Application of concept Drift Detection and Adaptive Framework for Non Linear Time Series Data from Cardiac Surgery
Authorea (Authorea)(2023)
摘要
The quality of machine learning (ML) models deployed in dynamic environments tends to decline over time due to disparities between the data used for training and the upcoming data available for prediction, which is commonly known as drift. Therefore, it is important for ML models to be capable of detecting any changes or drift in the data distribution and updating the ML model accordingly. This study presents various drift detection techniques to identify drift in the survival outcomes of patients who underwent cardiac surgery. Additionally, this study proposes several drift adaptation strategies, such as adaptive learning, incremental learning, and ensemble learning. Through a detailed analysis of the results, the study confirms the superior performance of ensemble model, achieving a minimum mean absolute error (MAE) of 2.65 and 3.4 for predicting hospital stay and ICU stay, respectively.
更多查看译文
关键词
concept drift detection,time series,adaptive framework,cardiac surgery
AI 理解论文
溯源树
样例
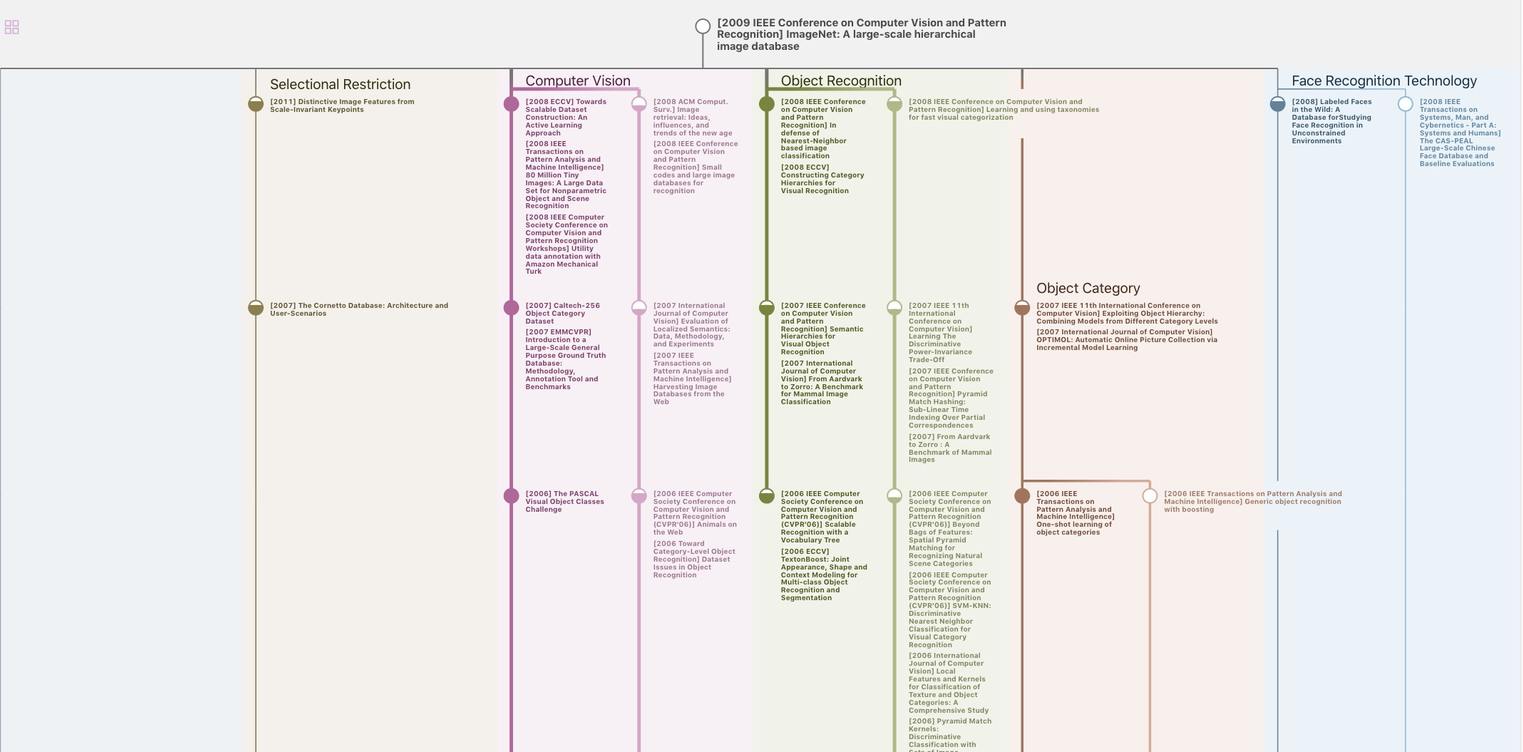
生成溯源树,研究论文发展脉络
Chat Paper
正在生成论文摘要