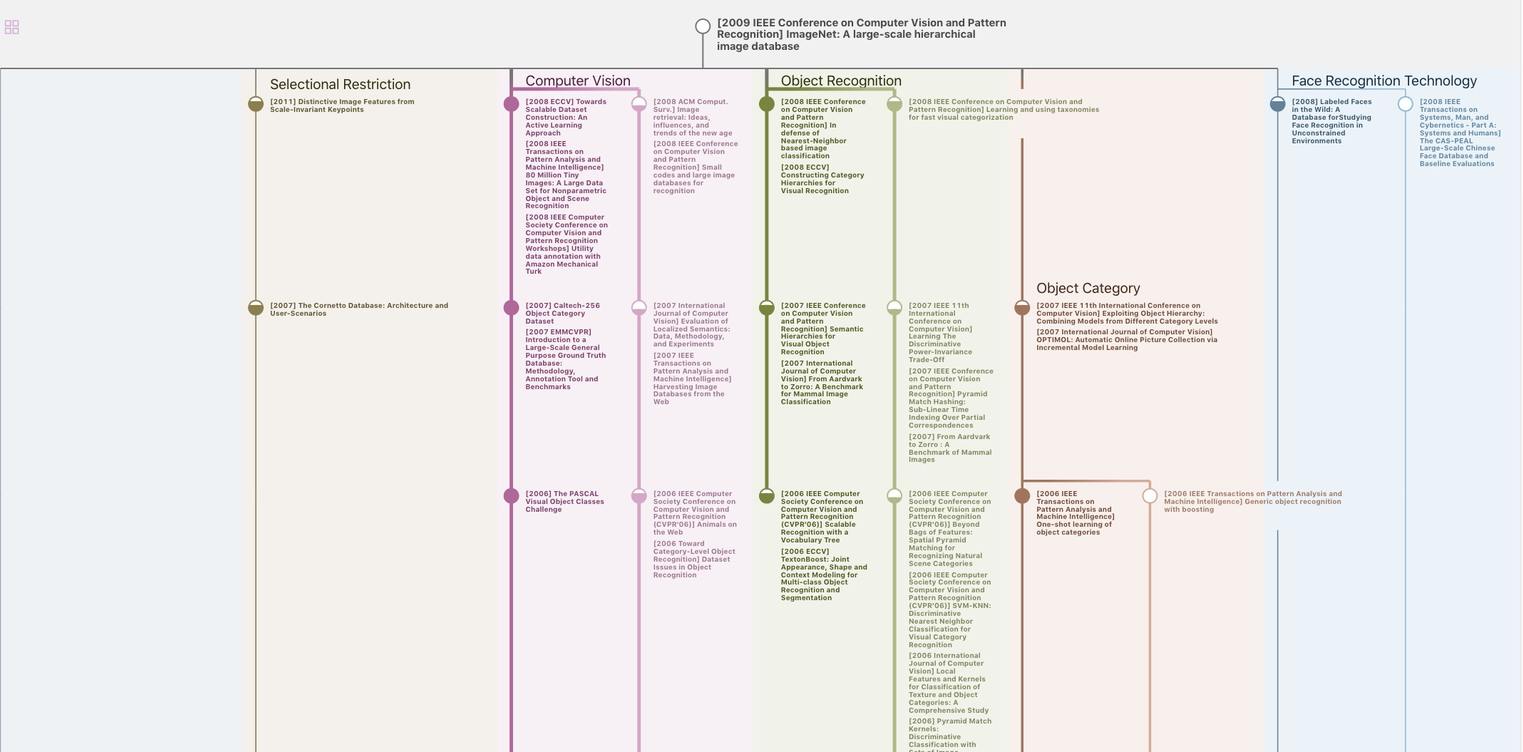
生成溯源树,研究论文发展脉络
Chat Paper
正在生成论文摘要