IDEA: Invariant Causal Defense for Graph Adversarial Robustness
arXiv (Cornell University)(2023)
摘要
Graph neural networks (GNNs) have achieved remarkable success in various tasks, however, their vulnerability to adversarial attacks raises concerns for the real-world applications. Existing defense methods can resist some attacks, but suffer unbearable performance degradation under other unknown attacks. This is due to their reliance on either limited observed adversarial examples to optimize (adversarial training) or specific heuristics to alter graph or model structures (graph purification or robust aggregation). In this paper, we propose an Invariant causal DEfense method against adversarial Attacks (IDEA), providing a new perspective to address this issue. The method aims to learn causal features that possess strong predictability for labels and invariant predictability across attacks, to achieve graph adversarial robustness. Through modeling and analyzing the causal relationships in graph adversarial attacks, we design two invariance objectives to learn the causal features. Extensive experiments demonstrate that our IDEA significantly outperforms all the baselines under both poisoning and evasion attacks on five benchmark datasets, highlighting the strong and invariant predictability of IDEA. The implementation of IDEA is available at https://anonymous.4open.science/r/IDEA_repo-666B.
更多查看译文
关键词
graph adversarial robustness,invariant causal defense
AI 理解论文
溯源树
样例
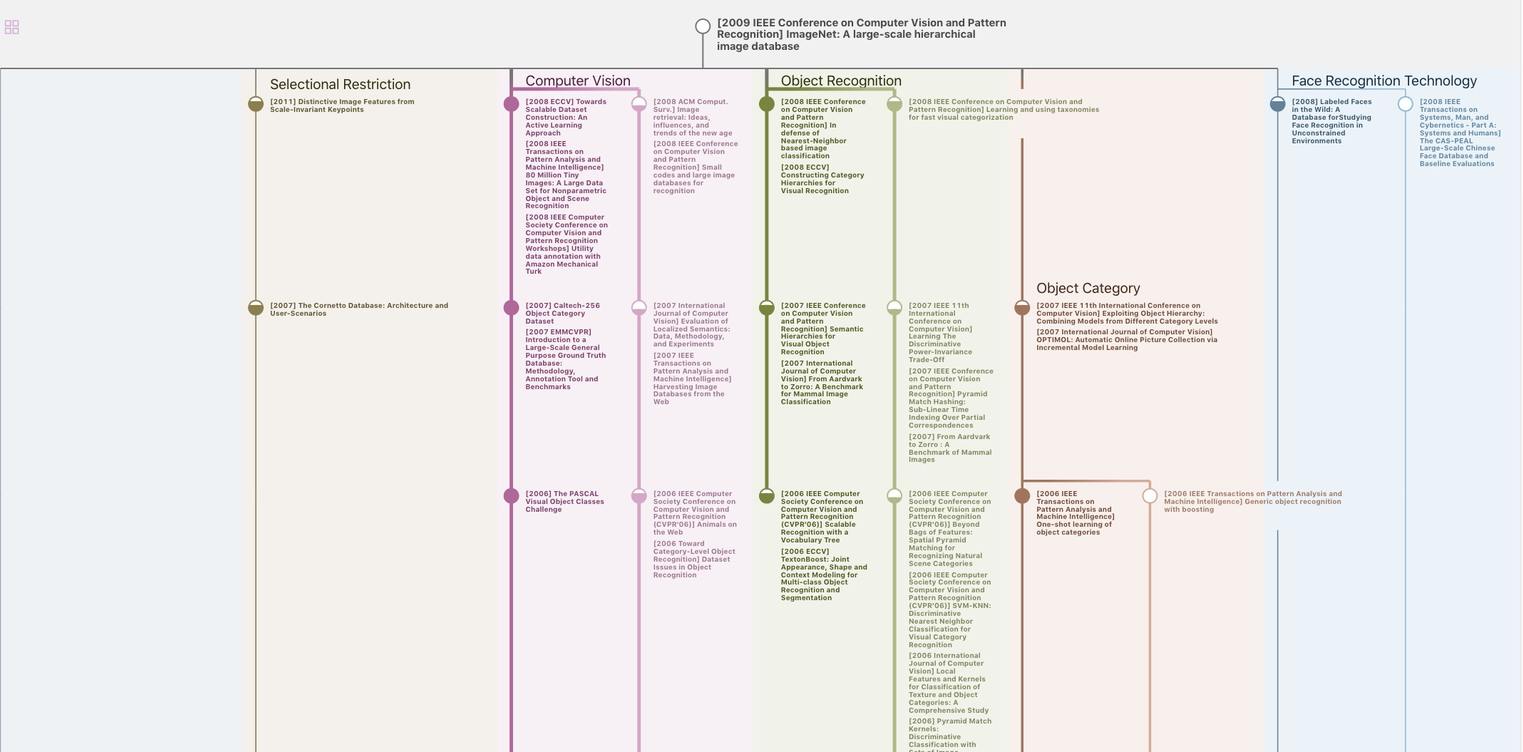
生成溯源树,研究论文发展脉络
Chat Paper
正在生成论文摘要