An Unsupervised Fault Detection and Diagnosis With Distribution Dissimilarity and Lasso Penalty
IEEE TRANSACTIONS ON CONTROL SYSTEMS TECHNOLOGY(2023)
摘要
Unsupervised fault detection and diagnosis methods generally have the following shortcomings in their projection vectors: 1) they may not be specially designed to differentiate between normal and abnormal samples; 2) they may remain unchanged for different abnormal conditions; and 3) the key variables for the process anomalies may have not been effectively selected. In this study, a fault detection and diagnosis scheme sparse distribution dissimilarity analytics (SDDA) is proposed with a lasso penalty and distribution dissimilarity to solve these issues. The proposed method is formulated through a nonconvex optimization problem with a lasso penalty, which aims to maximize the distribution dissimilarity between different data sets. Then, the nonconvex optimization problem is recast to an iterative convex optimization problem using the minorization-maximization algorithm. After that, the constraint conditions are removed using the Karush-Kuhn-Tucker conditions for further simplification. Finally, the unconstraint optimization problem is solved through the proposed feasible gradient direction method. Based on the obtained sparse projection vectors, a fault detection model with both static deviation and dynamic fluctuation is developed. Since the statistics are designed using distribution dissimilarity, some abnormal conditions with small fault magnitudes can also be accurately detected. Besides, a reconstruction-based contribution (RBC) method is proposed for the statistics, and its diagnosability has been strictly demonstrated in theory. The detection and diagnosis performance of the proposed SDDA method is validated using a simulated process and a real industrial process. Experimental results illustrate the superiority of the proposed method to some commonly used methods.
更多查看译文
关键词
Diagnosability analysis,distribution dissimilarity,fault detection and diagnosis,lasso penalty,nonconvex optimization
AI 理解论文
溯源树
样例
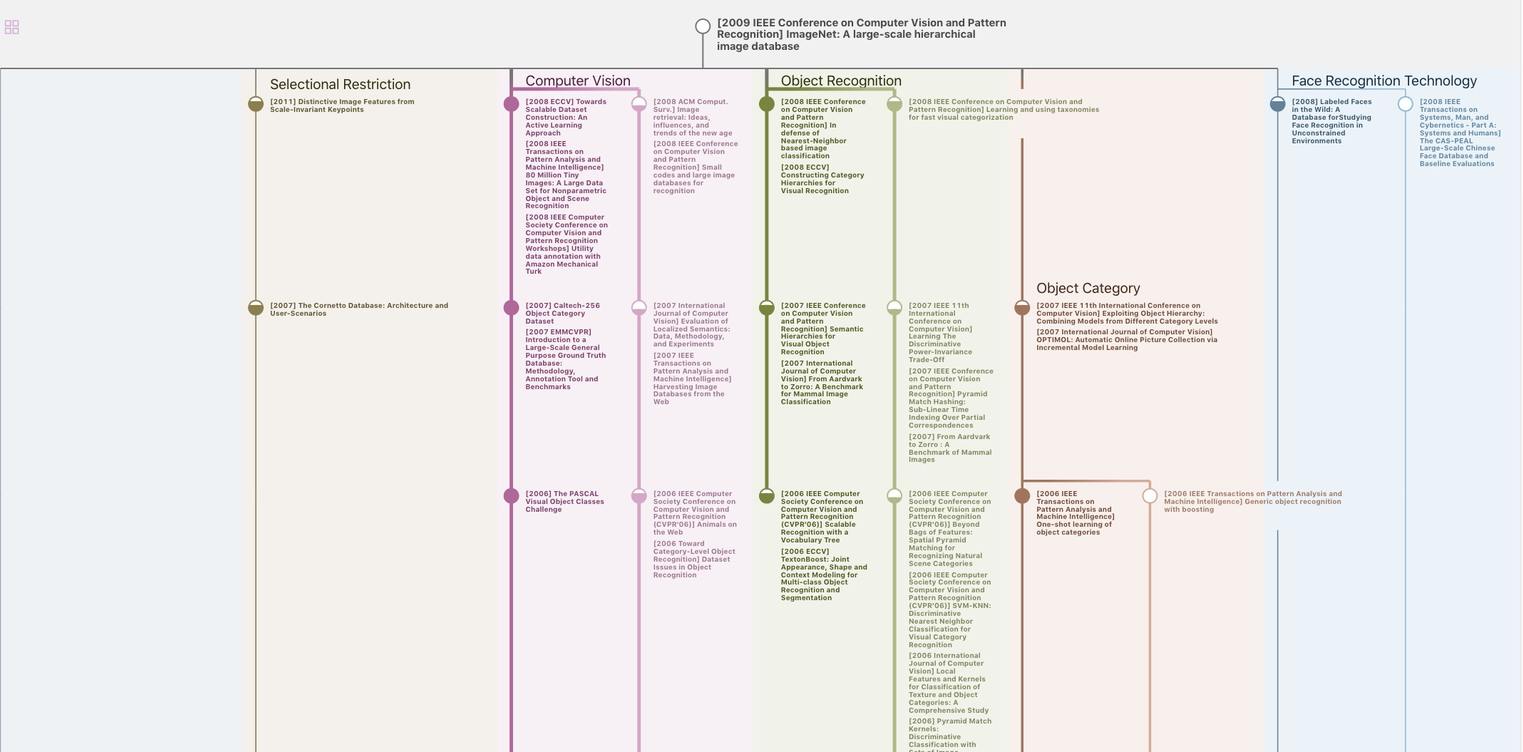
生成溯源树,研究论文发展脉络
Chat Paper
正在生成论文摘要