Time-segment Photovoltaic Forecasting and Uncertainty Analysis Based on Multi-objective Slime Mould Algorithm to Improve Support Vector Machine
IEEE Transactions on Power Systems(2023)
摘要
A photovoltaic power prediction and uncertainty analysis method, which is based on time-sharing, multi-objective slime mould optimization algorithm (MOSMA), support vector machine (SVM) and nonparametric kernel density estimation, has been proposed in this study. Furthermore, the high-resolution solar irradiance forecast value of a certain area is obtained through WRF model. The weather forecast value of local temperature and wind speed is crawled, which is matched to the same type of historical data in the corresponding period for processing. A new algorithm that MOSMA-SVM is also put forward to improve the power prediction accuracy. In addition, the probability density of the power prediction error is calculated using the nonparametric kernel density estimation method, and the confidence interval is established according to the probability density distribution. On this basis, the uncertainty of the photovoltaic power prediction is analyzed, and the prediction model is applied to the Yulala photovoltaic power plant in central Australia. In comparison to the particle swarm optimization support vector machine, whale algorithm optimization support vector machine, and three deep learning algorithms, the average absolute percentage error (MAPE) of the proposed algorithm is reduced by [0.25% - 27.13%], indicating that the proposed algorithm has higher accuracy.
更多查看译文
关键词
multi-objective slime mould optimization algorithm,support vector machine,power forecasting,WRF-SOLAR,KDE
AI 理解论文
溯源树
样例
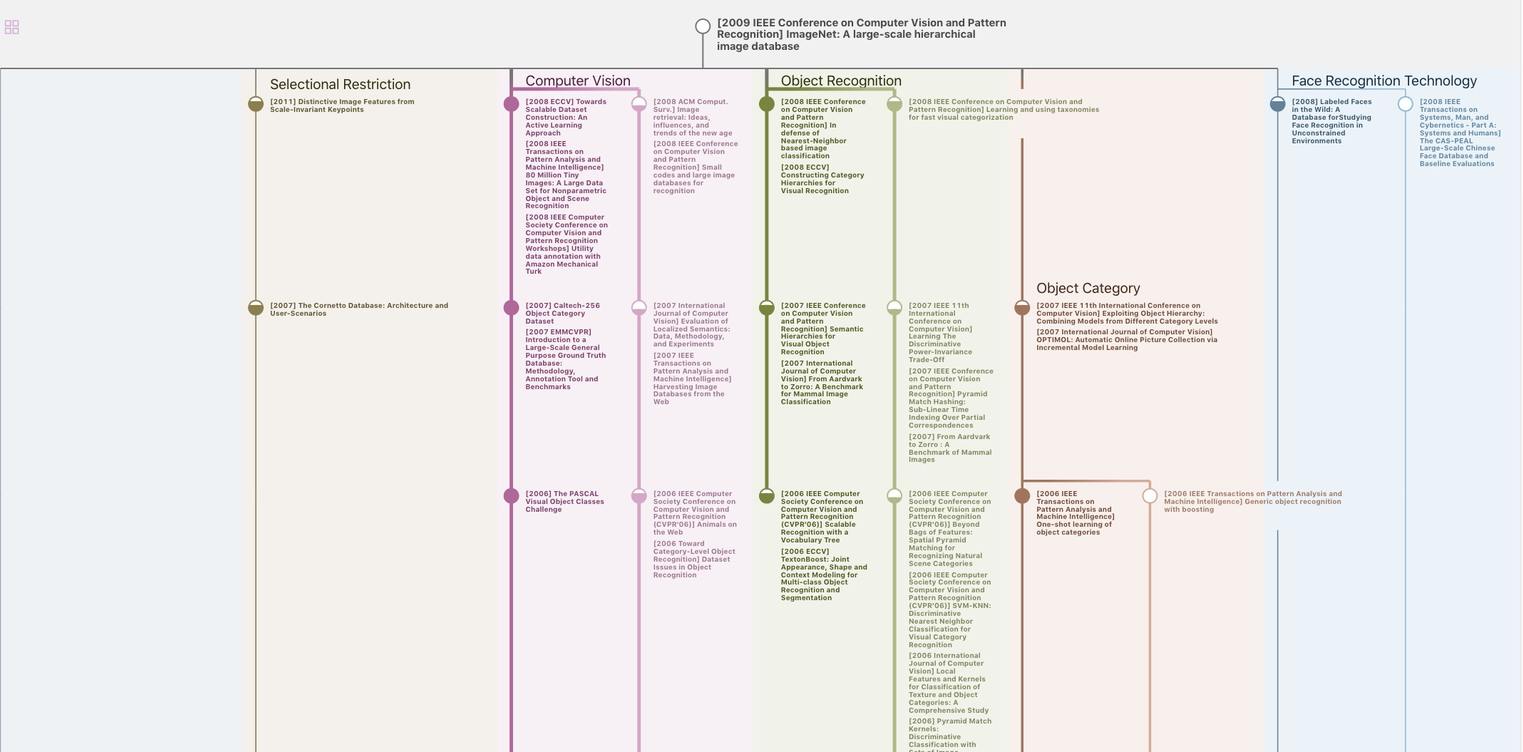
生成溯源树,研究论文发展脉络
Chat Paper
正在生成论文摘要