Training Supervised Neural Networks for PolSAR Despeckling With an Hybrid Approach
IEEE GEOSCIENCE AND REMOTE SENSING LETTERS(2024)
摘要
Synthetic aperture radar (SAR) are fundamental system for Earth Observation. In particular, polarimetric SAR (PolSAR) sensors provide images of a scene at different polarizations, enriching the information that can be retrieved. Due to their coherent nature, SAR images are complex data affected by a multiplicative noise, called speckle. The presence of this noise hinders the interpretation of images, making speckle removal a fundamental preprocessing step for further applications. Several deep learning (DL)-based approaches have been recently proposed for speckle removal in PolSAR data relying, due to the lack of a real ground truth, on different strategies for constructing training datasets making a real comparison complicated. In this work, a study on the construction of a training dataset for PolSAR despeckling is proposed. In particular, considering the analysis recently conducted on the construction of the dataset for training supervised neural networks for SAR amplitude despeckling, the aim is to extend such studies to the PolSAR case. In particular, the commonly used multitemporal approach, relying on the stack of real data, is compared with the so-called hybrid approach, in which a mixture of real and synthetic data is proposed. A specific DL solution has been chosen for such comparison, but the analysis could be extended to whatever supervised neural network. Moreover, for the sake of completeness, results are also compared with a well-assessed PolSAR despeckling filter in the literature.
更多查看译文
关键词
Covariance matrices,Training,Speckle,Noise measurement,Neural networks,Earth,Deep learning,CNN,deep learning (DL),despeckling,image restoration,statistical distribution,synthetic aperture radar (SAR)
AI 理解论文
溯源树
样例
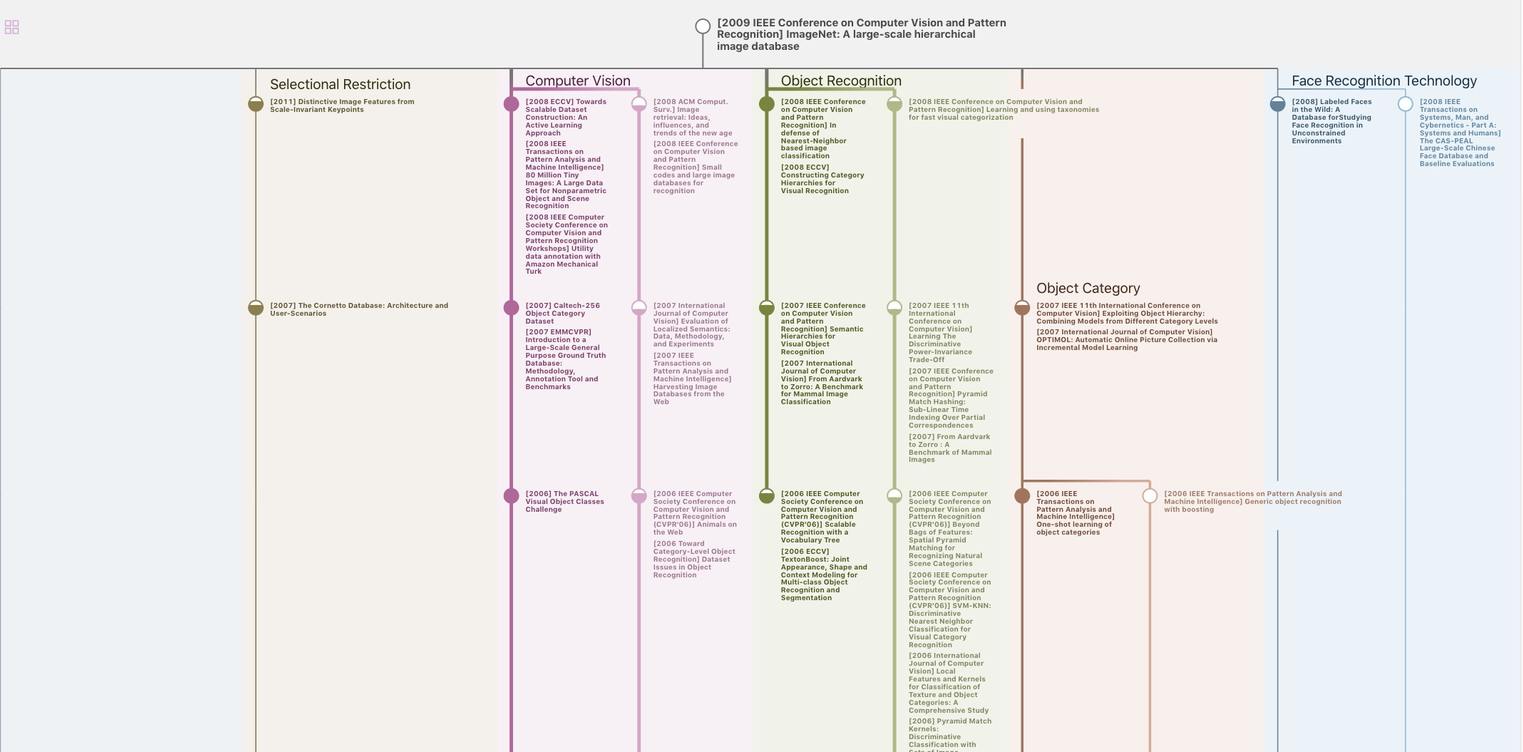
生成溯源树,研究论文发展脉络
Chat Paper
正在生成论文摘要