Pneumonia hospitalization risk attributable to ambient air pollution and temperature in a mid-temperate, industrial city: a time-series analysis
Research Square (Research Square)(2023)
Abstract
Abstract Background Pneumonia has become a public health problem of global concern, in which air pollution and meteorological conditions were important influencing factors. Methods A time series analysis was conducted on the number of pneumonia hospitalizations. The generalized additive model and distributed lag nonlinear model were used to investigate the single-day and cumulative lag-day effects of air pollutants and temperature on pneumonia hospitalizations. Results Air pollutants and meteorological factors were significantly associated with pneumonia hospitalizations. Over 7 days cumulative lag time, when the PM2.5, PM10, SO 2 , CO, and NO 2 increased by 10 µg/m 3 (0.1 mg/m 3 for CO), pneumonia hospitalizations increased by 0.56% (95% CI: 0.30, 0.81), 0.82% (95% CI: 0.62, 1.03), 4.74% (95% CI: 3.98, 5.50), 1.99% (95% CI: 1.60, 2.38), and 5.00% (95% CI: 4.24, 5.75) respectively, women and children are at higher risk. Within a cumulative lag time of 7 days, extreme cold (-18°C versus − 1°C) and extreme heat (27°C versus − 1°C) increased the risk of pneumonia hospitalizations by 8.96% (95% CI: 0.20, 18.49) and 40.78% (95% CI: 21.85, 62.65), respectively, with a higher risk in males, children, and elderly individuals. Conclusions From 2015 to 2019, pneumonia in Changchun showed an increasing trend and decreased sharply in 2020. Increased concentrations of air pollutants (PM2.5, PM10, SO 2 , CO, and NO 2 ) led to an increased risk of pneumonia hospitalizations, with a significant lag effect, among them, SO 2 and NO 2 are the strongest effect. Extreme heat and cold can lead to an increased risk of pneumonia hospitalizations.
MoreTranslated text
Key words
pneumonia,ambient air pollution,hospitalization,temperature,mid-temperate,time-series
AI Read Science
Must-Reading Tree
Example
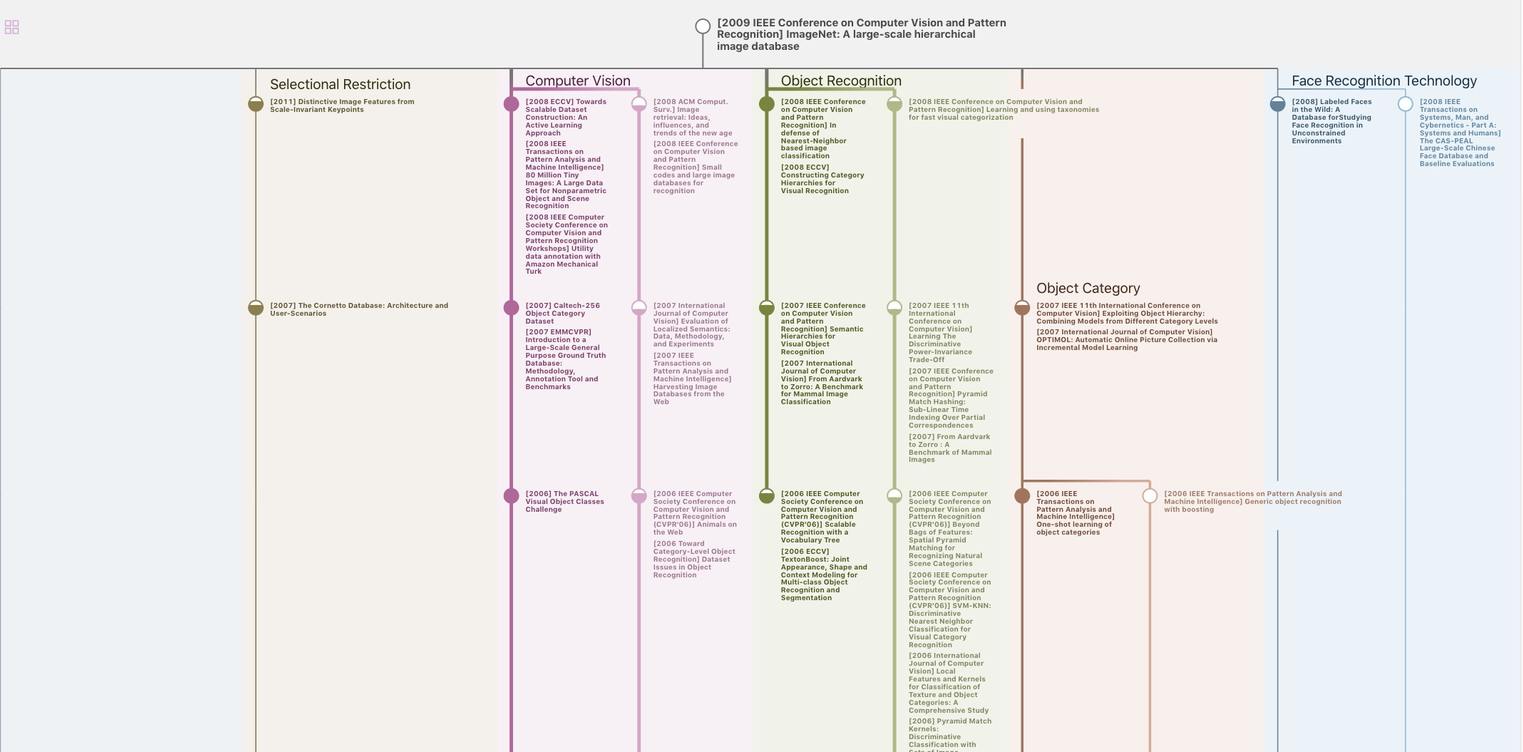
Generate MRT to find the research sequence of this paper
Chat Paper
Summary is being generated by the instructions you defined