Reinforcement Learning and Distributed Model Predictive Control for Conflict Resolution in Highly Constrained Spaces
arXiv (Cornell University)(2023)
摘要
This work presents a distributed algorithm for resolving cooperative multi-vehicle conflicts in highly constrained spaces. By formulating the conflict resolution problem as a Multi-Agent Reinforcement Learning (RL) problem, we can train a policy offline to drive the vehicles towards their destinations safely and efficiently in a simplified discrete environment. During the online execution, each vehicle first simulates the interaction among vehicles with the trained policy to obtain its strategy, which is used to guide the computation of a reference trajectory. A distributed Model Predictive Controller (MPC) is then proposed to track the reference while avoiding collisions. The preliminary results show that the combination of RL and distributed MPC has the potential to guide vehicles to resolve conflicts safely and smoothly while being less computationally demanding than the centralized approach.
更多查看译文
关键词
distributed model predictive control,conflict resolution,constrained spaces
AI 理解论文
溯源树
样例
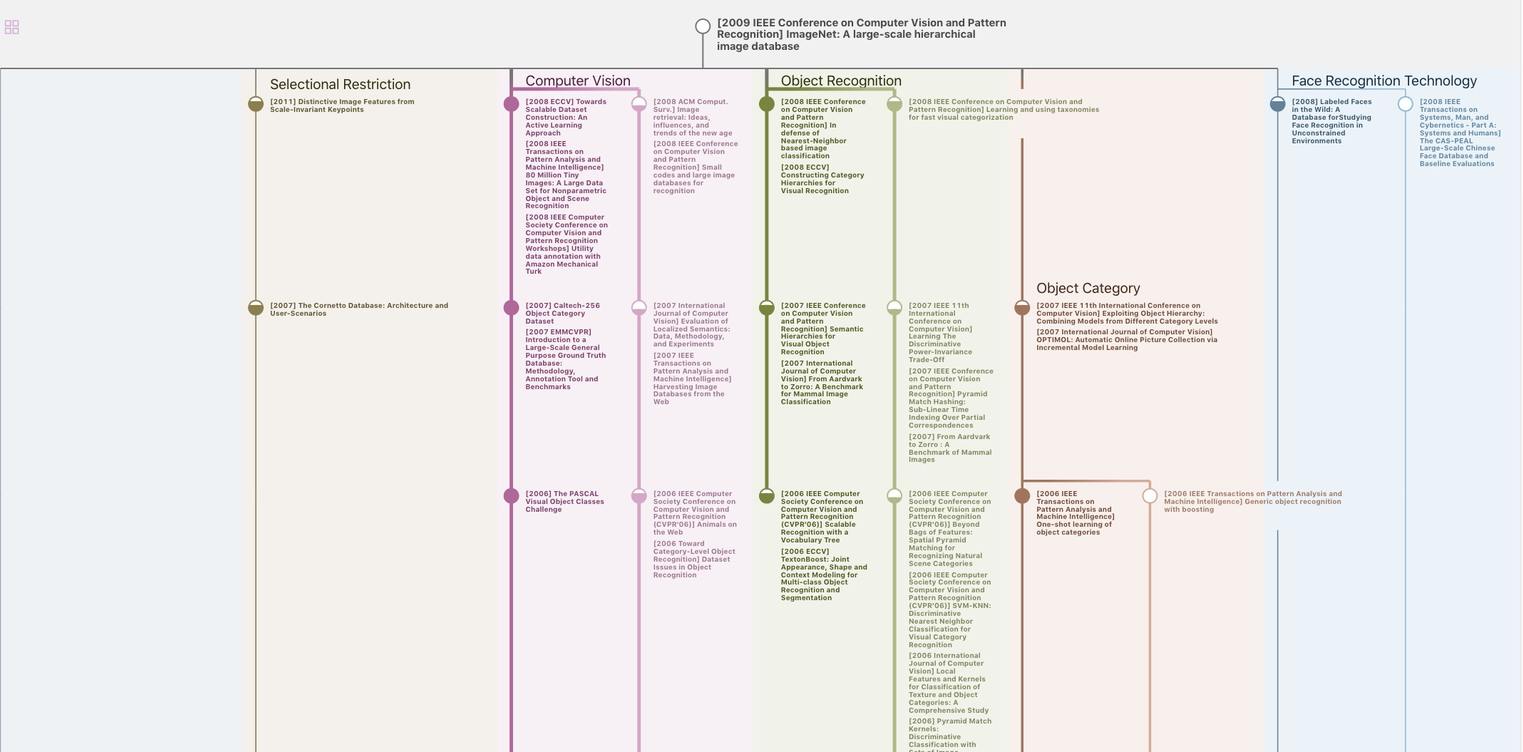
生成溯源树,研究论文发展脉络
Chat Paper
正在生成论文摘要