Learning Occupancy for Monocular 3D Object Detection
arXiv (Cornell University)(2023)
Abstract
Monocular 3D detection is a challenging task due to the lack of accurate 3D information. Existing approaches typically rely on geometry constraints and dense depth estimates to facilitate the learning, but often fail to fully exploit the benefits of three-dimensional feature extraction in frustum and 3D space. In this paper, we propose \textbf{OccupancyM3D}, a method of learning occupancy for monocular 3D detection. It directly learns occupancy in frustum and 3D space, leading to more discriminative and informative 3D features and representations. Specifically, by using synchronized raw sparse LiDAR point clouds, we define the space status and generate voxel-based occupancy labels. We formulate occupancy prediction as a simple classification problem and design associated occupancy losses. Resulting occupancy estimates are employed to enhance original frustum/3D features. As a result, experiments on KITTI and Waymo open datasets demonstrate that the proposed method achieves a new state of the art and surpasses other methods by a significant margin. Codes and pre-trained models will be available at \url{https://github.com/SPengLiang/OccupancyM3D}.
MoreTranslated text
Key words
monocular 3d object detection,object detection,occupancy
AI Read Science
Must-Reading Tree
Example
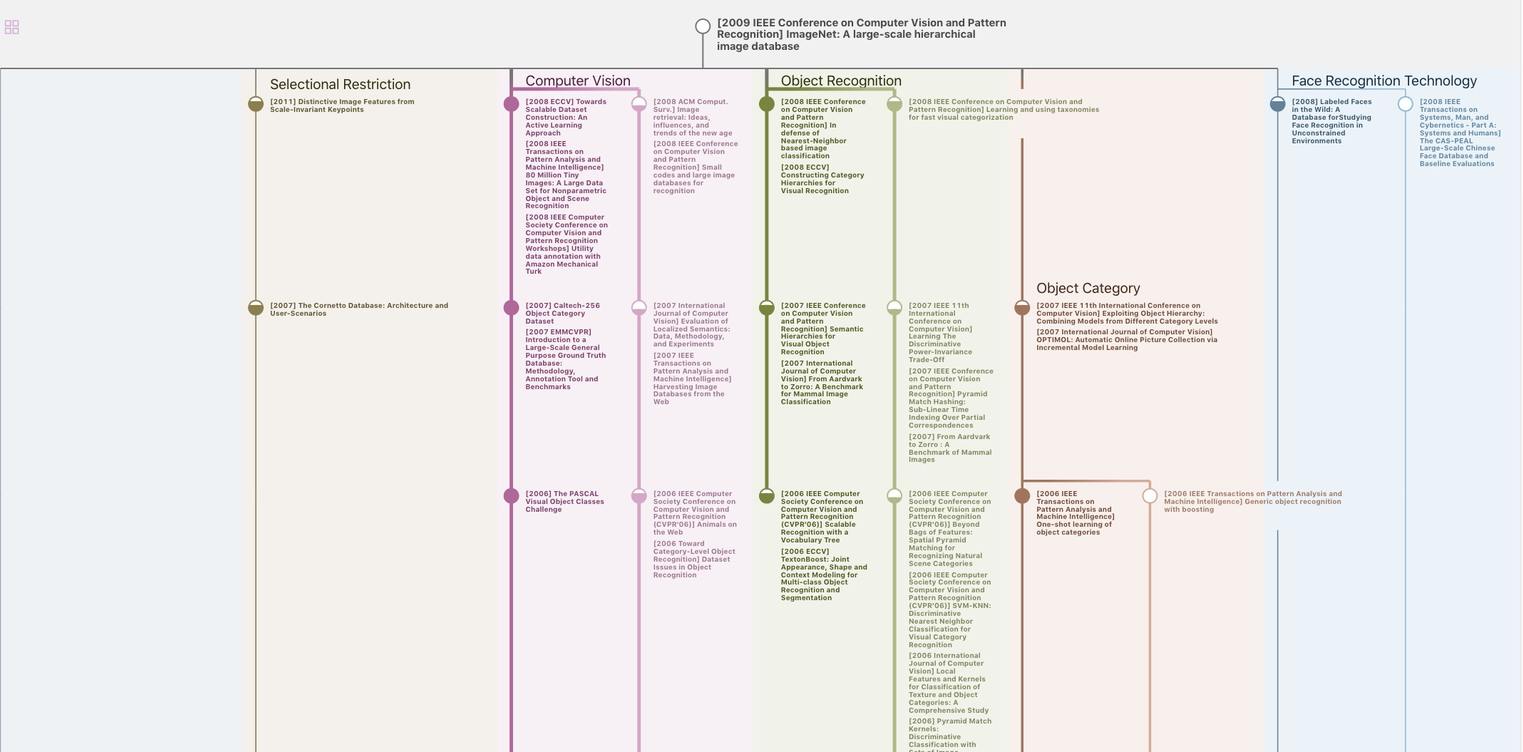
Generate MRT to find the research sequence of this paper
Chat Paper
Summary is being generated by the instructions you defined