Abstract LB067: A confident and operator-independent deep segmentation model to measure residual tumor volume in the follow-up MRIs for glioblastoma
Cancer Research(2023)
Abstract
Abstract Introduction: Magnetic resonance imaging (MRI) is the most common tool to examine glioblastoma. Preoperative MRI can be used to initial diagnosis, and surgery planning, while follow-up MRIs can be used to evaluate treatment responses, identify recurrency, and detect side effects. The follow-up MRIs are usually taken after the first-line therapy, such as maximal safe resection. In the past, radiologists manually segment the tumor regions from normal brain tissue on follow-up MRIs, which is time-consuming, error-prone, and challenging. Several deep-learning (DL) models have been developed utilizing preoperative images, but their performance has yet to be evaluated on follow-up MRIs. In this research, we built the largest follow-up MRI cohort (311 patients) to assess these DL models and their generalizability and performance on independent preoperative and follow-up images. We also made the first follow-up-based deep learning models for this specific task. Methods: All evaluation deep learning models (10 models) were trained by the Brain Tumor Segmentation challenge 2020 (BraTS’20) and evaluated by fifty pairs of preoperative and follow-up scans from our institution. The segmentation form our institution is evaluated by board certified radiologist. MRIs in the BraTS’20 dataset were all preoperative scans. After the evaluation, we randomly assigned 264 patients’ scans from our institution to the training dataset and 47 patients’ scans to the testing dataset. We compared three types of models in our follow-up deep learning model, including 1) UNet-3D, 2) UNet-3D+transfer-learning, and 3) UNet-3D+transfer-learning+baysian-learning. The benchmark for all models was the Dice similarity coefficient (DSC). DSC can measure the spatial overlap between model prediction and ground truth. The value of DSC is between zero to one. Zero means no overlap and one indicates complete overlap. Results: Our study demonstrates that the BraTS'20 trained models' performance decreased by 13.05% in independent preoperative MRI scans and 19.04% in follow-up MRI scans. The most significant mismatch regions were FLAIR hyperintense regions (3.68% drop in independent preoperative scans and 10.61% drop in independent follow-up scans) and Non-enhancing core (5.20% drop in independent preoperative scans and 11.99% drop in independent follow-up scans). Our best model can achieve the best DSC among three tumor regions compared to all evaluation models (FLAIR hyperintense regions: DSC 0.77 V.S. DSC 0.58; Enhancing tumor region: DSC 0.87 V.S. DSC 0.68; Non-enhancing tumor region: DSC 0.92 V.S. DSC 0.55). Conclusion: Maximal safe resection induced brain structure change, decreasing the performance of the preoperative-based DL model. Implementing a follow-up MRI-based segmentation model is essential to make accurate and generalizable results to address structural changes after maximal safe resection. Our follow-up DL model demonstrates the DSC score can be recovered. We commit to further developing the tool to assist radiologists in handling follow-up MRIs for glioblastoma patients. Citation Format: Kang Lin Hsieh, Tanjida Kabir, Luis Nunez-Rubiano, Yu-Chun Hsu, Yu Cai, Juan Rodriguez Quintero, Octavio Arevalo, Kangyi Zhao, Jackie Zhang, Jiguang Zhu Zhu, Roy Riascos, Xioaqian Jiang, Shayan Shams. A confident and operator-independent deep segmentation model to measure residual tumor volume in the follow-up MRIs for glioblastoma [abstract]. In: Proceedings of the American Association for Cancer Research Annual Meeting 2023; Part 2 (Clinical Trials and Late-Breaking Research); 2023 Apr 14-19; Orlando, FL. Philadelphia (PA): AACR; Cancer Res 2023;83(8_Suppl):Abstract nr LB067.
MoreTranslated text
Key words
residual tumor volume,glioblastoma,mris,operator-independent
AI Read Science
Must-Reading Tree
Example
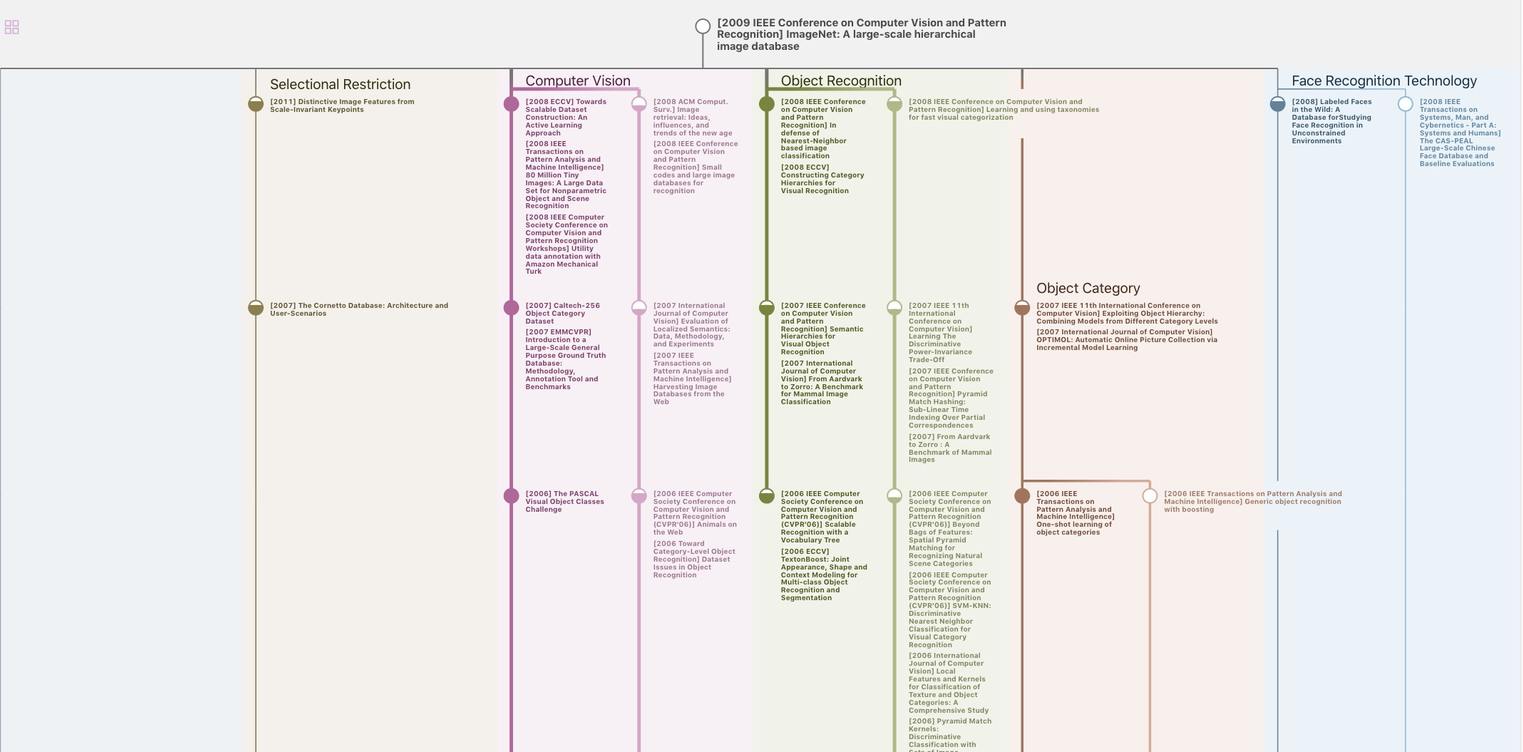
Generate MRT to find the research sequence of this paper
Chat Paper
Summary is being generated by the instructions you defined