Deep-Learning-Based LSTM Model for Predicting a Tidal River’s Water Levels: A Case Study of the Kapuas Kecil River, Indonesia
Communications in computer and information science(2023)
摘要
Accurate prediction of water levels in tidal rivers is crucial for effective disaster management in coastal areas. This study uses the LSTM deep learning method to forecast the water level dynamics of the Kapuas Kecil River and determine the optimal window size for precise predictions. Our results reveal an optimal window size of 336 h (equivalent to 14 days) for water level prediction using LSTM in this coastal region. Using this optimal window size, the LSTM model consistently outperforms GRU and RNN models in comparative assessments. These findings offer not only valuable insights into water level prediction in the study area but also the potential of deep learning to enhance flood and disaster management in similar river systems globally.
更多查看译文
AI 理解论文
溯源树
样例
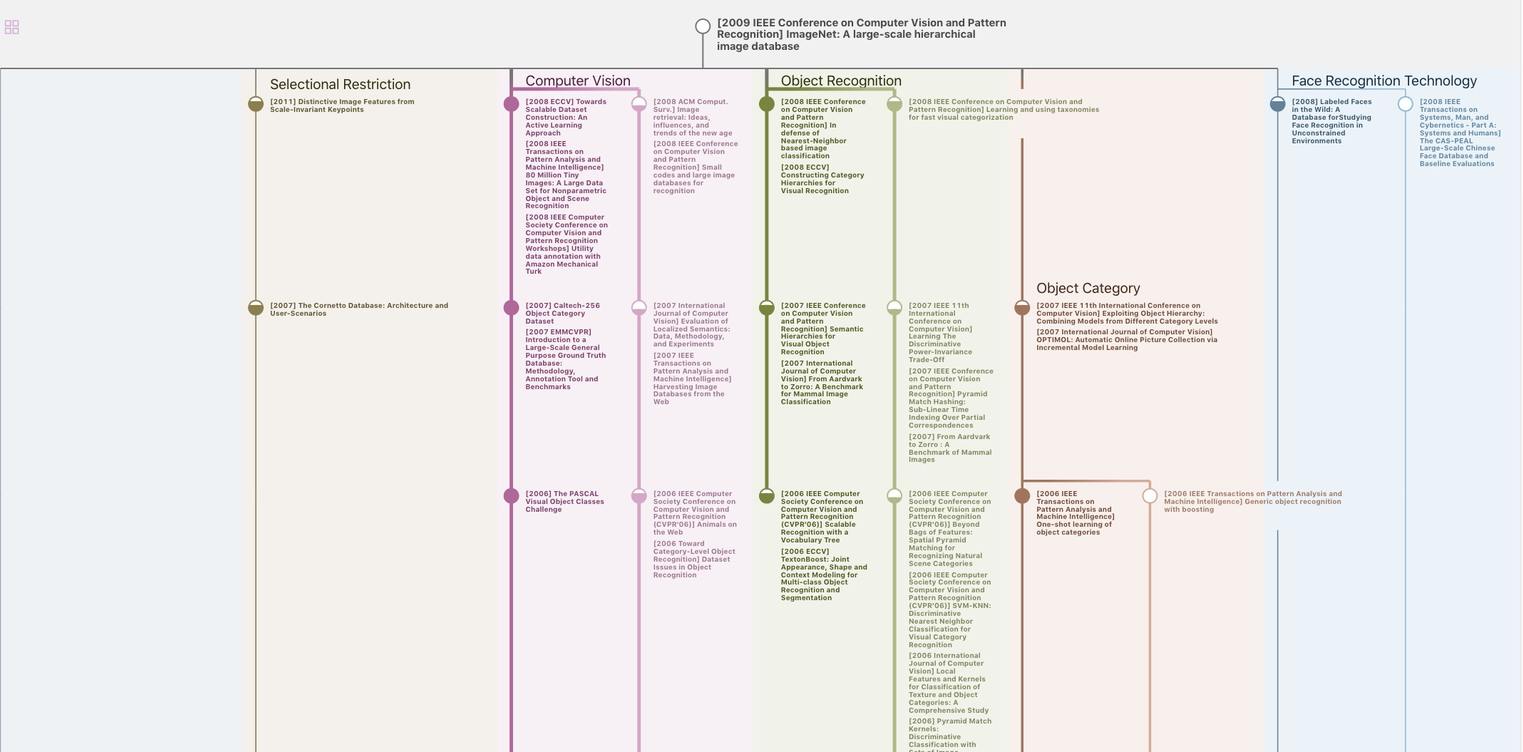
生成溯源树,研究论文发展脉络
Chat Paper
正在生成论文摘要