Computationally Efficient Labeling of Cancer Related Forum Posts by Non-Clinical Text Information Retrieval
arXiv (Cornell University)(2023)
摘要
An abundance of information about cancer exists online, but categorizing and extracting useful information from it is difficult. Almost all research within healthcare data processing is concerned with formal clinical data, but there is valuable information in non-clinical data too. The present study combines methods within distributed computing, text retrieval, clustering, and classification into a coherent and computationally efficient system, that can clarify cancer patient trajectories based on non-clinical and freely available information. We produce a fully-functional prototype that can retrieve, cluster and present information about cancer trajectories from non-clinical forum posts. We evaluate three clustering algorithms (MR-DBSCAN, DBSCAN, and HDBSCAN) and compare them in terms of Adjusted Rand Index and total run time as a function of the number of posts retrieved and the neighborhood radius. Clustering results show that neighborhood radius has the most significant impact on clustering performance. For small values, the data set is split accordingly, but high values produce a large number of possible partitions and searching for the best partition is hereby time-consuming. With a proper estimated radius, MR-DBSCAN can cluster 50000 forum posts in 46.1 seconds, compared to DBSCAN (143.4) and HDBSCAN (282.3). We conduct an interview with the Danish Cancer Society and present our software prototype. The organization sees a potential in software that can democratize online information about cancer and foresee that such systems will be required in the future.
更多查看译文
关键词
cancer related forum posts,efficient labeling,text,non-clinical
AI 理解论文
溯源树
样例
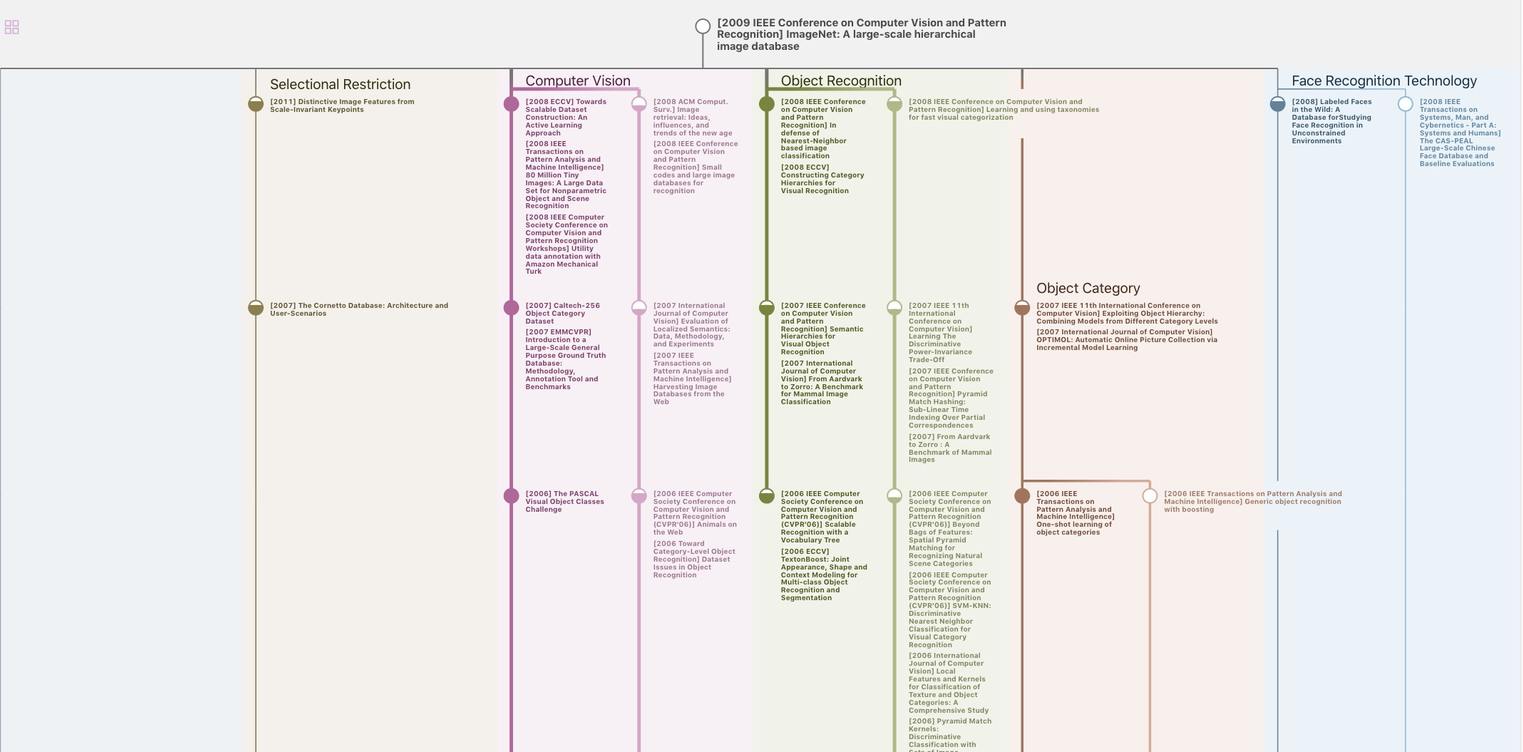
生成溯源树,研究论文发展脉络
Chat Paper
正在生成论文摘要