Deep-Learning-Aided Intraframe Motion Correction for Low-Count Dynamic Brain PET
IEEE TRANSACTIONS ON RADIATION AND PLASMA MEDICAL SCIENCES(2024)
摘要
Data-driven intraframe motion correction of a dynamic brain PET scan (with each frame duration on the order of minutes) is often achieved through the co-registration of high-temporal-resolution (e.g., 1-s duration) subframes to estimate subject head motion. However, this conventional method of subframe co-registration may perform poorly during periods of low counts and/or drastic changes in the spatial tracer distribution over time. Here, we propose a deep learning (DL), U-Net-based convolutional neural network model which aids in the PET motion estimation to overcome these limitations. Unlike DL models for PET denoising, a nonstandard 2.5-D DL model was used which transforms the high-temporal-resolution subframes into nonquantitative DL subframes which allow for improved differentiation between noise and structural/functional landmarks and estimate a constant tracer distribution across time. When estimating motion during periods of drastic change in spatial distribution (within the first minute of the scan, similar to 1-s temporal resolution), the proposed DL method was found to reduce the expected magnitude of error (+/-) in the estimation for an artificially injected motion trace from 16 mm and 7 degrees (conventional method) to 0.7 mm and 0.6 degrees (DL method). During periods of low counts but a relatively constant spatial tracer distribution (60th min of the scan, similar to 1-s temporal resolution), an expected error was reduced from 0.5 mm and 0.7 degrees (conventional method) to 0.3 mm and 0.4 degrees (DL method). The use of the DL method was found to significantly improve the accuracy of an image-derived input function calculation when motion was present during the first minute of the scan.
更多查看译文
关键词
Deep learning (DL),motion compensation,positron emission tomography
AI 理解论文
溯源树
样例
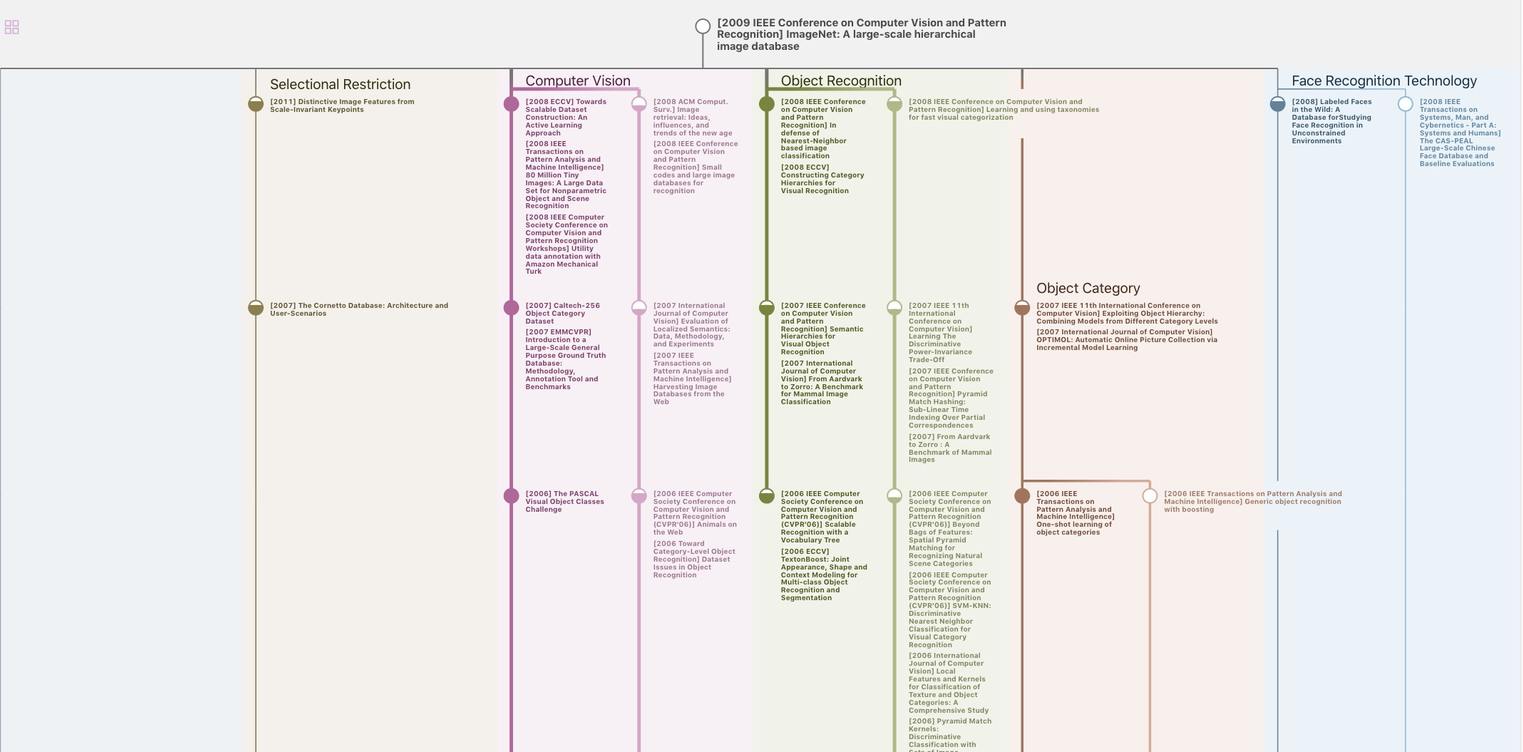
生成溯源树,研究论文发展脉络
Chat Paper
正在生成论文摘要