Better Generative Replay for Continual Federated Learning
arXiv (Cornell University)(2023)
摘要
Federated learning is a technique that enables a centralized server to learn from distributed clients via communications without accessing the client local data. However, existing federated learning works mainly focus on a single task scenario with static data. In this paper, we introduce the problem of continual federated learning, where clients incrementally learn new tasks and history data cannot be stored due to certain reasons, such as limited storage and data retention policy. Generative replay based methods are effective for continual learning without storing history data, but adapting them for this setting is challenging. By analyzing the behaviors of clients during training, we find that the unstable training process caused by distributed training on non-IID data leads to a notable performance degradation. To address this problem, we propose our FedCIL model with two simple but effective solutions: model consolidation and consistency enforcement. Our experimental results on multiple benchmark datasets demonstrate that our method significantly outperforms baselines.
更多查看译文
关键词
better generative replay,learning
AI 理解论文
溯源树
样例
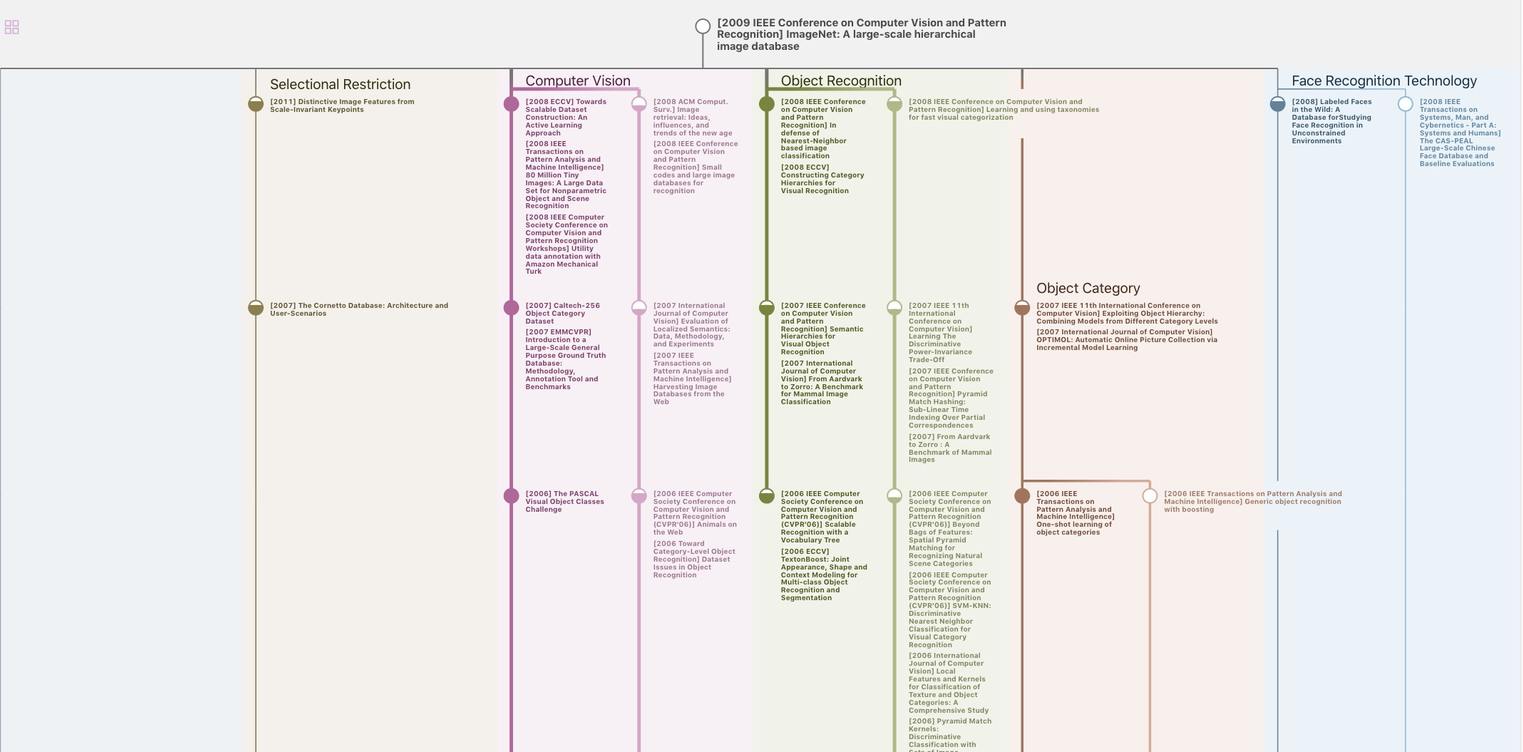
生成溯源树,研究论文发展脉络
Chat Paper
正在生成论文摘要